An efficient surrogate-assisted Taguchi salp swarm algorithm and its application for intrusion detection
Wireless Networks(2024)
摘要
The meta-heuristic algorithms require a lot of fitness calculations to get good enough solutions, which constitutes an obstacle to solving computationally complex practical problems. Recently, it has been found that surrogate-assisted meta-heuristic algorithms show potential in solving expensive complex optimization problems. This paper proposes an efficient surrogate-assisted Taguchi salp swarm algorithm (SATSSA) to solve expensive complex optimization problems. The SATSSA uses a combination of the local surrogate-assisted model (LSAM), global surrogate-assisted model (GSAM), and k-means clustering surrogate-assisted model (KCSAM) to fit the fitness function. To enhance the prediction ability of the model, an improved salp swarm algorithm with the Taguchi method (TSSA) is proposed to update and predict the model. GSAM is mainly used to capture the entire landscape of the search space. KCSAM is designed to capture part of the search space to improve the exploration capability of the algorithm. LSAM is used to capture the contours around the optimal individual. The proposed SATSSA is compared with other four excellent algorithms on 30D, 50D, and 100D benchmark functions. In addition, SATSSA is also applied to intrusion detection. Simulation results show that SATSSA is effective in improving detection rate and reducing false alarm rate.
更多查看译文
关键词
Surrogate-assisted,Salp swarm algorithm,Taguchi method,Expensive optimization problems,Intrusion detection
AI 理解论文
溯源树
样例
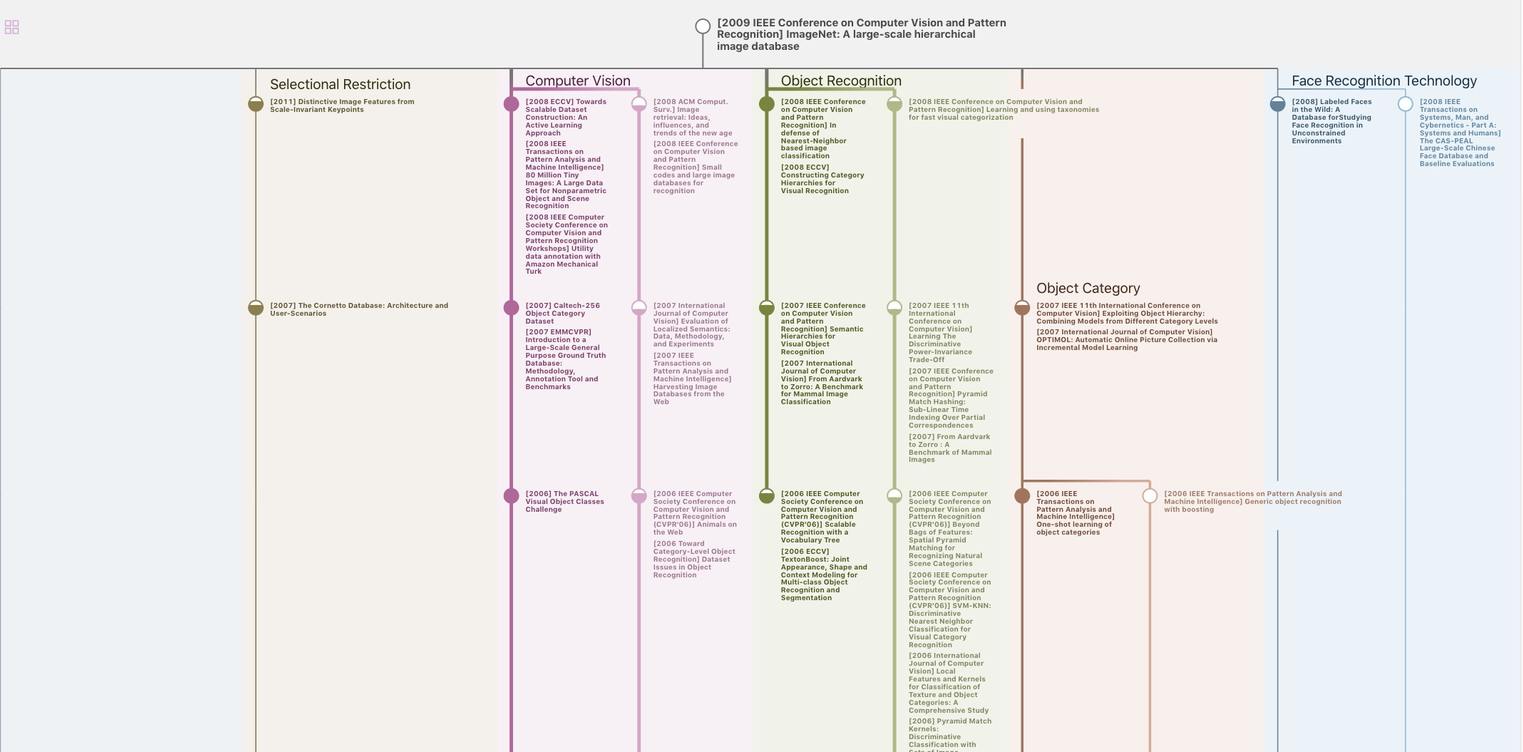
生成溯源树,研究论文发展脉络
Chat Paper
正在生成论文摘要