Effective Face Inpainting by Conditional Generative Adversarial Network.
2023 IEEE International Conference on Big Data (BigData)(2023)
摘要
In the paper, we propose a two-stage face-inpainting approach based on conditional generative adversarial networks. In the first stage, a deep-learning model is trained for predicting face landmarks. It also dynamically adjusts the penalty value of the loss function based on the view-degree of a face to improve the ability of predicting high view-degree faces. In the second stage, masked face images and their corresponding face landmarks are concatenated to form the condition of training a conditional Generative Adversarial Network (GAN) for inpainting the masked face. If an input masked image is a nearly-frontal face, an additional procedure for face symmetry processing will be performed before the image is input into the inpainting model. The experimental results show that the proposed training method in the first stage can effectively enhance the robustness of the face-landmark prediction model and reduce the impact of data imbalance, thereby improving the effect of later face inpainting. They also show that the proposed face-inpainting model in the second stage can better maintain the geometric structures and symmetric outlooks of inpainted faces than previous ones.
更多查看译文
关键词
Conditional Generative Adversarial Networks,Face Inpainting,Face-landmark Prediction,Symmetry Processing
AI 理解论文
溯源树
样例
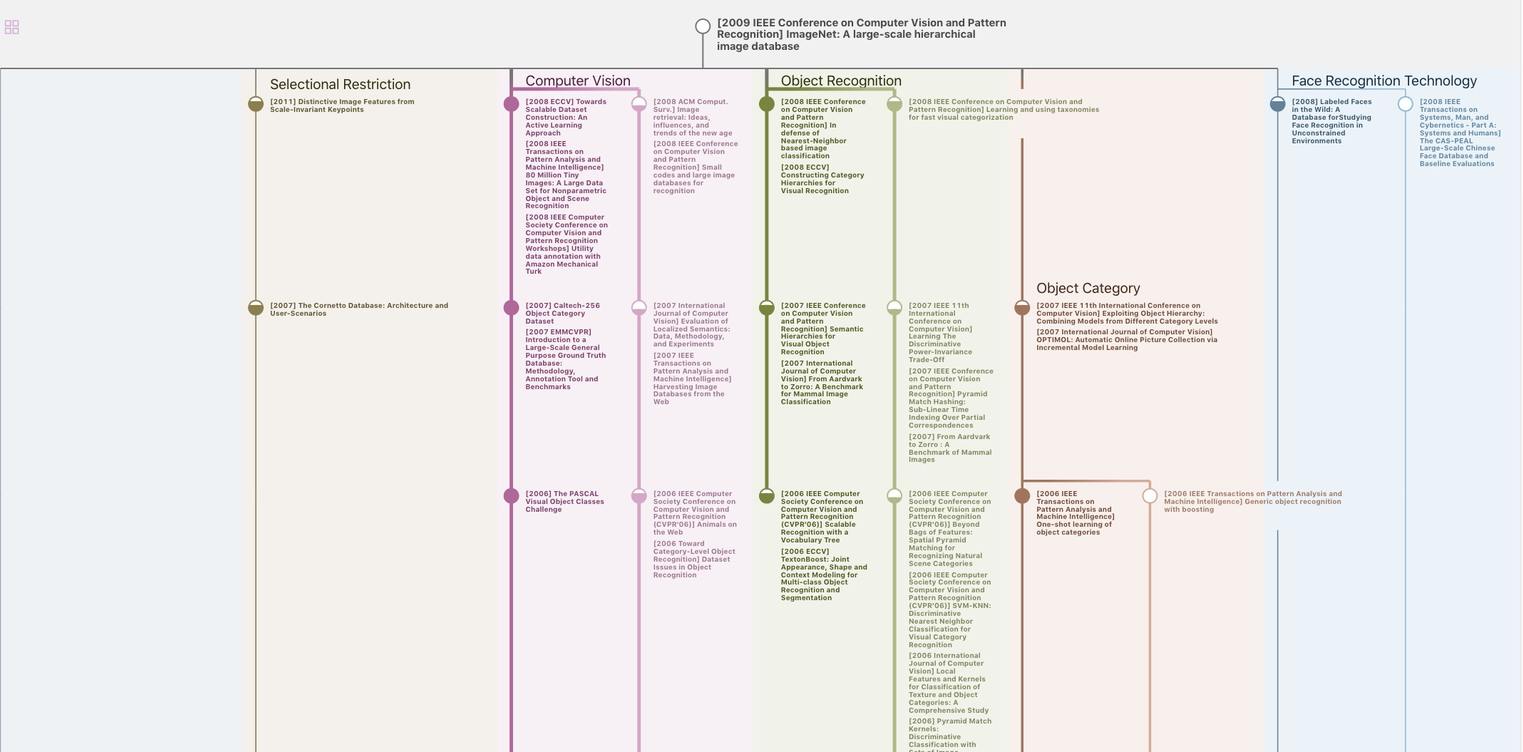
生成溯源树,研究论文发展脉络
Chat Paper
正在生成论文摘要