An Efficient and Adaptive Reconstructive Homogeneous Block-Based Local Tensor Robust PCA for Feature Extraction of Hyperspectral Images
IEEE JOURNAL OF SELECTED TOPICS IN APPLIED EARTH OBSERVATIONS AND REMOTE SENSING(2024)
摘要
Model-driven tensor robust principal component analysis (TRPCA) has been widely applied to feature extraction of hyperspectral images (HSIs) and successfully protected two-dimensional spectral contextual information. Nevertheless, the current TRPCA-based feature extraction methods still destroy the underlying spectral and spatial-spectral joint contextual features. Moreover, these global iterative algorithms commonly ignore the heterogeneity of different real-world regions, increase the calculation burden, and improve practice operating time. To solve these issues, an efficient reconstructive homogeneous block-based local TRPCA is proposed for low-rank feature extraction, composed of a homogeneous block rebuilder and a local TRPCA low-rank feature extractor. The proposed local TRPCA is a novel data-model-driven algorithm depending on the data regulation. It remains the primary spatial and spectral contextual information and extracts the underlying homogeneity and heterogeneity characteristics of spatial, spectral, and spatial-spectral joint variables, which provides more essential features for further research than other model-driven TRPCA models. Furthermore, our local TRPCA feature extractor is an elaborate divide-and-rule model that executes on each homogeneous data block to extract low-rank features adaptively, remarkably decreasing computing cost and time. Experimental results on six hyperspectral datasets demonstrate that the proposed local TRPCA is more adaptive to HSIs and outperforms other state-of-the-art TRPCA-based feature extraction algorithms.
更多查看译文
关键词
Feature extraction,hybrid spatial-spectral frontal slice,hyperspectral image (HSI),reconstructive homogeneous block,tensor robust principal component analysis (TRPCA)
AI 理解论文
溯源树
样例
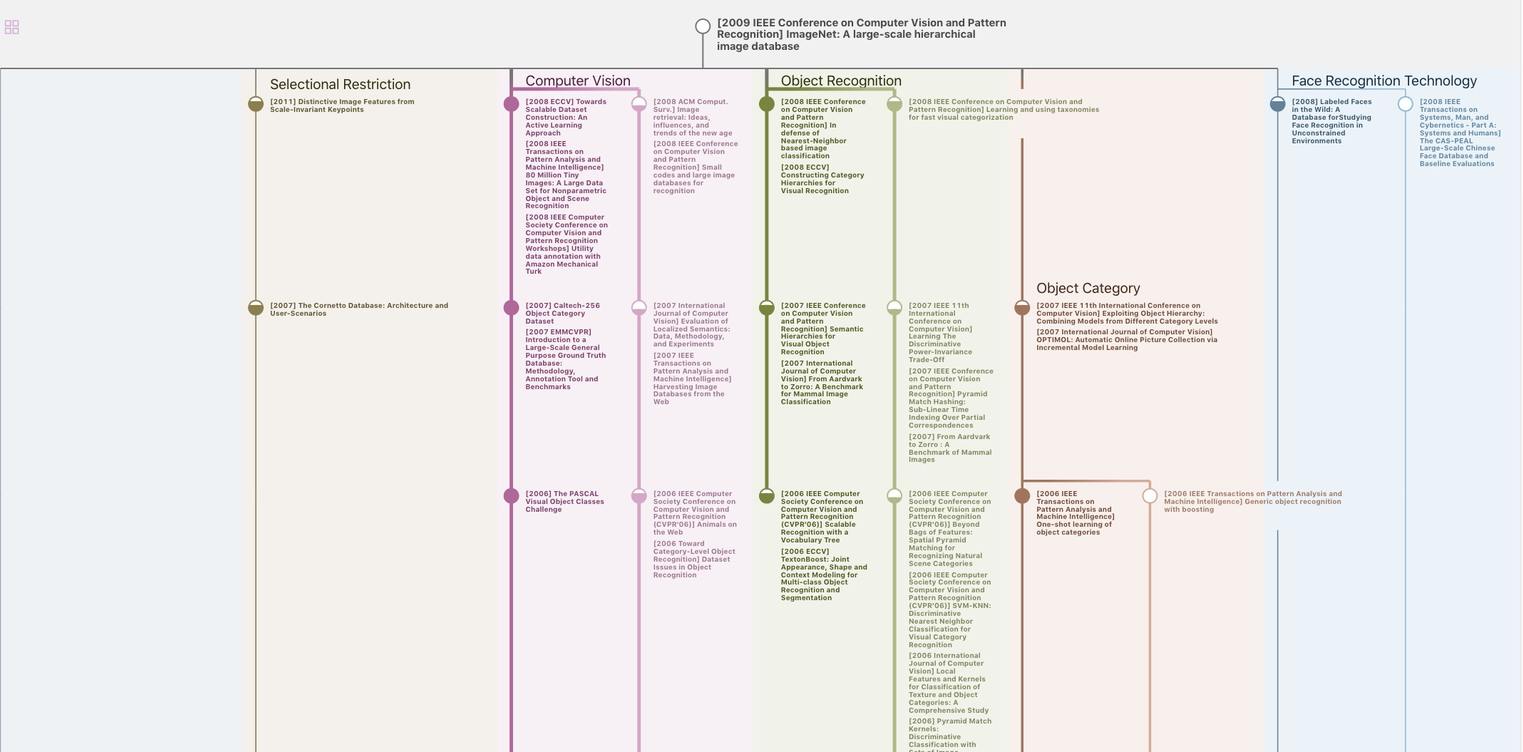
生成溯源树,研究论文发展脉络
Chat Paper
正在生成论文摘要