Model-based and Data-driven Calibration of the X-ray CT Image based on Proton Radiographies
2022 IEEE Nuclear Science Symposium and Medical Imaging Conference (NSS/MIC)(2022)
摘要
In proton beam therapy the semi-empirical calibration of the X-ray CT image introduces inaccuracies into the treatment planning. The promise of proton imaging is to potentially eliminate these inaccuracies by (1) replacing the X-ray CT image with the proton CT image based on tomographic image reconstruction of many proton radiographies or (2) adjusting the semi-empirical calibration of the X-ray CT image based on optimization algorithms for few proton radiographies. Both (1) and (2) are based on the model of the proton radiography (in water equivalent thickness, WET) as a line integral of the proton CT image (in relative stopping power, RSP) along the estimation of the proton trajectory. However, this model (i.e., the forward-projection) is not perfectly consistent to the measurement (i.e., the proton radiography). Depending on the detector configurations, in clinical data these intrinsic inconsistencies can result in the same order of magnitude as the calibration inaccuracies that are intended to be eliminated. The purpose of this work is to overcome the intrinsic limitation of the model-based optimization with a novel data-driven approach relaying on supervised machine learning. The availability of the ground truth CT image in simulations is exploited. Realistic proton radiographies are simulated relying on pre-clinical data. Results demonstrate the potential capability of the data-driven approach. A tuning of the hyperparameters and the number of layers of the convolutional neural network is ongoing. Further investigations involving different data and different detector configurations are foreseen.
更多查看译文
关键词
Computed Tomography,X-ray CT,X-ray CT Images,Convolutional Neural Network,Treatment Plan,Supervised Learning,Image Reconstruction,Hyperparameter Tuning,Proton Beam,Proton Therapy,Detector Configuration,Stopping Power,Model-based Optimization,Standard Curve,Mean Absolute Error,Object Of Interest,Projection Angle
AI 理解论文
溯源树
样例
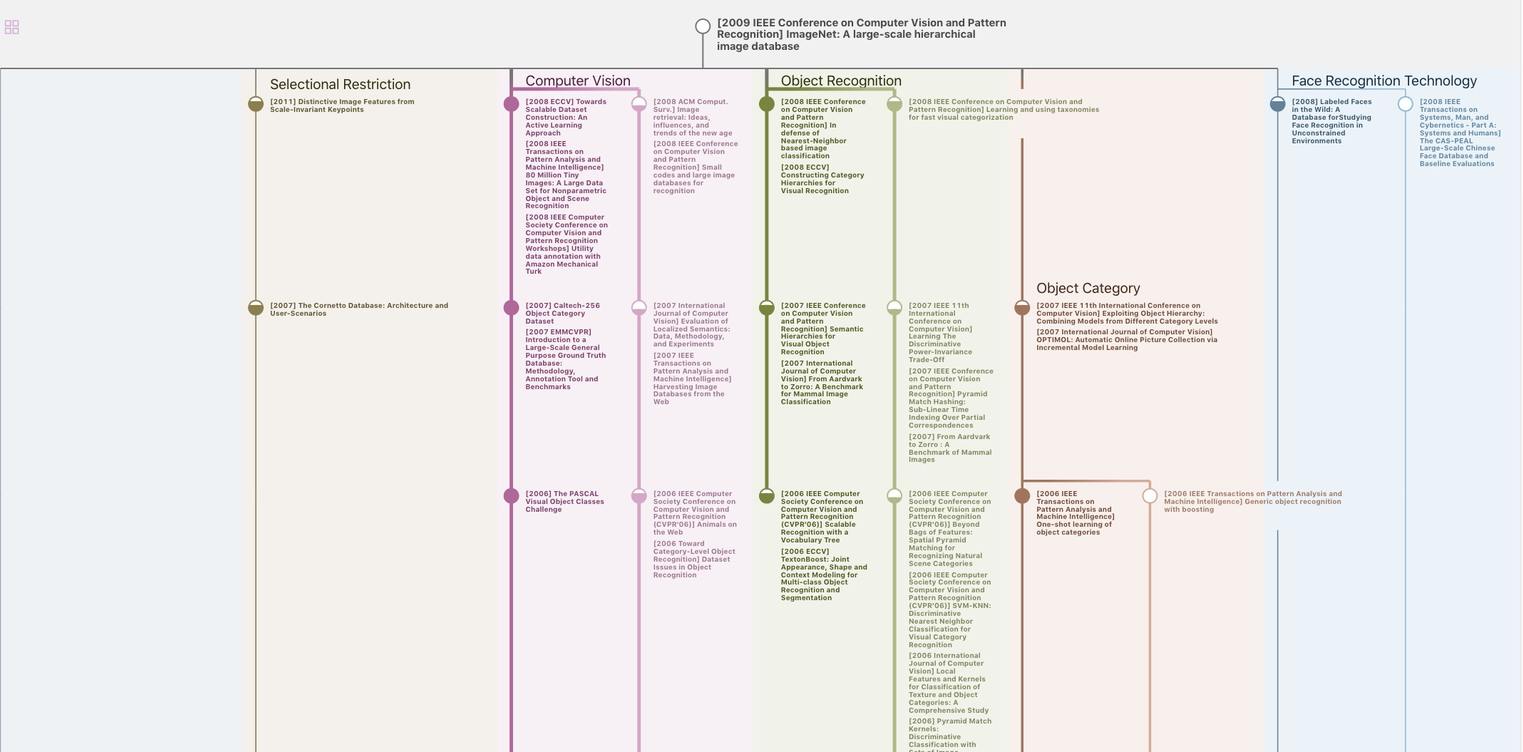
生成溯源树,研究论文发展脉络
Chat Paper
正在生成论文摘要