A Histopathologic Image Analysis for the Classification of Endocervical Adenocarcinoma Silva Patterns Depend on Weakly Supervised Deep Learning
The American Journal of Pathology(2024)
摘要
Twenty-five percent of cervical cancers are classified as endocervical adenocarcinomas (EACs), which comprise a highly heterogeneous group of tumors. A histopathologic risk stratification system known as the Silva pattern system was developed based on morphology. However, accurately classifying such patterns can be challenging. The study objective was to develop a deep learning pipeline (Silva3-AI) that automatically analyzes whole slide image–based histopathologic images and identifies Silva patterns with high accuracy. Initially, a total of 202 patients with EACs and histopathologic slides were obtained from Qilu Hospital of Shandong University for developing and internally testing the Silva3-AI model. Subsequently, an additional 161 patients and slides were collected from seven other medical centers for independent testing. The Silva3-AI model was developed using a vision transformer and recurrent neural network architecture, utilizing multi-magnification patches, and its performance was evaluated based on a class-specific area under the receiver-operating characteristic curve. Silva3-AI achieved a class-specific area under the receiver-operating characteristic curve of 0.947 for Silva A, 0.908 for Silva B, and 0.947 for Silva C on the independent test set. Notably, the performance of Silva3-AI was consistent with that of professional pathologists with 10 years’ diagnostic experience. The findings in this study implicate Silva3-AI, which could predict the Silva pattern in an interpretable manner and achieved performance comparable to that of experienced pathologists. Furthermore, the visualization of prediction heatmaps facilitated the identification of tumor microenvironment heterogeneity, which is known to contribute to variations in Silva patterns.
更多查看译文
AI 理解论文
溯源树
样例
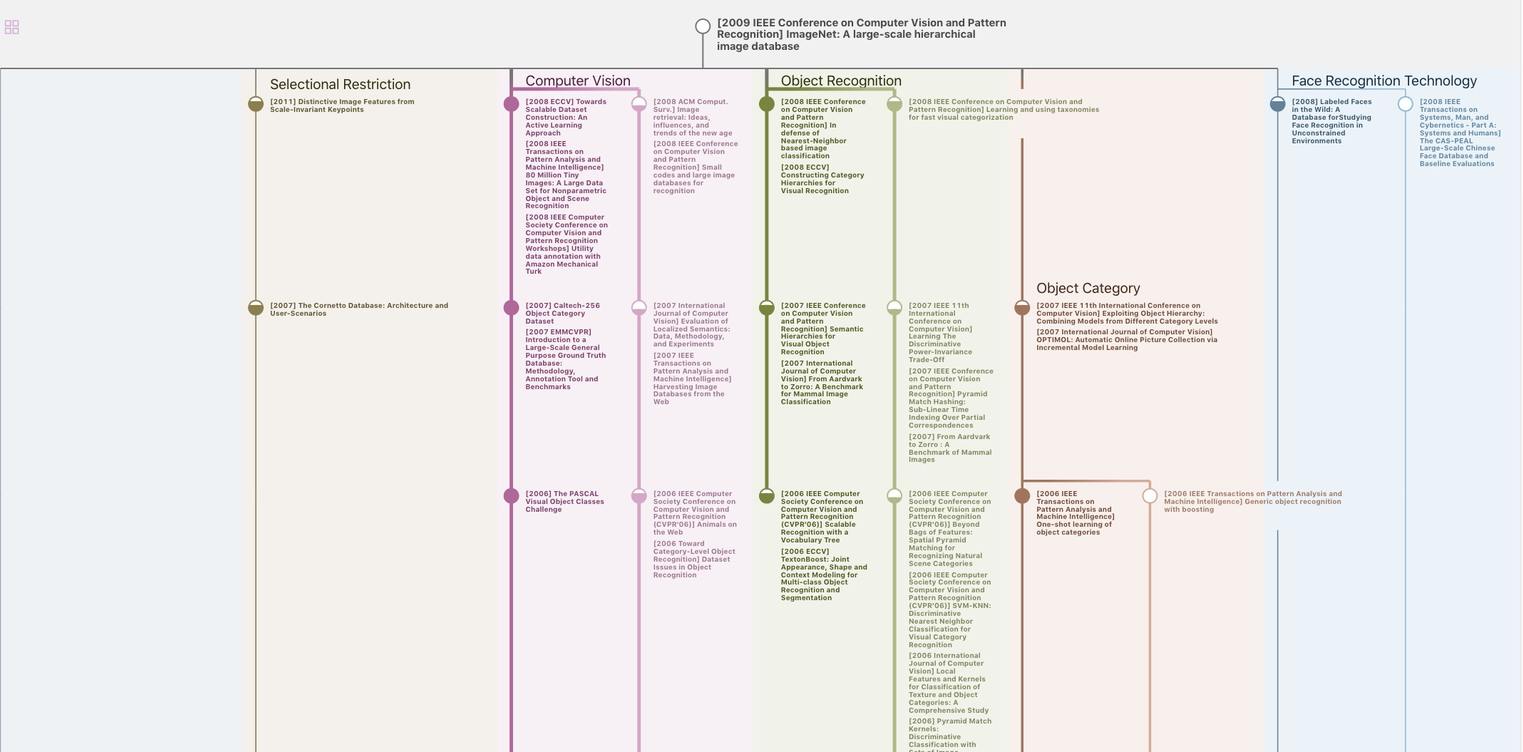
生成溯源树,研究论文发展脉络
Chat Paper
正在生成论文摘要