Fine-Tuned Language Models Generate Stable Inorganic Materials as Text
CoRR(2024)
摘要
We propose fine-tuning large language models for generation of stable
materials. While unorthodox, fine-tuning large language models on text-encoded
atomistic data is simple to implement yet reliable, with around 90
structures obeying physical constraints on atom positions and charges. Using
energy above hull calculations from both learned ML potentials and
gold-standard DFT calculations, we show that our strongest model (fine-tuned
LLaMA-2 70B) can generate materials predicted to be metastable at about twice
the rate (49
prompting's inherent flexibility, our models can simultaneously be used for
unconditional generation of stable material, infilling of partial structures
and text-conditional generation. Finally, we show that language models' ability
to capture key symmetries of crystal structures improves with model scale,
suggesting that the biases of pretrained LLMs are surprisingly well-suited for
atomistic data.
更多查看译文
关键词
generative model,large language model,stable materials,AI for science
AI 理解论文
溯源树
样例
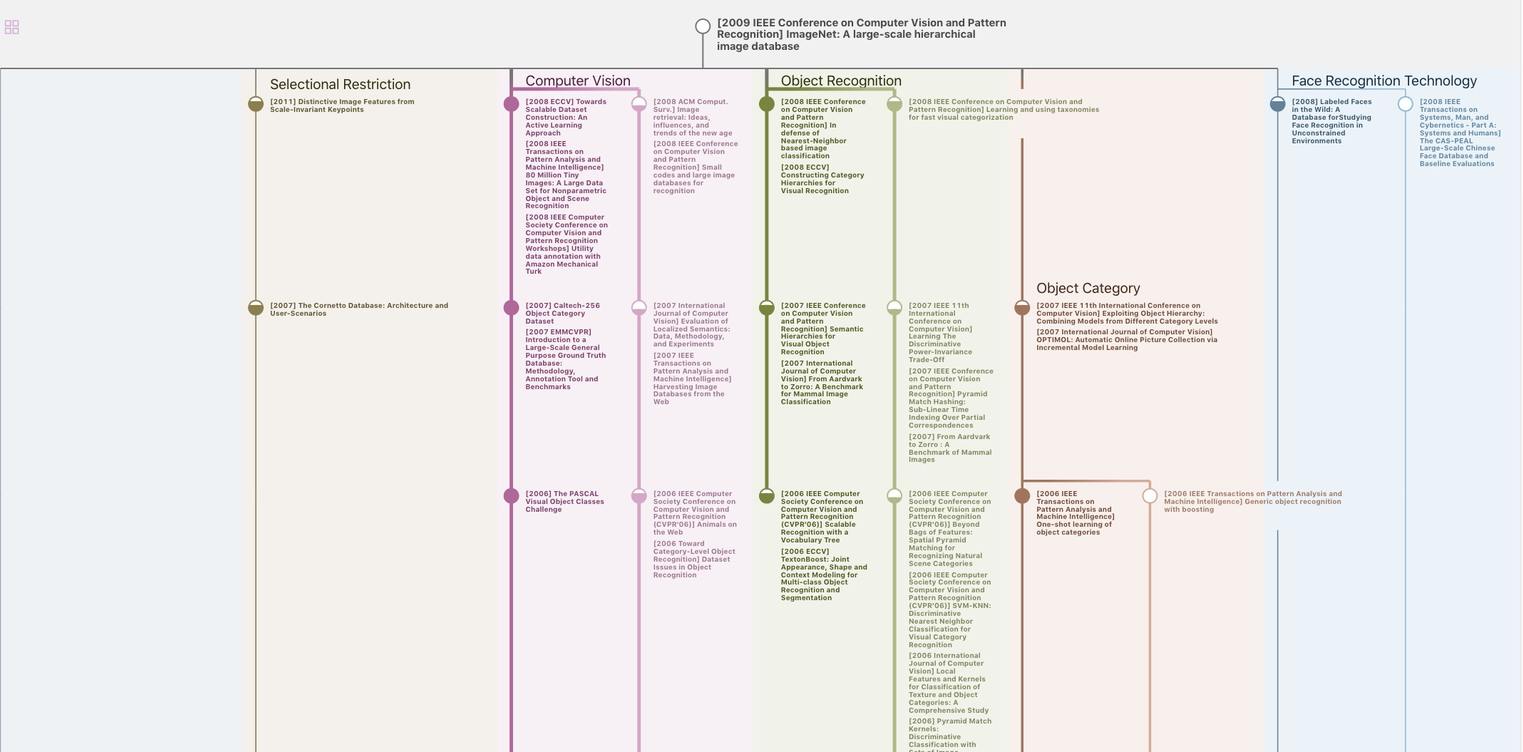
生成溯源树,研究论文发展脉络
Chat Paper
正在生成论文摘要