Deep Learning-Based Coseismic Deformation Estimation From InSAR Interferograms
IEEE TRANSACTIONS ON GEOSCIENCE AND REMOTE SENSING(2024)
摘要
Accurate automated extraction of coseismic deformation from synthetic aperture radar (SAR) data can be challenging owing to interference from inherent atmospheric noise. Particularly, the limited displacement of small-to-moderate earthquakes (Mw < 6.5) can easily be obscured by phase errors and/or noise. To address this issue, we developed an autoencoder model based on a deep learning framework (i.e., Pytorch) to automate the accurate extraction of coseismic displacement from interferometric SAR (InSAR) interferograms. We constructed a training dataset using simulated interferograms. Our trained model performed well for interferograms with real noise. When applied to worldwide real earthquakes of various rupture styles, the model produced clear coseismic displacement with less noise and a better fit to coseismic fault models compared to the differential InSAR (D-InSAR) method without noise correction. Additionally, it achieved co-seismic deformation similar to popular InSAR time series and Global Navigation Satellite System (GNSS) methods. The approach will enhance the proceduralization and popularization of InSAR applications in earthquake monitoring, providing improved constraints on the kinematic characteristics of earthquakes.
更多查看译文
关键词
Autoencoder,coseismic displacement,deep learning,error removal,time-series interferometric synthetic aperture radar (InSAR)
AI 理解论文
溯源树
样例
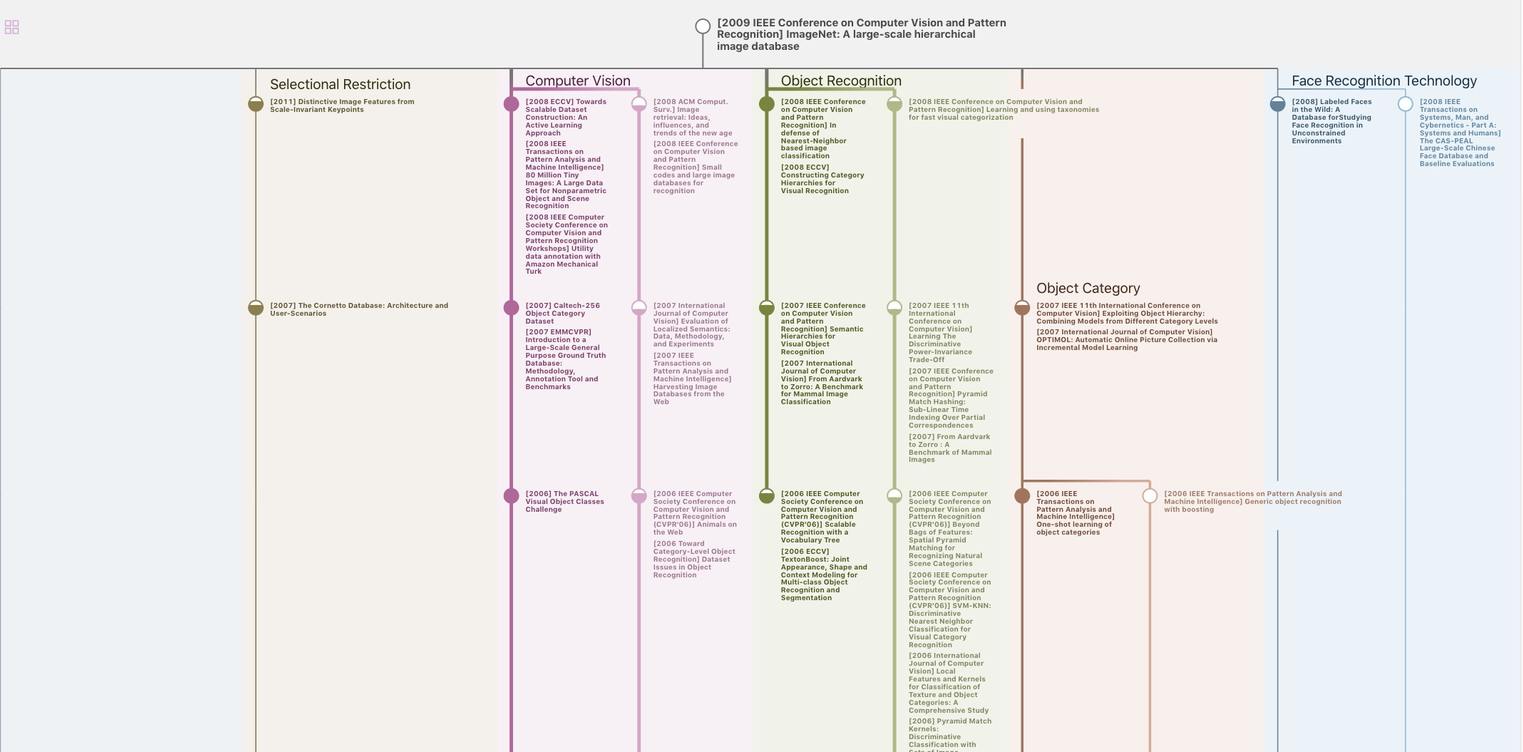
生成溯源树,研究论文发展脉络
Chat Paper
正在生成论文摘要