An Improved Copula-Based Framework for Efficient Global Sensitivity Analysis
WATER RESOURCES RESEARCH(2024)
摘要
Global sensitivity analysis (GSA) enhances our understanding of computational models and simplifies model parameter estimation. VarIance-based Sensitivity analysis using COpUlaS (VISCOUS) is a variance-based GSA framework. The advantage of VISCOUS is that it can use existing model input-output data (e.g., water model parameters-responses) to estimate the first- and total-order Sobol' sensitivity indices. This study improves VISCOUS by refining its handling of marginal densities of the Gaussian mixture copula model (GMCM). We then evaluate VISCOUS using three types of generic functions relevant to water system models. We observe that its performance depends on function dimension, input-output data size, and non-identifiability. Function dimension refers to the number of uncertain input factors analyzed in GSA, and non-identifiability refers to the inability to estimate GMCM parameters. VISCOUS proves powerful in estimating first-order sensitivity with a small amount of input-output data (e.g., 200 in this study), regardless of function dimension. It always ranks input factors correctly in both first- and total-order terms. For estimating total-order sensitivity, it is recommended to use VISCOUS when the function dimension is not very high (e.g., less than 20) due to the challenge of producing sufficient input-output data for accurate GMCM inferences (e.g., more than 10,000 data). In cases where all input factors are equally important (a rarity in practice), VISCOUS faces non-identifiability issues that impact its performance. We provide a didactic example and an open-source Python code, pyVISCOUS, for broader user adoption.
更多查看译文
关键词
global sensitivity analysis,Sobol' sensitivity index,given-data approach,computationally efficient,Gaussian mixture copula model,non-identifiability
AI 理解论文
溯源树
样例
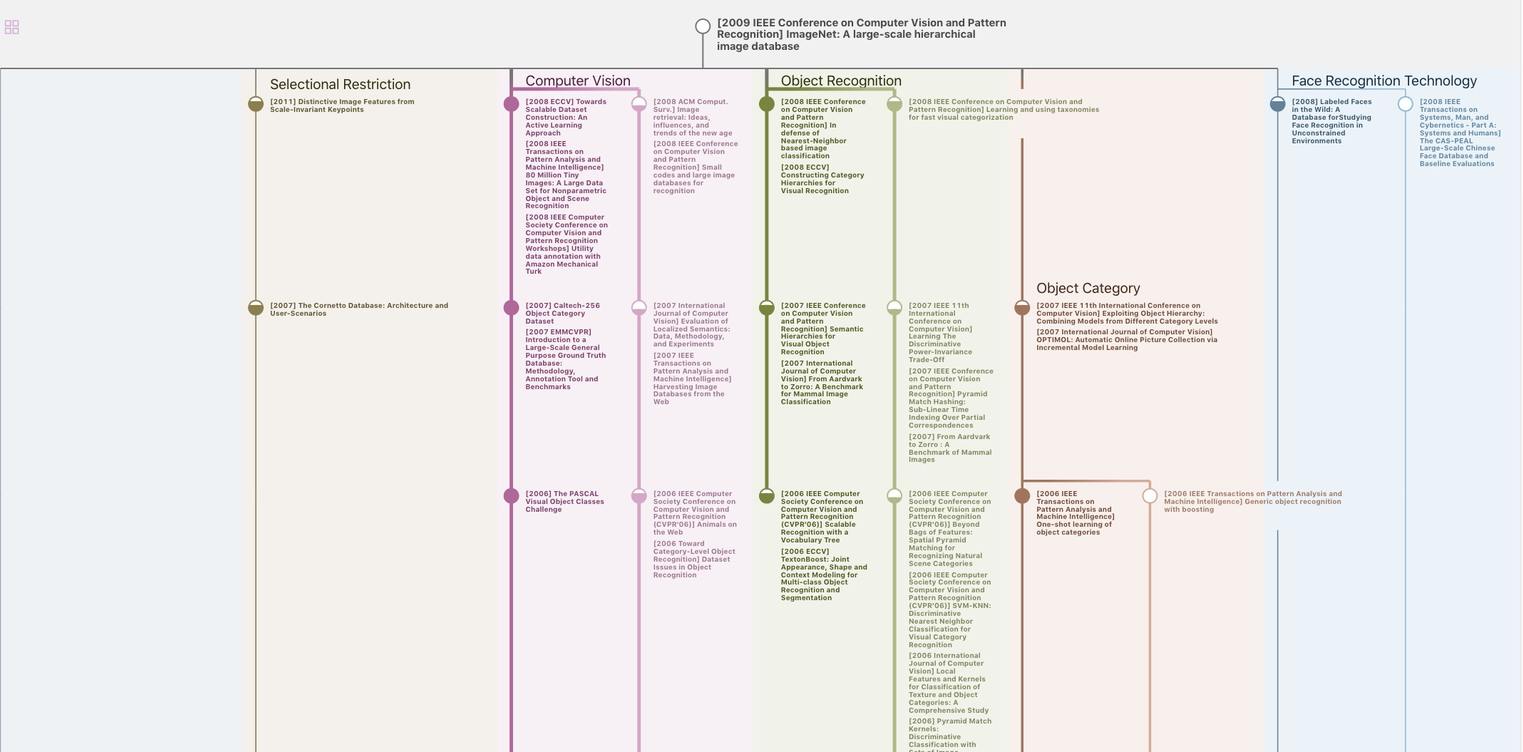
生成溯源树,研究论文发展脉络
Chat Paper
正在生成论文摘要