NeuralFloors: Conditional Street-Level Scene Generation from BEV Semantic Maps Via Neural Fields
IEEE Robotics and Automation Letters(2024)
关键词
Deep learning for visual perception,computer vision for transportation,neural rendering,cross-view transformation,data-driven simulation
AI 理解论文
溯源树
样例
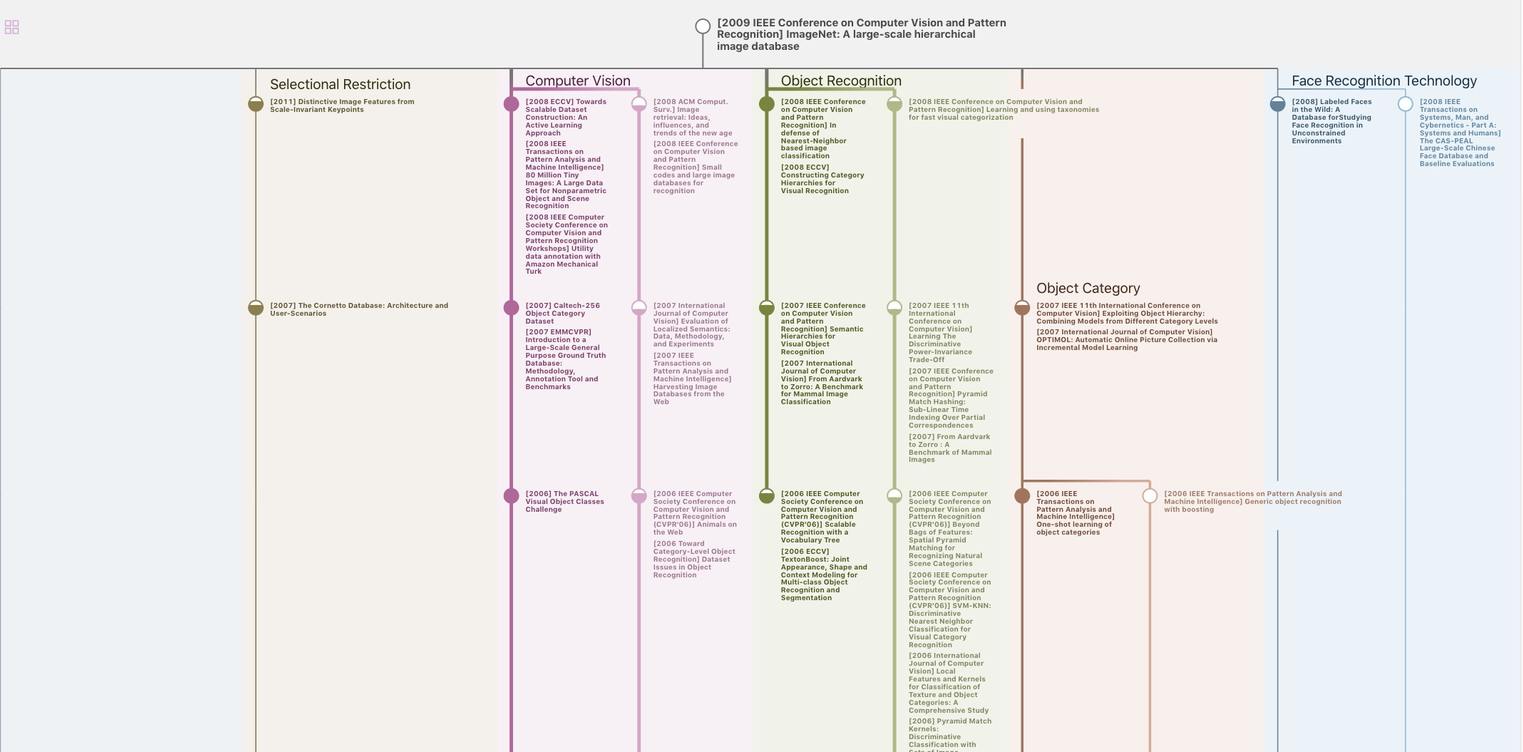
生成溯源树,研究论文发展脉络
Chat Paper
正在生成论文摘要