Functional Arm Movement Classification in Stroke Survivors Using Deep Learning with Accelerometry Data.
2023 IEEE International Conference on Big Data (BigData)(2023)
摘要
The rehabilitation of the upper extremity (UE) plays a pivotal role in the recovery process for stroke survivors. Despite the routine practice of UE rehabilitation, assessing its effectiveness poses a significant challenge. Evaluation methods vary, ranging from clinical assessments of UE motor skills and patient feedback to the utilization of wearable technology equipped with accelerometer or gyro sensors. In this study, we employed deep learning approaches such as Multi-Layer Perceptron, Long Short-term Memory, and Gated Recurrent Units, in conjunction with data from accelerometry sensors embedded in wrist-wearable Inertial Measurement Units. Our findings demonstrate the successful differentiation between functional and non-functional UE movements in individuals recovering from strokes with 90% and 74% of classification accuracy for intra-subject and inter-subject models, respectively. The methodologies employed in this study exhibit consistency and reliability, providing precise outcomes for individual and acceptable outcomes for cross-participant evaluations. Notably, our advanced models directly utilized raw accelerometry data instead of manual extracted features that was often used in traditional machine learning algorithms. These innovative techniques offer cost-effective and adaptable tools for monitoring UE functionality in real-world settings. The insights derived from this study hold the potential to be transformative in tailoring rehabilitation strategies for adults affected by strokes.
更多查看译文
关键词
deep learning,upper extremity,stroke,rehabilitation
AI 理解论文
溯源树
样例
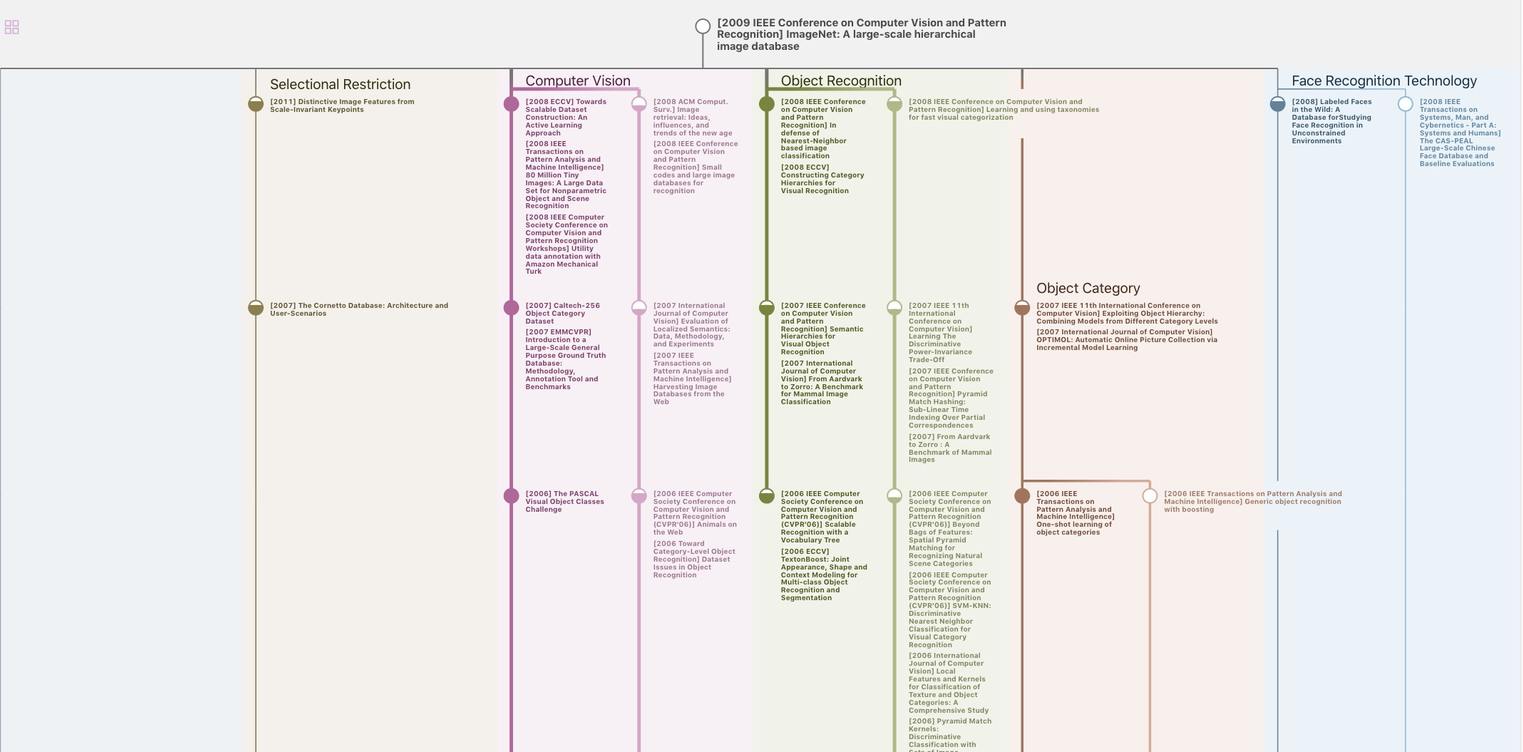
生成溯源树,研究论文发展脉络
Chat Paper
正在生成论文摘要