Graph-enhanced context aware framework for session-based recommendation
Neurocomputing(2024)
摘要
Session-based recommendation (SBR) aims to recommend the next item according to the short-term historical interaction by the anonymous user. It is a popular method to transform sessions into graph architectures, and apply graph neural networks (GNN) to extract features and make recommendation in SBR. The previous GNN-based methods only focus on the propagation mechanism based on the primitive item graph, ignoring the hierarchical context in items and sessions. Besides, the problem of data sparsity is exacerbated in SBR due to the short-term length of session. Contrastive learning (CL) based on self-supervised augmentation shows potentials to tackle this problem. The existing CL-based SBR methods only consider from user aspect in the CL task, which is similar to the cross-entropy loss and ignores the explicit information of items. To address the above defects, we propose a novel Graph-Enhanced Context Aware Framework (GECAF), which can fully investigate the item context and session context through a more fine-grained graph construction. Especially, GECAF propose an item-based contrastive learning method to enrich semantic information in the item context. Extensive experiments on three datasets show the effectiveness of our model, which achieves state-of-art performance for the session-based recommendation.
更多查看译文
关键词
Session-based recommendation,Contrastive learning,Graph embedding learning
AI 理解论文
溯源树
样例
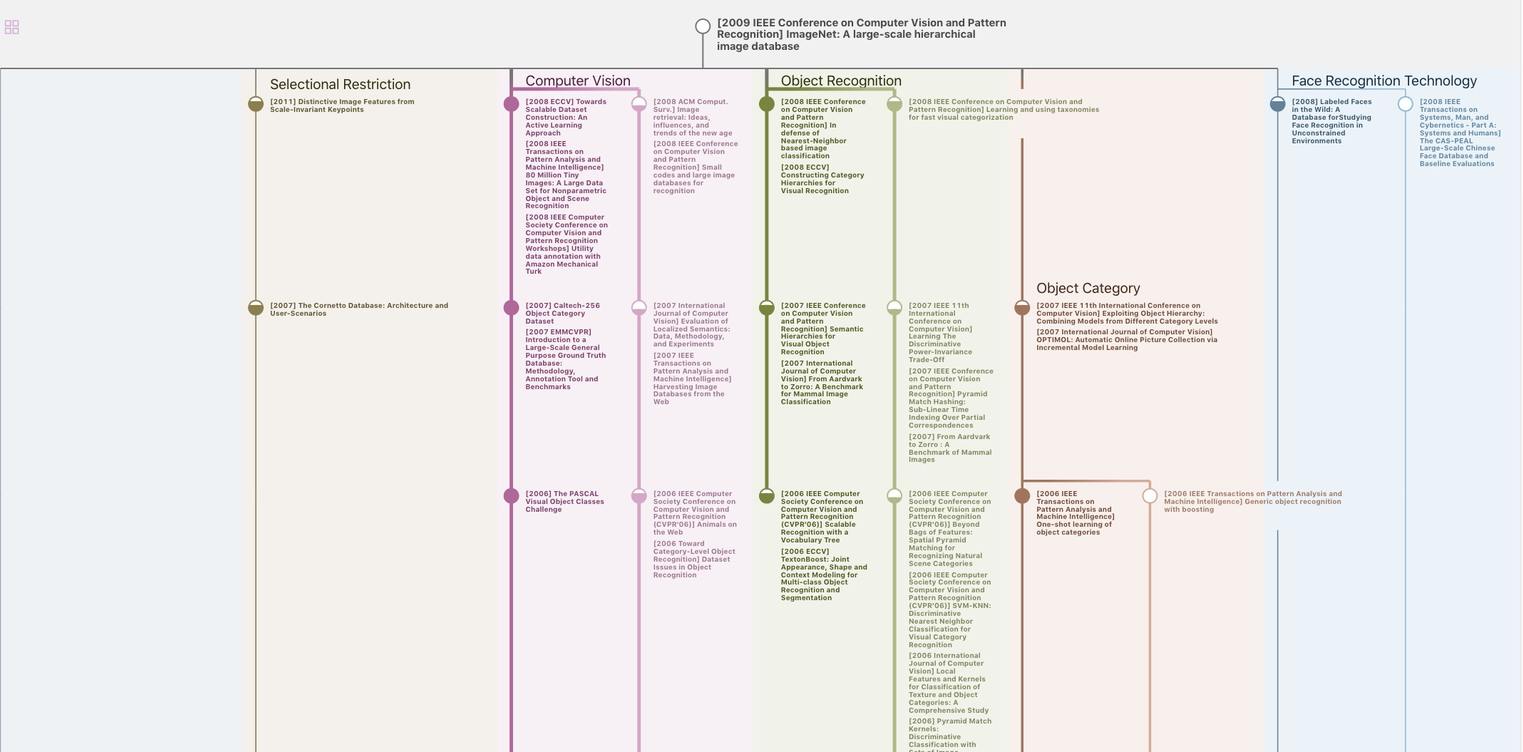
生成溯源树,研究论文发展脉络
Chat Paper
正在生成论文摘要