Convolutional Attention Network for Electrical/Ultrasound Dual-Modal Fusion 2-D Image Reconstruction
IEEE Transactions on Instrumentation and Measurement(2024)
摘要
It is urgent to monitor the dynamic industrial process in real-time, fast and accurately, and provide useful information for visualization. Various modalities include abundant information such as conflict, redundancy, and complementarity. It is reasonable to combine different information from multiple modalities to make a more accurate assessment for image reconstruction. In this article, a convolutional attention fusion network for electrical/ultrasound dual-modal fusion image reconstruction is proposed. Two fully connected modules are used to transform the measurement spatial information of different sensitive principles into image spatial information of the same dimension, which solves the heterogeneity problem of different modal information; in the process of feature extraction, the ill-posedness of image reconstruction is improved by adding feature dimension; the spatial pyramid pooling module is used to obtain multiscale feature information and provide more effective features for image reconstruction; according to the correlation between different modal features, the importance of different modal features to the reconstruction target is judged by attention mechanism (AM), and different modal local features with different attention weights, which promotes effective fusion and collaborative imaging of dual-modal information. The experimental results show that the convolution attention fusion reconstruction network improves the reconstruction accuracy and anti-noise performance compared with the dual-modal concatenation fusion reconstruction method and the single-modal reconstruction method.
更多查看译文
关键词
Attention,convolution network,dual-modal,electrical resistance tomography (ERT),image reconstruction,ultrasound transmission tomography
AI 理解论文
溯源树
样例
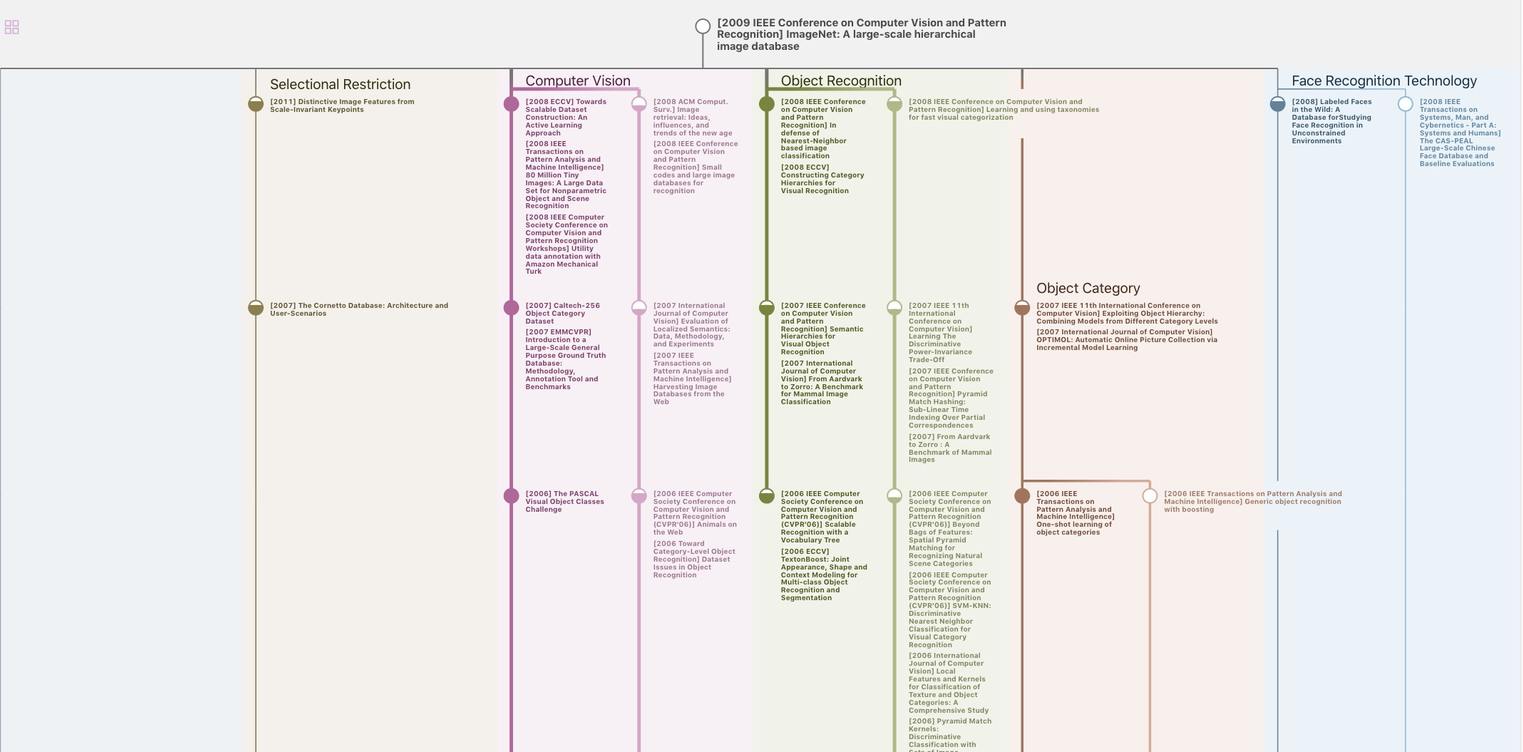
生成溯源树,研究论文发展脉络
Chat Paper
正在生成论文摘要