Learning a Bayesian network with multiple latent variables for implicit relation representation
Data Mining and Knowledge Discovery(2024)
摘要
Artificial intelligence applications could be more powerful and comprehensive by incorporating the ability of inference, which could be achieved by probabilistic inference over implicit relations. It is significant yet challenging to represent implicit relations among observed variables and latent ones like disease etiologies and user preferences. In this paper, we propose the BN with multiple latent variables (MLBN) as the framework for representing the dependence relations, where multiple latent variables are incorporated to describe multi-dimensional abstract concepts. However, the efficiency of MLBN learning and effectiveness of MLBN based applications are still nontrivial due to the presence of multiple latent variables. To this end, we first propose the constraint induced and Spark based algorithm for MLBN learning, as well as several optimization strategies. Moreover, we present the concept of variation degree and further design a subgraph based algorithm for incremental learning of MLBN. Experimental results suggest that our proposed MLBN model could represent the dependence relations correctly. Our proposed method outperforms some state-of-the-art competitors for personalized recommendation, and facilitates some typical approaches to achieve better performance.
更多查看译文
关键词
Implicit relation,Bayesian network,Latent variable,Incremental learning,Parallel algorithm
AI 理解论文
溯源树
样例
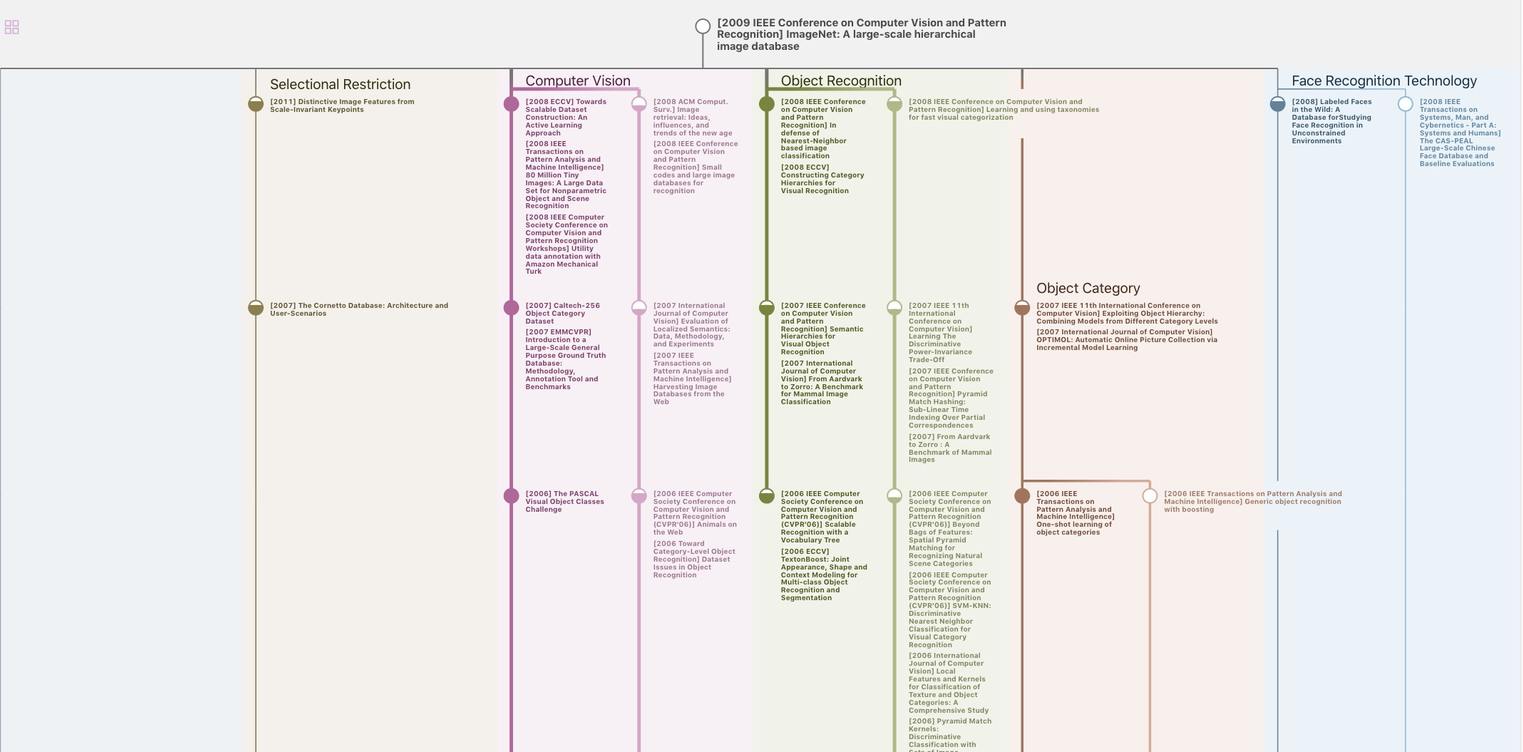
生成溯源树,研究论文发展脉络
Chat Paper
正在生成论文摘要