Non-contact vehicle weight identification method based on explainable machine learning models and computer vision
Journal of Civil Structural Health Monitoring(2024)
摘要
This paper first explores an alternative non-contact method based on computer vision and explainable machine learning (EML) models to identify and predict vehicle overload cost-effectively. First, 1108 sets of data are extracted from traditional contact measurements, non-contact measurements (Optical Character Recognition and thermal imaging), and literature collection to establish a novel and comprehensive database. The missing value imputation and the randomized search are then selected to find the optimal ML model for further analysis. Moreover, two typical theoretical and five ML models are adopted to evaluate the optimal model’s performance. Finally, the sHapley Additive exPlanations (SHAP) is applied to interpret the influence factors of the optimal ML model. The results indicate that the divided length between the tire and the ground is the most significant input feature, followed by the tire’s inflation pressure, the section height of tire, and the radius. Finally, the proposed model has great application potential for enhancing the efficiency of non-contact vehicle weight-in-motion (WIM) weighing.
更多查看译文
关键词
Weight-in-motion,Non-contact identification,Machine learning,SHapley Additive exPlanations,Tire-roadway contact force
AI 理解论文
溯源树
样例
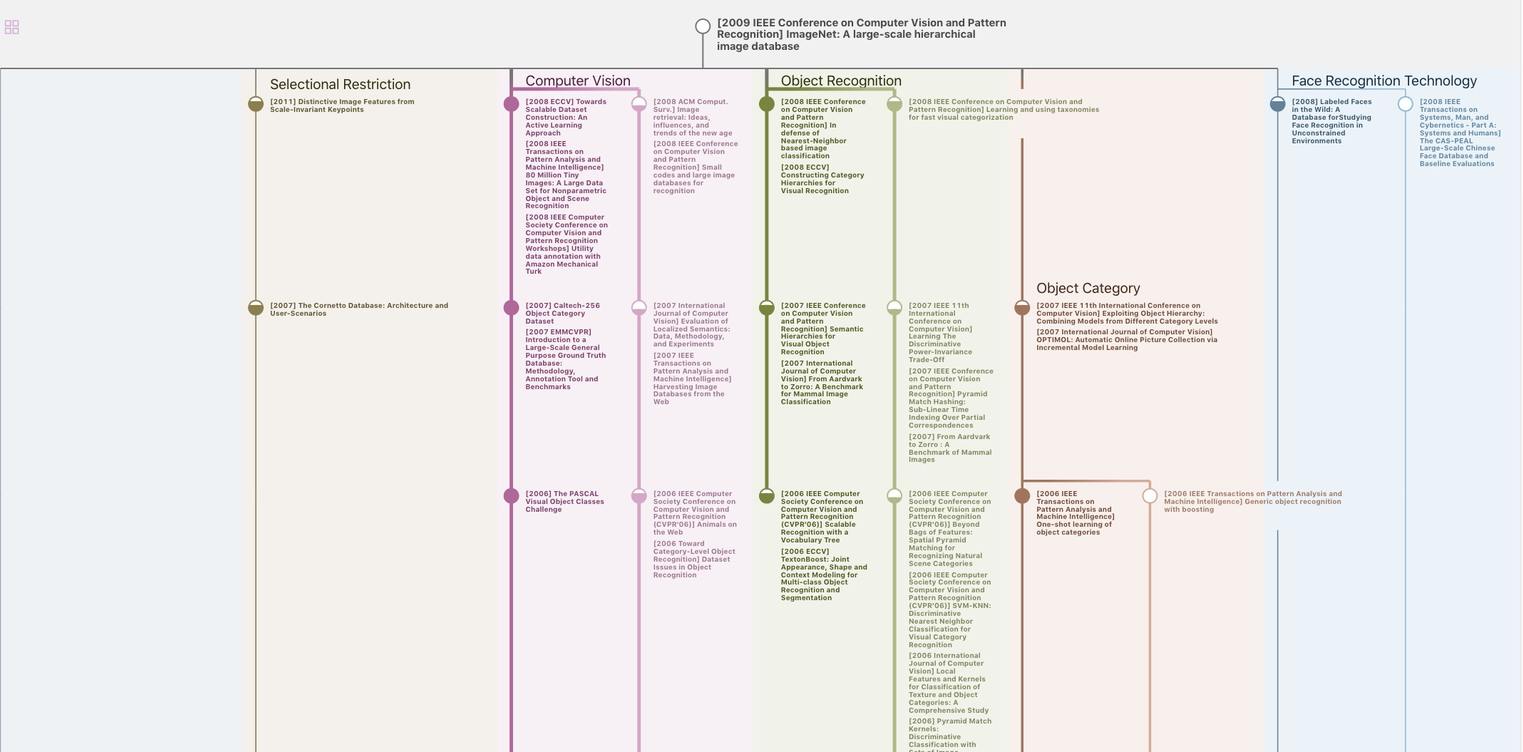
生成溯源树,研究论文发展脉络
Chat Paper
正在生成论文摘要