Bridging Information Gaps in Menopause Status Classification Through Natural Language Processing.
JAMIA OPEN(2024)
关键词
natural language processing,menopause,phenotyping,women's health
AI 理解论文
溯源树
样例
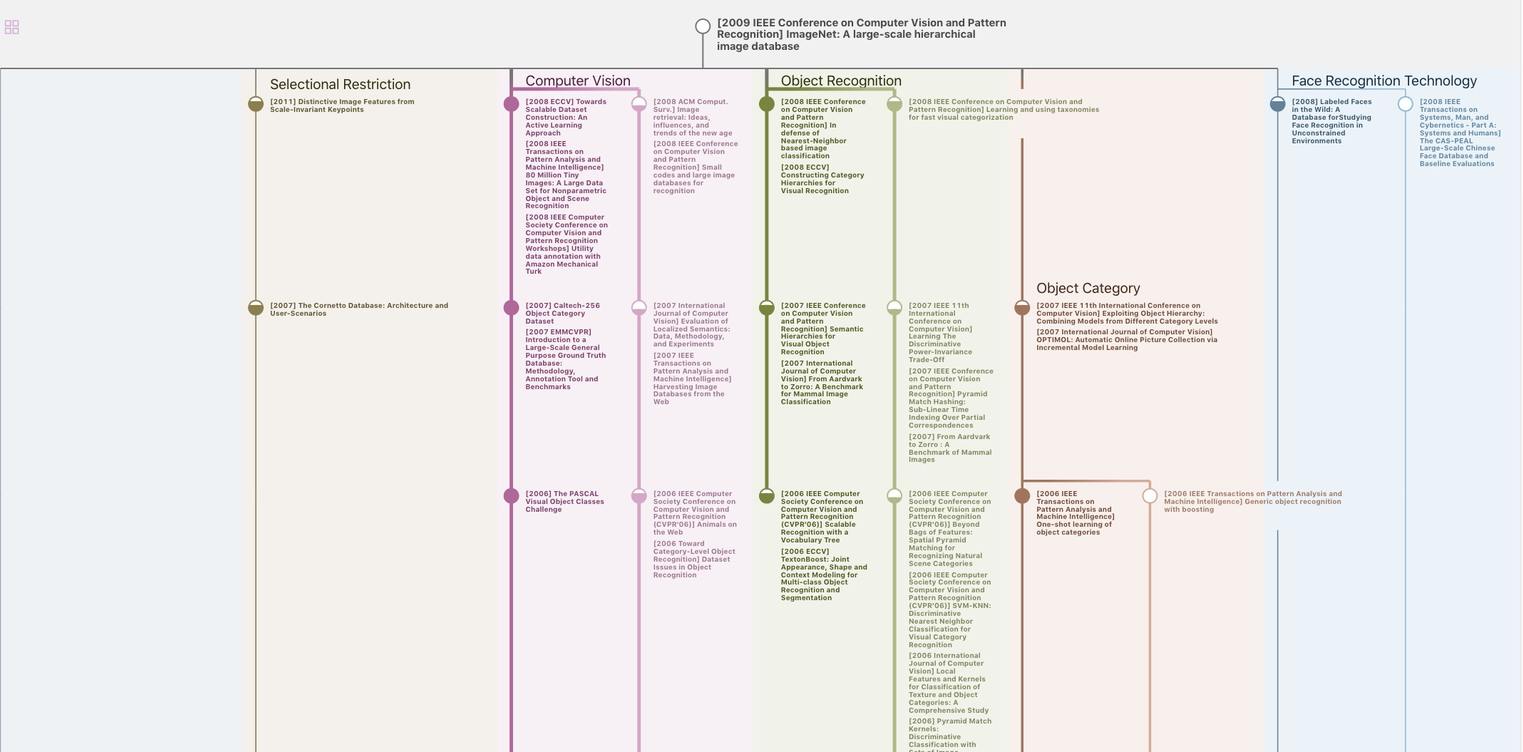
生成溯源树,研究论文发展脉络
Chat Paper
正在生成论文摘要
JAMIA OPEN(2024)