LLMBind: A Unified Modality-Task Integration Framework
CoRR(2024)
Abstract
In the multi-modal domain, the dependence of various models on specific inputformats leads to user confusion and hinders progress. To address thischallenge, we introduce LLMBind, a novel framework designed to unify adiverse array of multi-modal tasks. By harnessing a Mixture-of-Experts (MoE)Large Language Model (LLM), LLMBind processes multi-modal inputs and generatestask-specific tokens, enabling the invocation of corresponding models toaccomplish tasks. This unique approach empowers LLMBind to interpret inputs andgenerate outputs across various modalities, including image, text, video, andaudio. Furthermore, we have constructed an interaction dataset comprising 400kinstructions, which unlocks the ability of LLMBind for interactive visualgeneration and editing tasks. Extensive experimentation demonstrates thatLLMBind achieves very superior performance across diverse tasks and outperformsexisting models in user evaluations conducted in real-world scenarios.Moreover, the adaptability of LLMBind allows for seamless integration with thelatest models and extension to new modality tasks, highlighting its potentialto serve as a unified AI agent for modeling universal modalities.
MoreTranslated text
PDF
View via Publisher
AI Read Science
Must-Reading Tree
Example
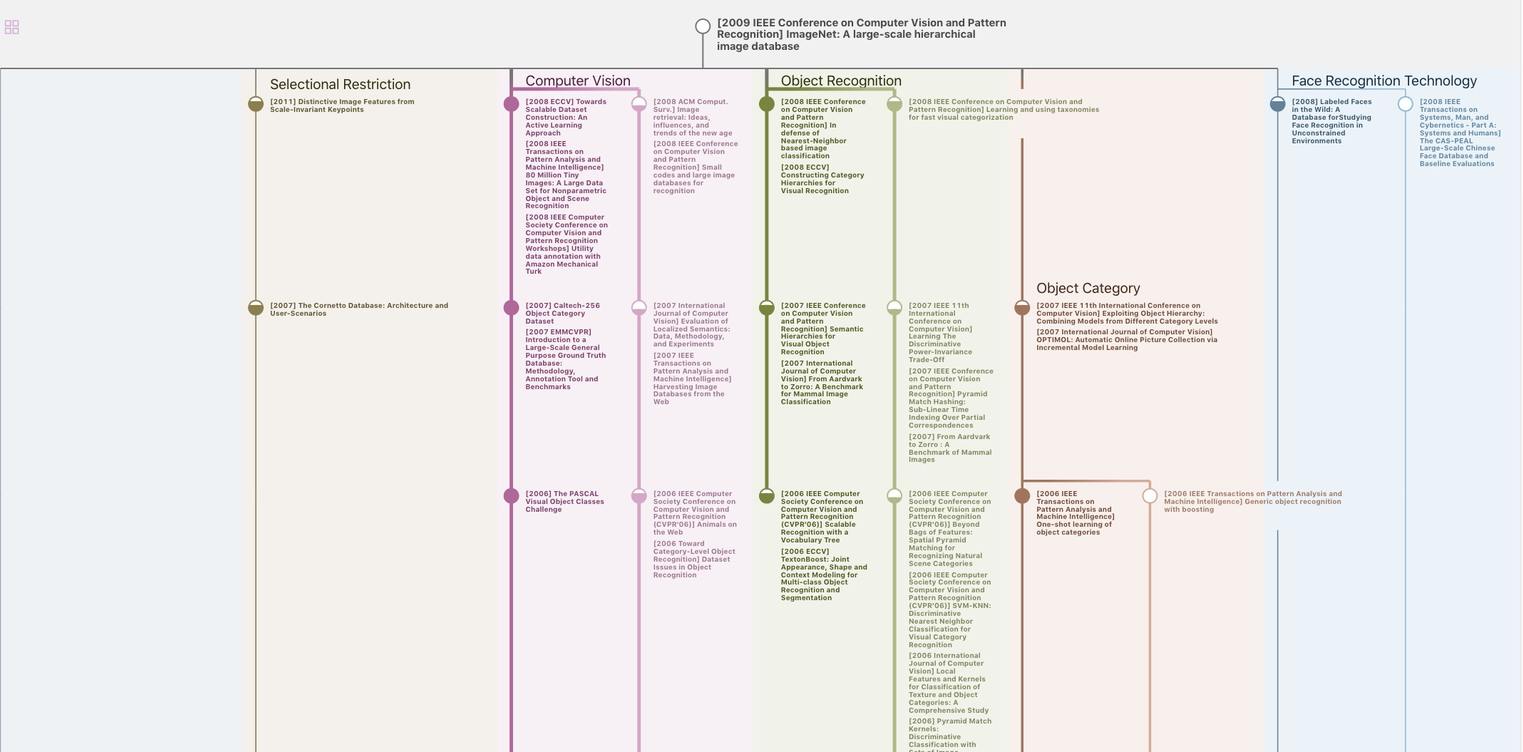
Generate MRT to find the research sequence of this paper
Data Disclaimer
The page data are from open Internet sources, cooperative publishers and automatic analysis results through AI technology. We do not make any commitments and guarantees for the validity, accuracy, correctness, reliability, completeness and timeliness of the page data. If you have any questions, please contact us by email: report@aminer.cn
Chat Paper
Summary is being generated by the instructions you defined