Small Gastric Polyp Detection Based on the Improved YOLOv5
MULTIMEDIA TOOLS AND APPLICATIONS(2024)
Key words
Polyp,Deep learning,YOLOv5,Small target,Detection
AI Read Science
Must-Reading Tree
Example
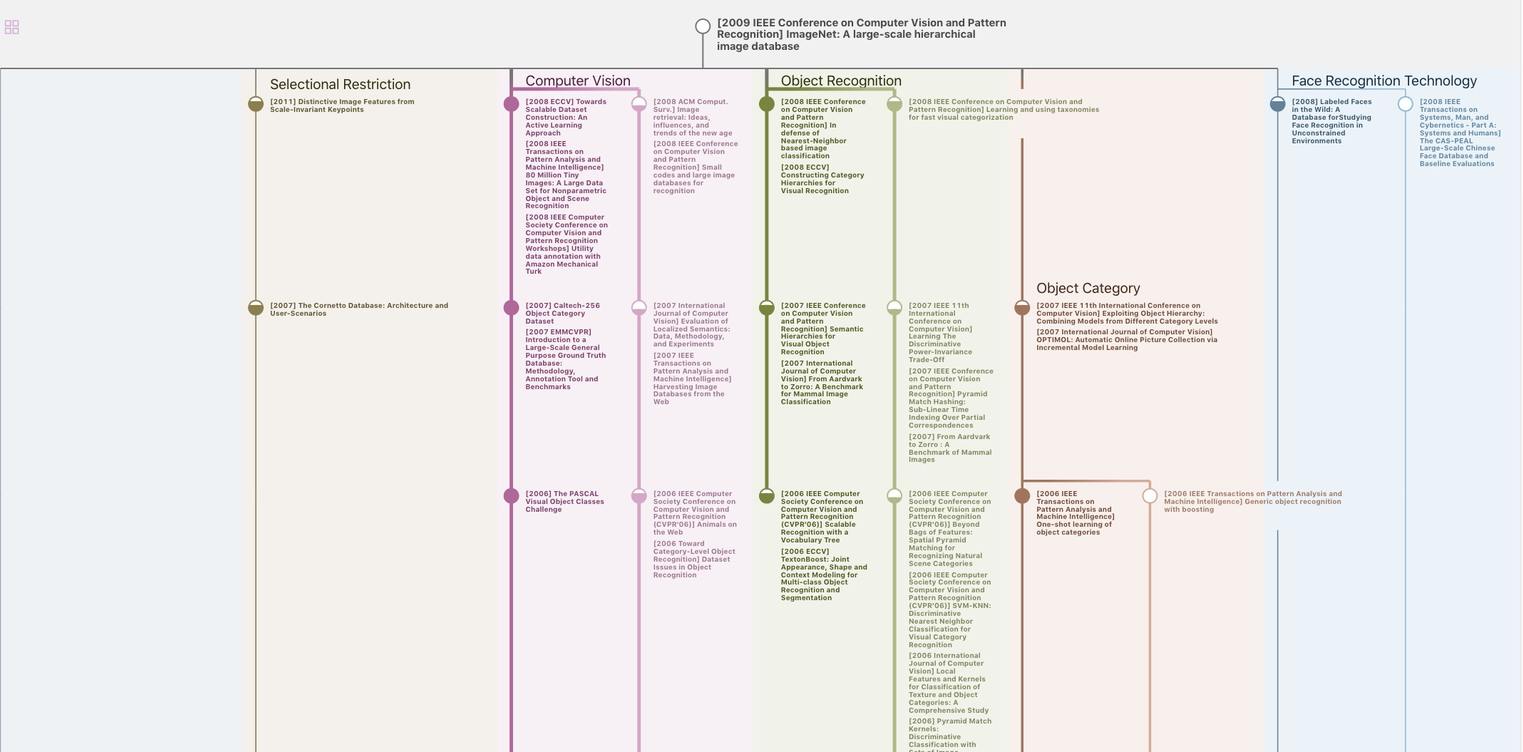
Generate MRT to find the research sequence of this paper
Chat Paper
Summary is being generated by the instructions you defined