Predicting the Ages of Galaxies with an Artificial Neural Network
Monthly Notices of the Royal Astronomical Society(2024)
关键词
methods :dataanalysis,galaxies: stellarcontent,galaxies: fundamental parameters
AI 理解论文
溯源树
样例
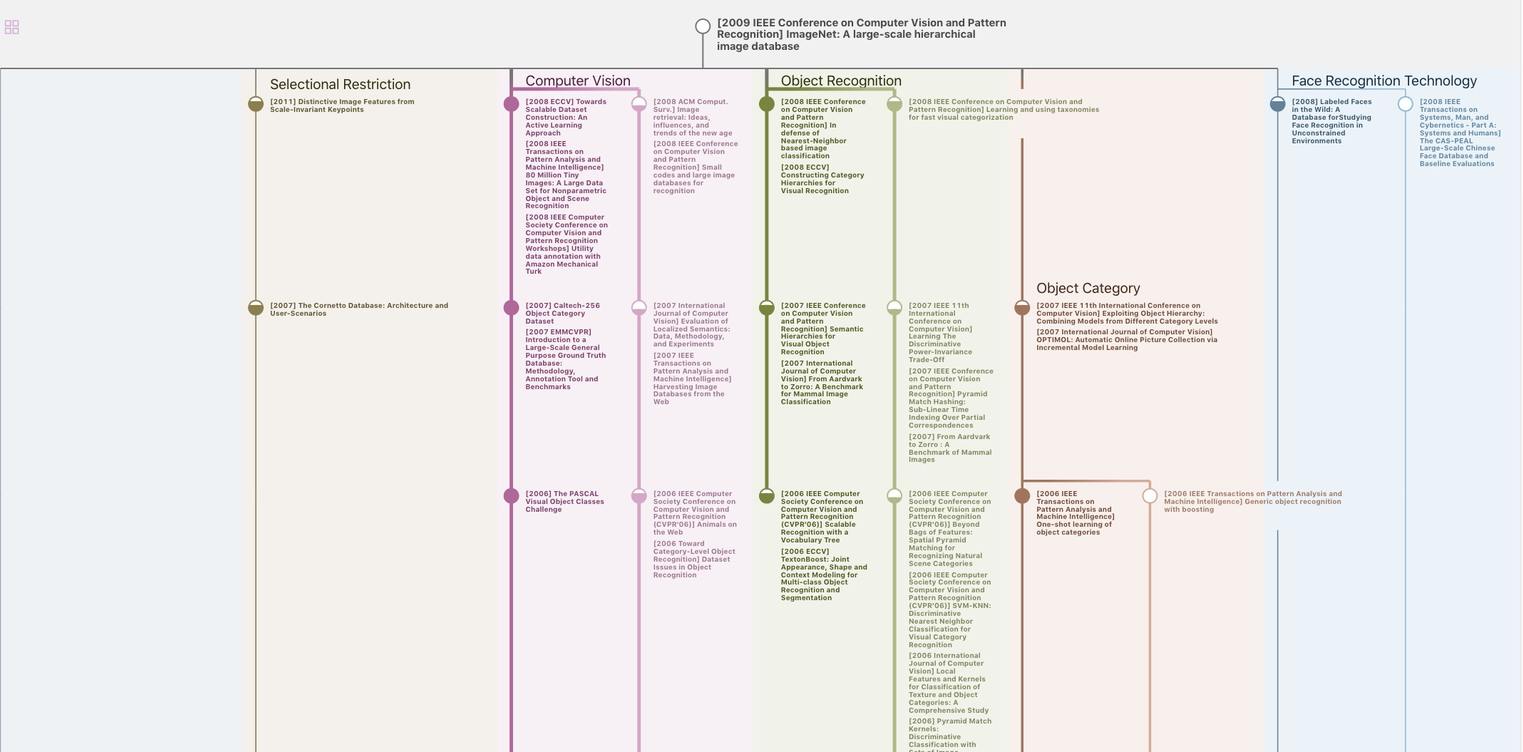
生成溯源树,研究论文发展脉络
Chat Paper
正在生成论文摘要