Model Predictive Bang-Bang Controller Synthesis via Approximate Value Functions
arxiv(2024)
摘要
In this paper, we propose a novel method for addressing Optimal Control
Problems (OCPs) with input-affine dynamics and cost functions. This approach
adopts a Model Predictive Control (MPC) strategy, wherein a controller is
synthesized to handle an approximated OCP within a finite time horizon. Upon
reaching this horizon, the controller is re-calibrated to tackle another
approximation of the OCP, with the approximation updated based on the final
state and time information. To tackle each OCP instance, all non-polynomial
terms are Taylor-expanded about the current time and state and the resulting
Hamilton-Jacobi-Bellman (HJB) PDE is solved via Sum-of-Squares (SOS)
programming, providing us with an approximate polynomial value function that
can be used to synthesize a bang-bang controller.
更多查看译文
AI 理解论文
溯源树
样例
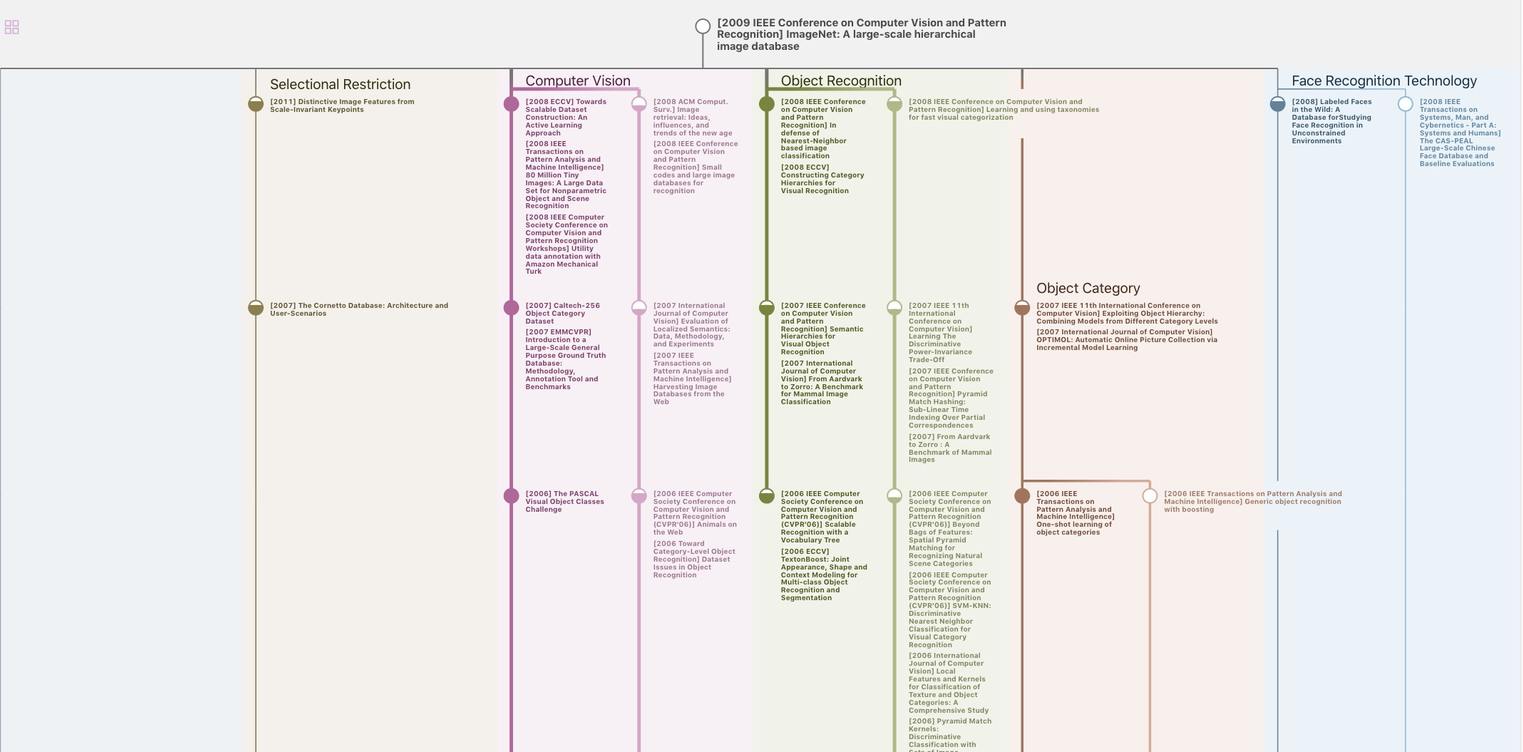
生成溯源树,研究论文发展脉络
Chat Paper
正在生成论文摘要