Misbehaviour Detection for Smart Grids using a Privacy-centric and Computationally Efficient Federated Learning Approach.
Global Communications Conference(2023)
摘要
Federated Learning (FL)-based Intrusion Detection Systems (IDSs) have recently surfaced as viable privacy-preserving solution to decentralized grid zones. However, conventional synchronous FL methods face technical challenges including the lack of consideration of communication delays and straggler nodes. To level the playing field, we propose a novel power system misbehaviour detection framework that leverages semi-asynchronous federated learning and dynamic aggregation. Specifically, our framework introduces an adaptive learning rate mechanism in the semi-asynchronous FL setting, allowing for efficient model updates and mitigating the impact of stragglers on the training process. Experiments conducted on publicly available Mississippi State University and Oak Ridge National Laboratory Power System Attack (MSU-ORNL PSA) Dataset demonstrate that our adaptive learning semi-asynchronous FL framework achieves superior attack detection rate while safeguarding data confidentiality and minimizing the negative effects of practical world communication latency and straggler nodes. Furthermore, our proposed method shows a significant 40% improvement in training time compared to conventional synchronous FL methods, showcasing the effectiveness and efficiency of our recommended approach.
更多查看译文
关键词
Federated Learning,Intrusion Detection,Internet of Things (IoT),Smart Grid
AI 理解论文
溯源树
样例
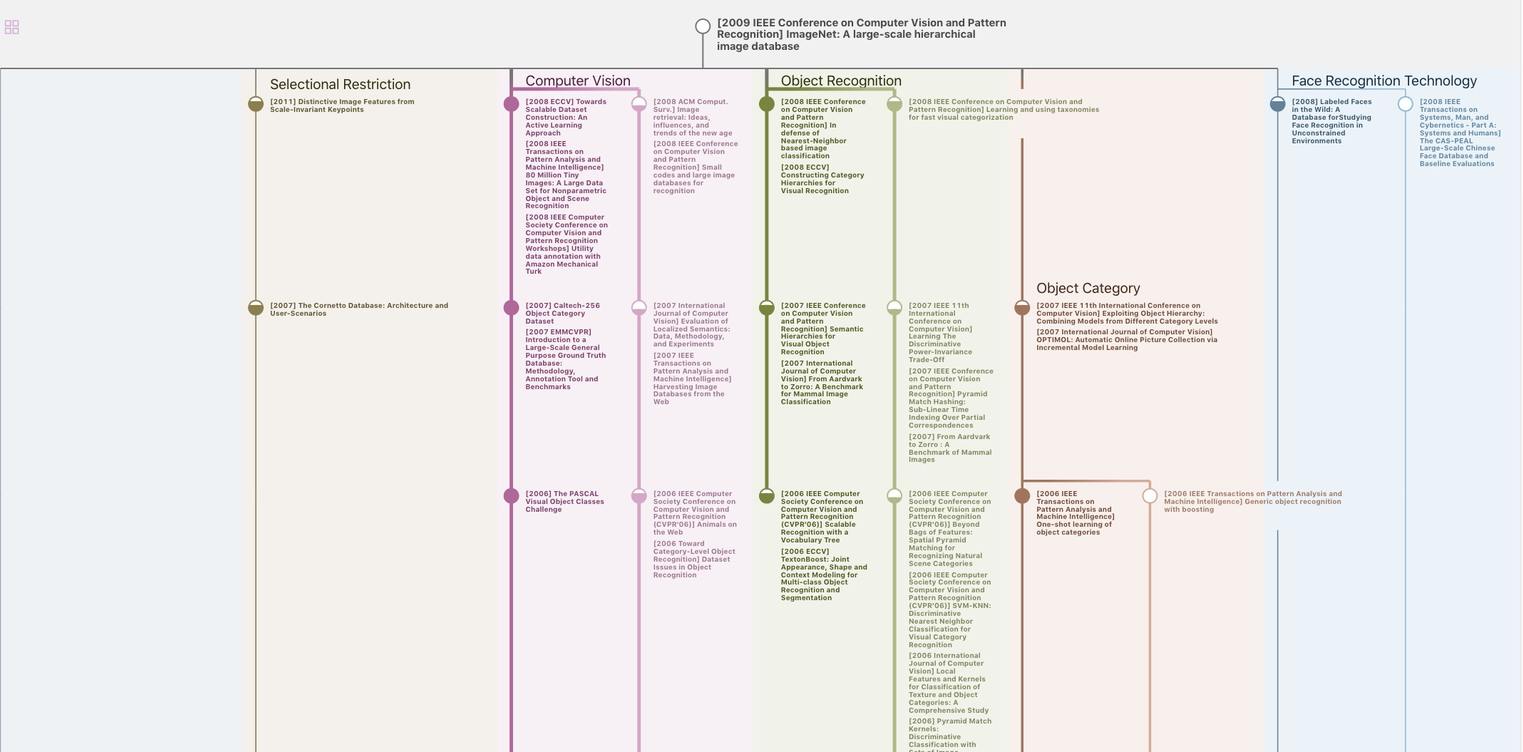
生成溯源树,研究论文发展脉络
Chat Paper
正在生成论文摘要