Re-attentive experience replay in off-policy reinforcement learning
Machine Learning(2024)
摘要
Experience replay, which stores past samples for reuse, has become a fundamental component of off-policy reinforcement learning. Some pioneering works have indicated that prioritization or reweighting of samples with on-policiness can yield significant performance improvements. However, this method doesn’t pay enough attention to sample diversity, which may result in instability or even long-term performance slumps. In this work, we introduce a novel Re-attention criterion to reevaluate recent experiences, thus benefiting the agent from learning about them. We call this overall algorithm, Re-attentive Experience Replay (RAER). RAER employs a parameter-insensitive dynamic testing technique to enhance the attention of samples generated by policies with promising trends in overall performance. By wisely leveraging diverse samples, RAER fulfills the positive effects of on-policiness while avoiding its potential negative influences. Extensive experiments demonstrate the effectiveness of RAER in improving both performance and stability. Moreover, replacing the on-policiness component of the state-of-the-art approach with RAER can yield significant benefits.
更多查看译文
关键词
Reinforcement learning,Experience replay,Sample diversity,Stability
AI 理解论文
溯源树
样例
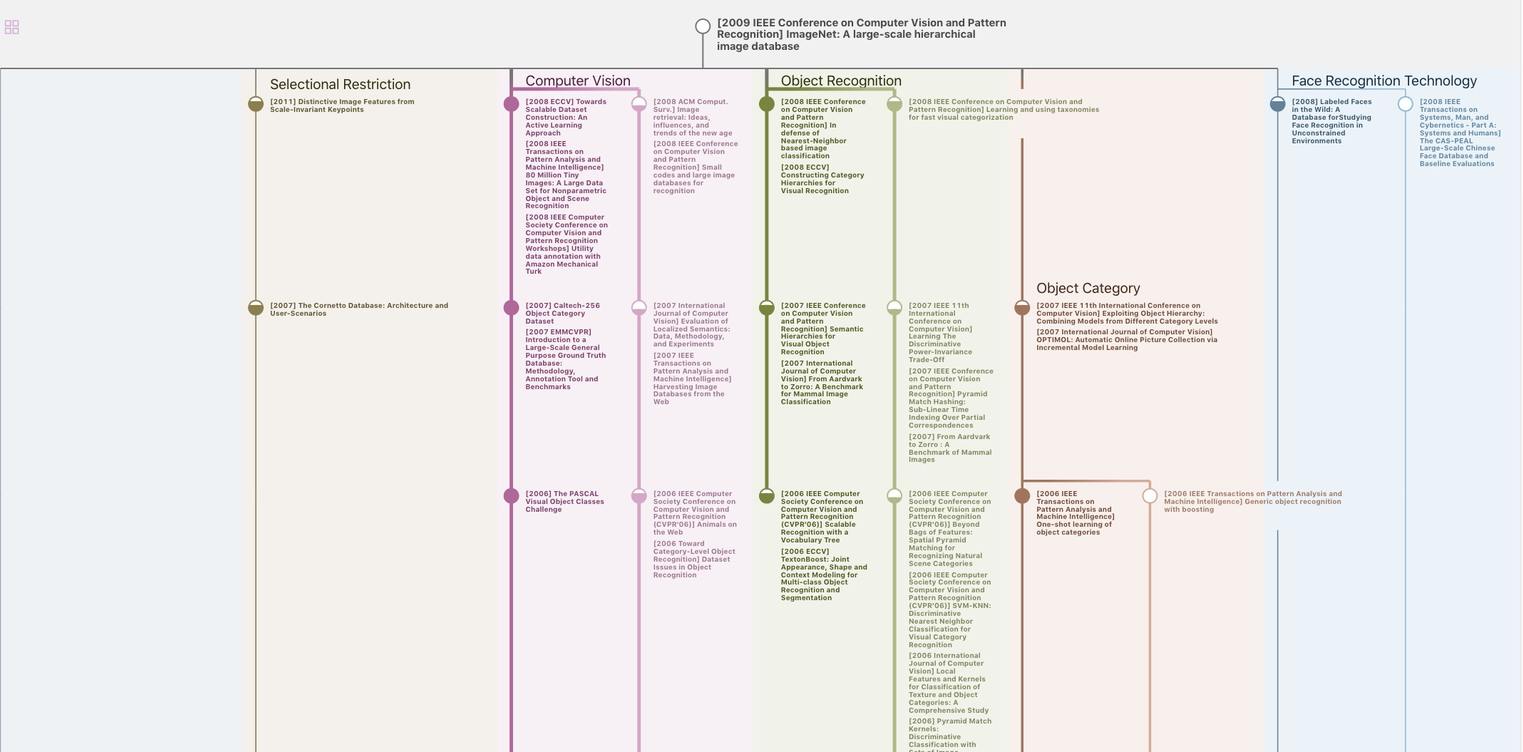
生成溯源树,研究论文发展脉络
Chat Paper
正在生成论文摘要