Safe Reinforcement Learning for Lane-Changing with Comprehensive Analysis of Safety Detection.
2023 IEEE 26th International Conference on Intelligent Transportation Systems (ITSC)(2023)
摘要
Reinforcement learning (RL) is an experience-driven and data-driven learning method that can well solve the lane-changing problems. However, because traditional RL methods rely on exploration of the environment, the agent is prone to unsafe behavior during exploration, leading to disastrous consequences. It prevents its application in systems that must ensure users' safety, such as autonomous driving. To address this issue, we propose a safe reinforcement learning (safe RL) method, define safety detection parameters, and comprehensively analyze the impact of the proposed parameters on the safety and driving efficiency during lane-changing. Based on the above parameters, a safety detection model is constructed, which can guide the agent to explore in the safe action space, enhance the agent's safety while exploration, and increase the learning convergence speed. In this paper, we use high-fidelity simulation software to simulate and validate the conventional RL method and the proposed safe RL method, respectively. According to the experiments, the safe RL approach suggested in our work dramatically lowers the collision rate during learning and speeds up learning convergence. Moreover, during evaluation, the collision rate of the proposed method can be reduced to 0%, while the average speed of the vehicle is reduced by only about 1 m/s. The results are available as videos at https://youtube.com/playlist?list=PLPFN_7Uwj6PioPAjP4ZBbJPyEAZELkfPD.
更多查看译文
关键词
Autonomous driving,safe reinforcement learning,lane-changing,decision-making and control
AI 理解论文
溯源树
样例
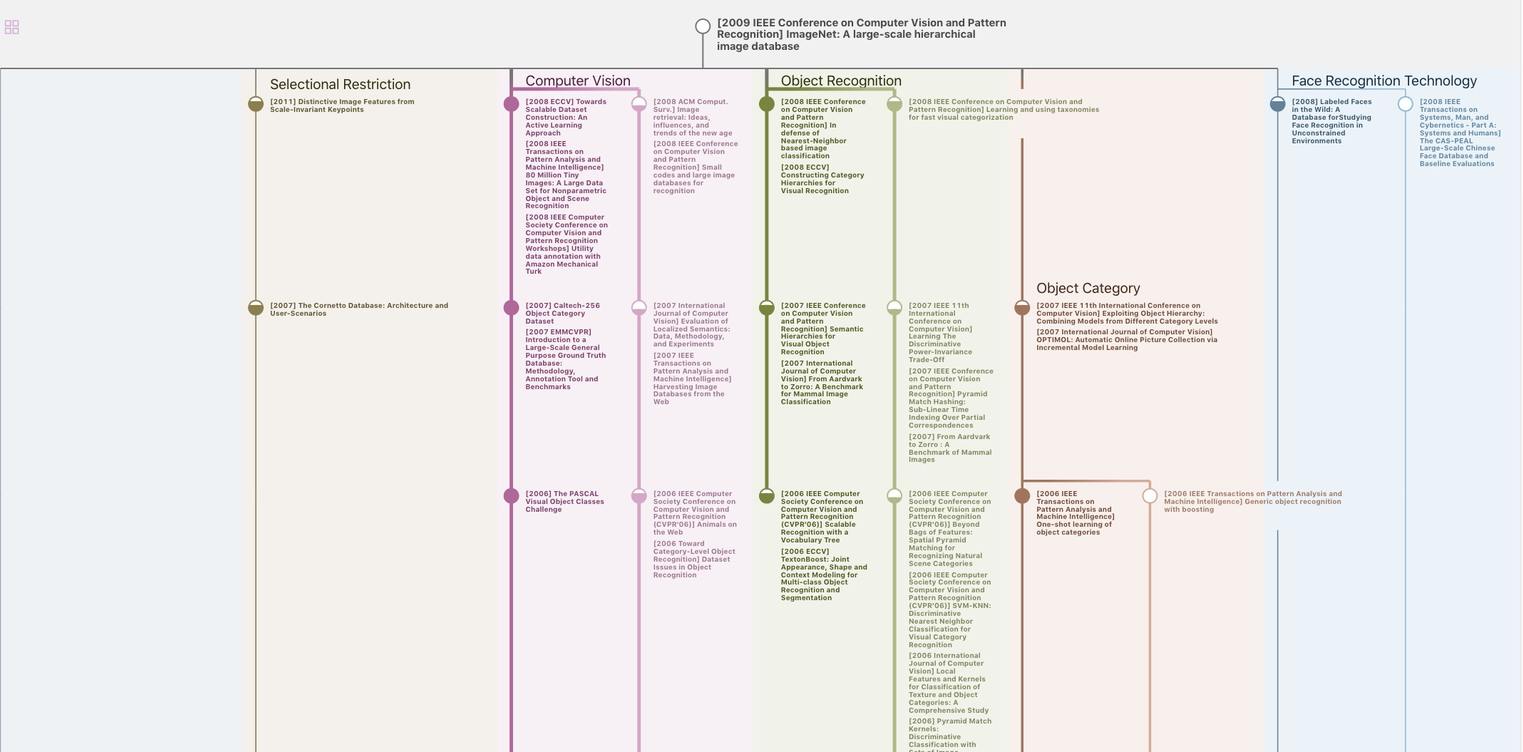
生成溯源树,研究论文发展脉络
Chat Paper
正在生成论文摘要