An RRAM-Based Computing-in-Memory Architecture and Its Application in Accelerating Transformer Inference
IEEE TRANSACTIONS ON VERY LARGE SCALE INTEGRATION (VLSI) SYSTEMS(2024)
摘要
Deep neural network (DNN)-based transformer models have demonstrated remarkable performance in natural language processing (NLP) applications. Unfortunately, the unique scaled dot-product attention mechanism and intensive memory access pose a significant challenge during inference on power-constrained edge devices. One emerging solution to this challenge is computing-in-memory (CIM), which uses memory cells for logic computation to reduce data movement and overcome the memory wall. However, existing CIM designs do not support high-precision computations, such as floating-point operations, which are essential for NLP applications. Furthermore, CIM architectures require complex control modules and costly peripheral circuits to harness the full potential of in-memory computation. Hence, this article proposes a scalable RRAM-based in-memory floating-point computation architecture (RIME) that uses single-cycle nor, nand, and minority logic to implement in-memory floating-point operations. RIME features efficient parallel and pipeline capabilities with a centralized control module and a simplified peripheral circuit to eliminate data movement during computation. Furthermore, the article proposes pipelined implementations of matrix-matrix multiplication (MatMul) and softmax functions, enabling the construction of a transformer accelerator based on RIME. Extensive experimental results show that compared with GPU-based implementation, the RIME-based transformer accelerator improves timing efficiency by 2.3 $\times$ and energy efficiency by 1.7 $\times$ without compromising inference accuracy.
更多查看译文
关键词
Accelerator,computing-in-memory (CIM),energy efficiency,resistive random access memory (RRAM),scalability,transformer
AI 理解论文
溯源树
样例
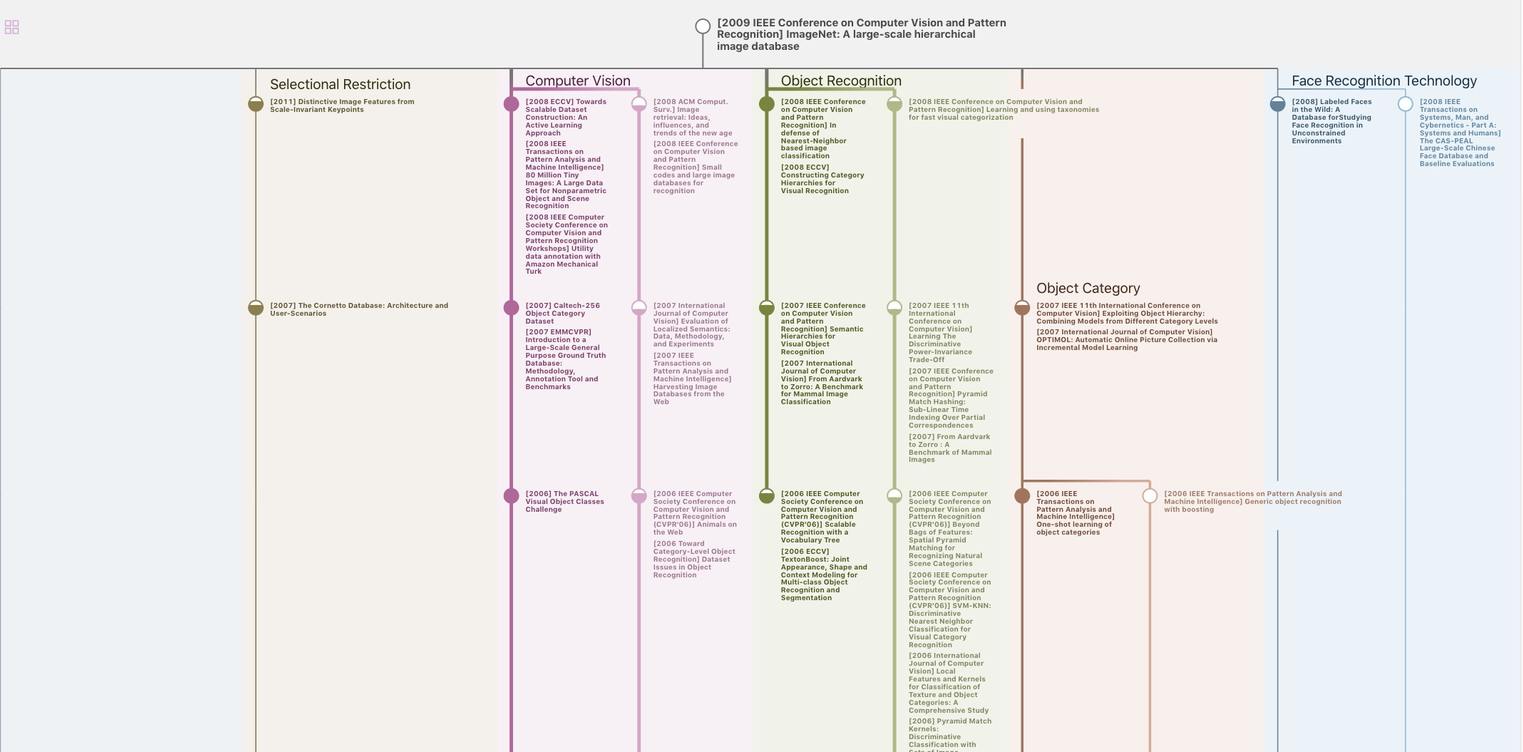
生成溯源树,研究论文发展脉络
Chat Paper
正在生成论文摘要