Physics-Informed Multitemporal Ensemble Learning for Near Real-Time Precipitation Estimates from Himawari-8/-9
IEEE GEOSCIENCE AND REMOTE SENSING LETTERS(2024)
Key words
Precipitation,Atmospheric modeling,Spatial resolution,Data models,Atmospheric measurements,Indexes,Real-time systems,Ensemble learning,multitemporal,near real-time (NRT),physics-informed,precipitation
AI Read Science
Must-Reading Tree
Example
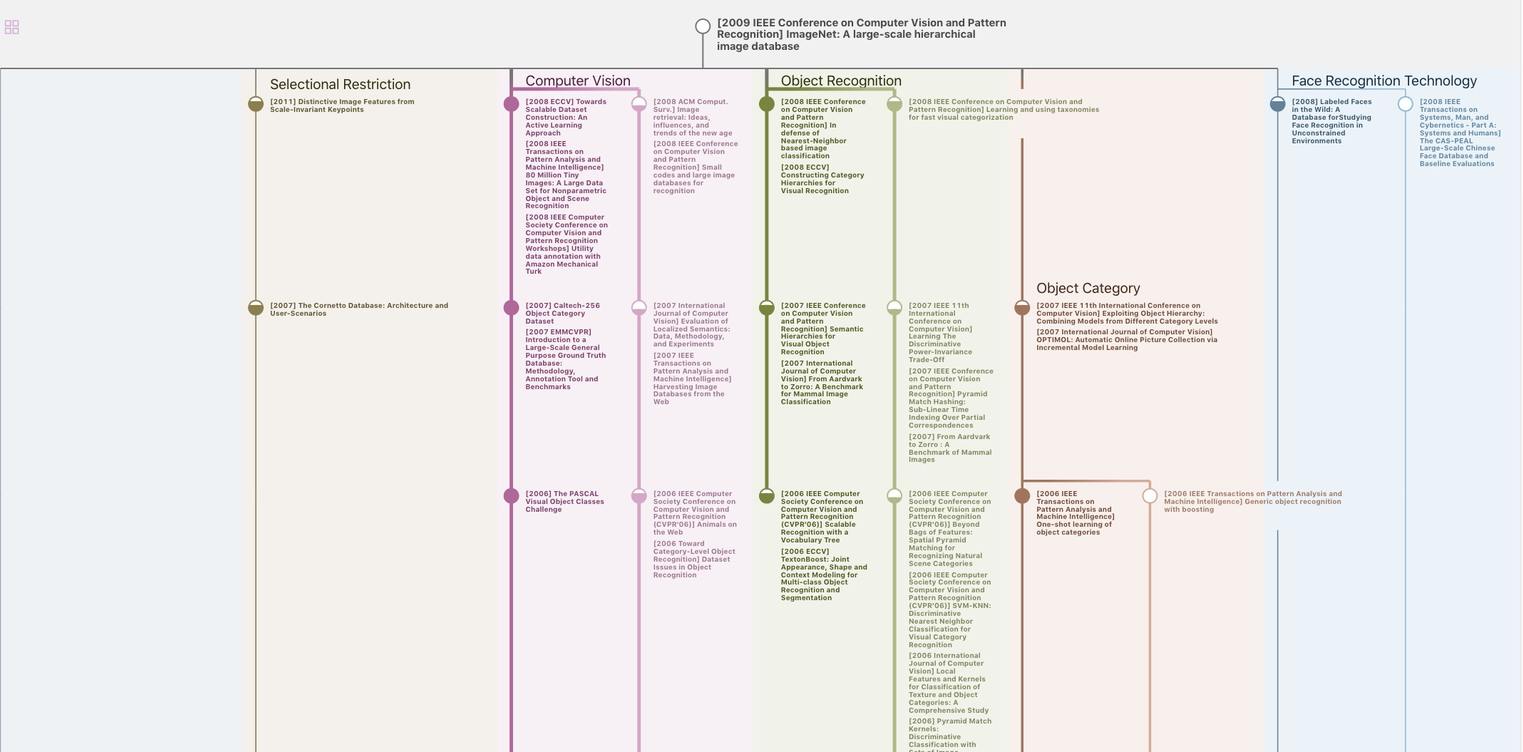
Generate MRT to find the research sequence of this paper
Chat Paper
Summary is being generated by the instructions you defined