Fully Unsupervised Domain-Agnostic Image Retrieval
IEEE Transactions on Circuits and Systems for Video Technology(2023)
摘要
Recent research in cross-domain image retrieval has focused on addressing two challenging issues: handling domain variations in the data and dealing with the lack of sufficient training labels. However, these problems have often been studied separately, limiting the practicality and significance of the research outcomes. The existing cross-domain setting is also restricted to cases where domain labels are known during training, and all samples have semantic category information or instance correspondences. In this paper, we propose a novel approach to address a more general and practical problem:
fully unsupervised domain-agnostic image retrieval
under the domain-unknown setting, where no annotations are provided. Our approach tackles both the
domain variation
and
missing labels
challenges simultaneously. We introduce a new fully unsupervised One-Shot Synthesis-based Contrastive learning method (termed OSSCo) to project images from different data distributions into a shared feature space for similarity measurement. To handle the domain-unknown setting, we propose One-Shot unpaired image-to-image Translation (OST) between a randomly selected one-shot image and the rest of the training images. By minimizing the global distance between the original images and the generated images from OST, the model learns domain-agnostic representations. To address the label-unknown setting, we employ contrastive learning with a synthesis-based transform module from the OST training. This allows for effective representation learning without any annotations or external constraints. We evaluate our proposed method on diverse datasets, and the results demonstrate its effectiveness. Notably, our approach achieves comparable performance to current state-of-the-art supervised methods.
更多查看译文
关键词
One-shot image translation,Unsupervised learning,Image retrieval,Domain adaptation
AI 理解论文
溯源树
样例
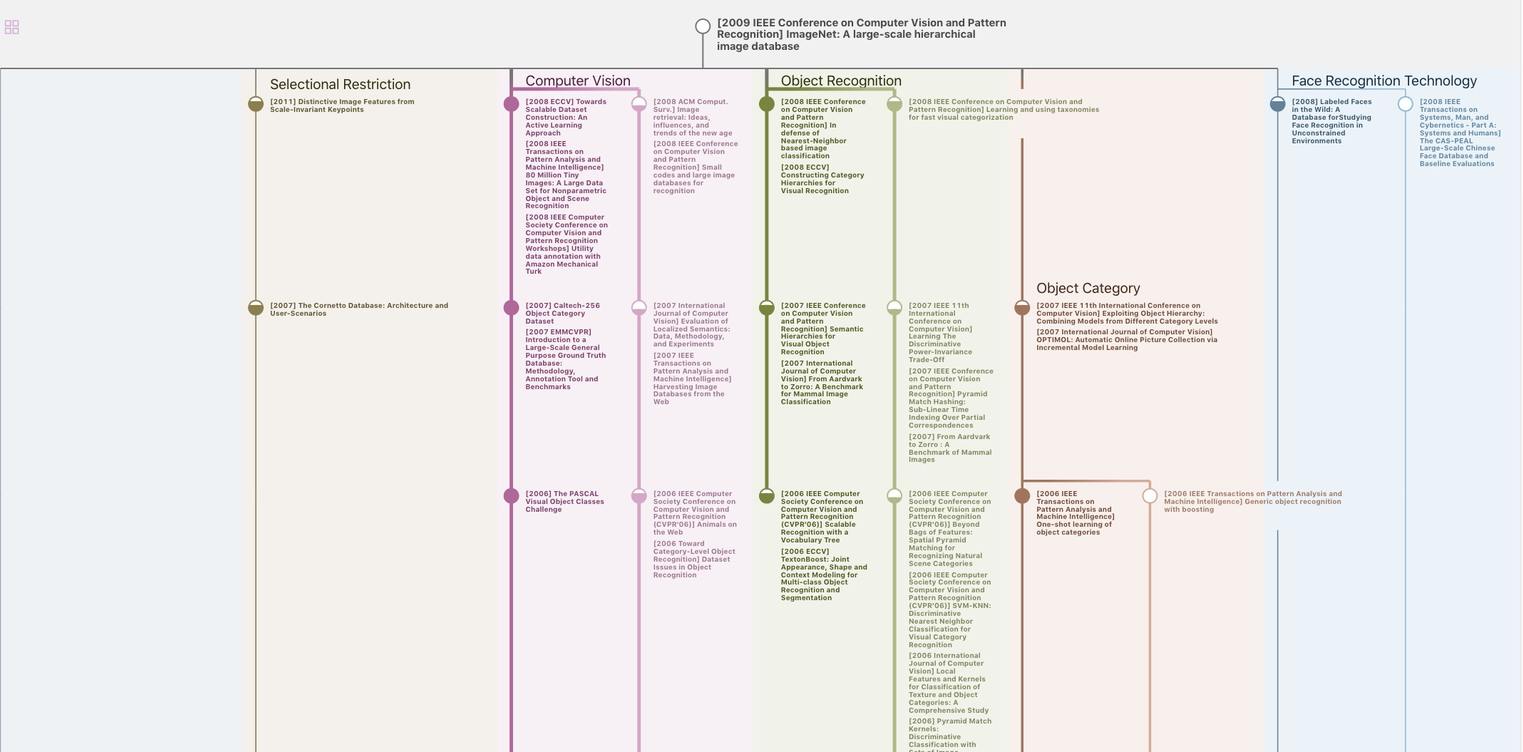
生成溯源树,研究论文发展脉络
Chat Paper
正在生成论文摘要