Neural Network-Based Small Cursor Detection for Embedded Assistive Technology
The Visual Computer(2024)
Key words
Assistive device testbed,Machine learning,Embedded systems,Small object detection,Human–computer interface
AI Read Science
Must-Reading Tree
Example
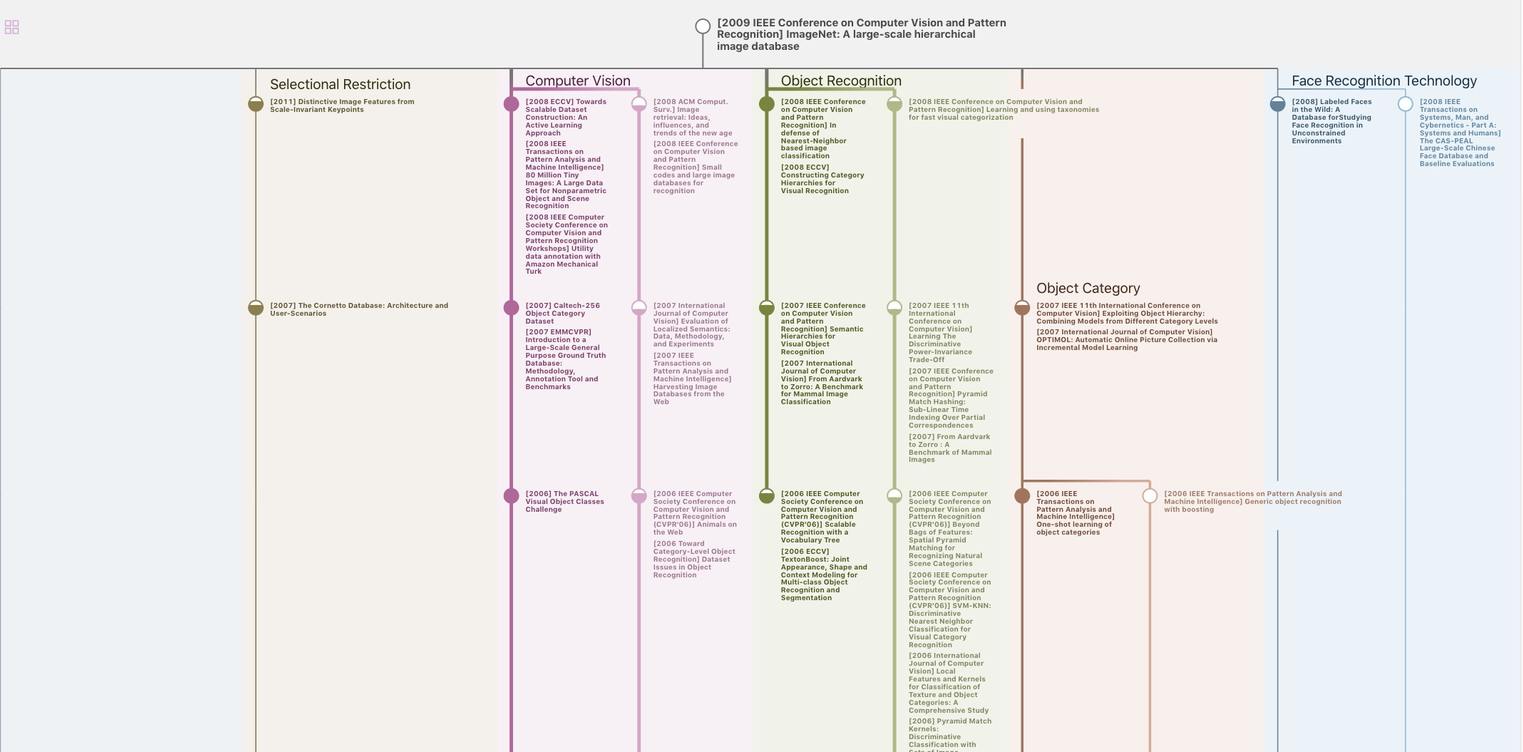
Generate MRT to find the research sequence of this paper
Chat Paper
Summary is being generated by the instructions you defined