Feature Attribution-Guided Contrastive Learning: Mitigating Lexical Bias in Toxic Speech Detection
2023 International Conference on New Trends in Computational Intelligence (NTCI)(2023)
摘要
Automatic detection methods for toxic speech can potentially curb the dissemination of offensive, abusive, hateful, and other toxic speech on social media. However, these methods often show bias by overreacting to words such as identity terms, profanity, and swear words that occur in non-toxic speech and erroneously classifying them as toxic speech. Recent research has attempted to regularise relevant biased words in predefined lexicons to mitigate their impact on model classification. However, this approach faces two challenges. Firstly, words such as pro-fanity and swear words in biased words play a crucial role in the model's ability to identify toxic speech, and excessive suppression of these words using regularization methods can detrimentally affect the performance of the classification model. Secondly, due to the limitations of regularization techniques, existing methods rely on manually constructed biased word dictionaries, which can only mitigate bias associated with identity-related terms. This bias should not significantly impact hate speech prediction. It is challenging to encompass lexical biases such as profanity and swear words that are specific to different datasets beyond identity terms To address the above challenges, this paper proposes a novel feature attribution-guided contrastive learning method. The method consists of two repeated steps across epochs. In each epoch, first identifies keywords in sentences that are crucial for predicting toxicity through feature attribution. Then it applies contrastive learning to separate samples that have common toxic keywords but different labels. Experiments show that our approach can mitigate lexical bias in toxic speech detection without any data augmentation or prior knowledge and achieve competitive performance gains.
更多查看译文
关键词
Toxic Speech,Bias Mitigation,Contrastive Learning,Feature Attribution
AI 理解论文
溯源树
样例
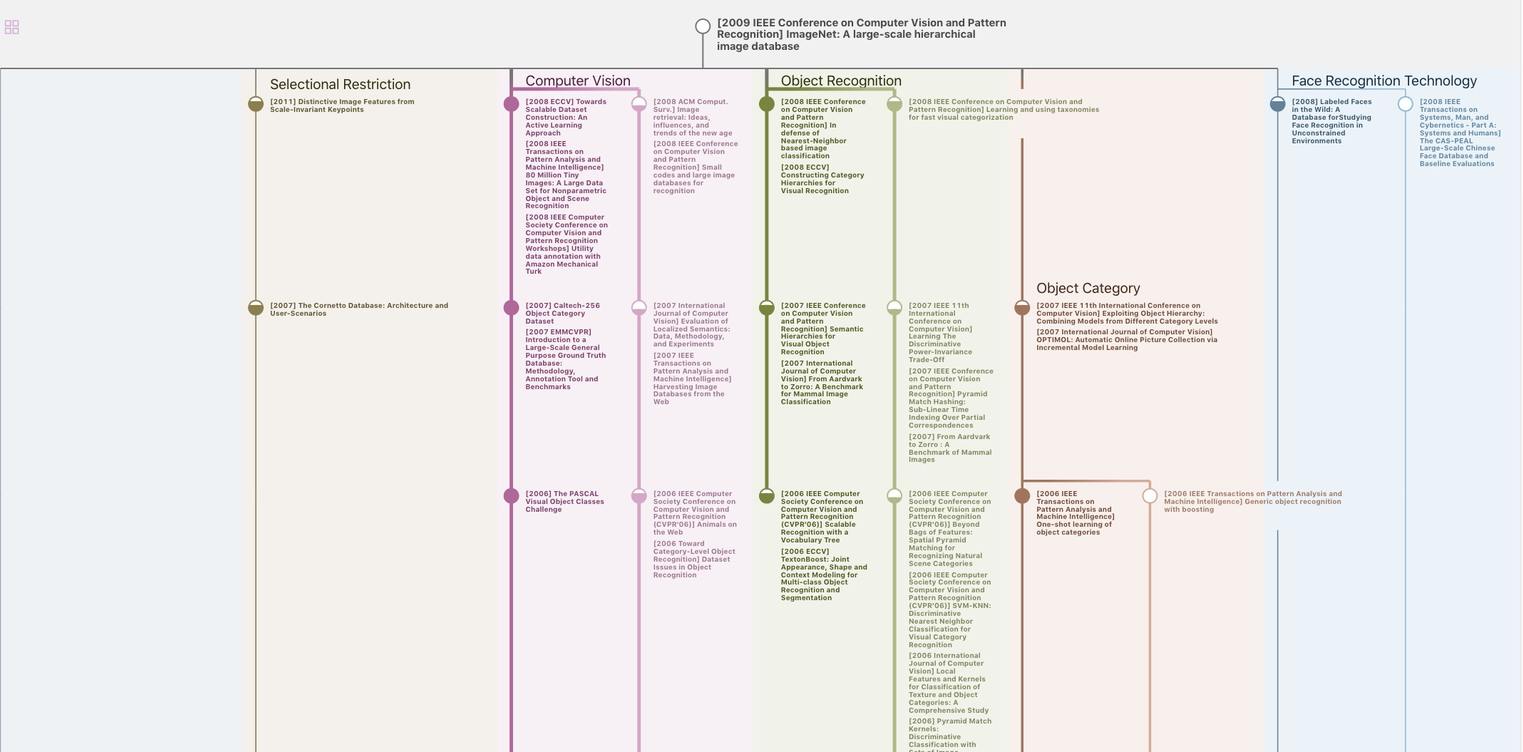
生成溯源树,研究论文发展脉络
Chat Paper
正在生成论文摘要