YOLO-HMC: an Improved Method for PCB Surface Defect Detection
IEEE Transactions on Instrumentation and Measurement(2024)
关键词
YOLO,Feature extraction,Inspection,Surface treatment,Deep learning,Adaptation models,Semantics,Deep learning (DL),defect defection,machine vision,printed circuit boards (PCBs),YOLOv5
AI 理解论文
溯源树
样例
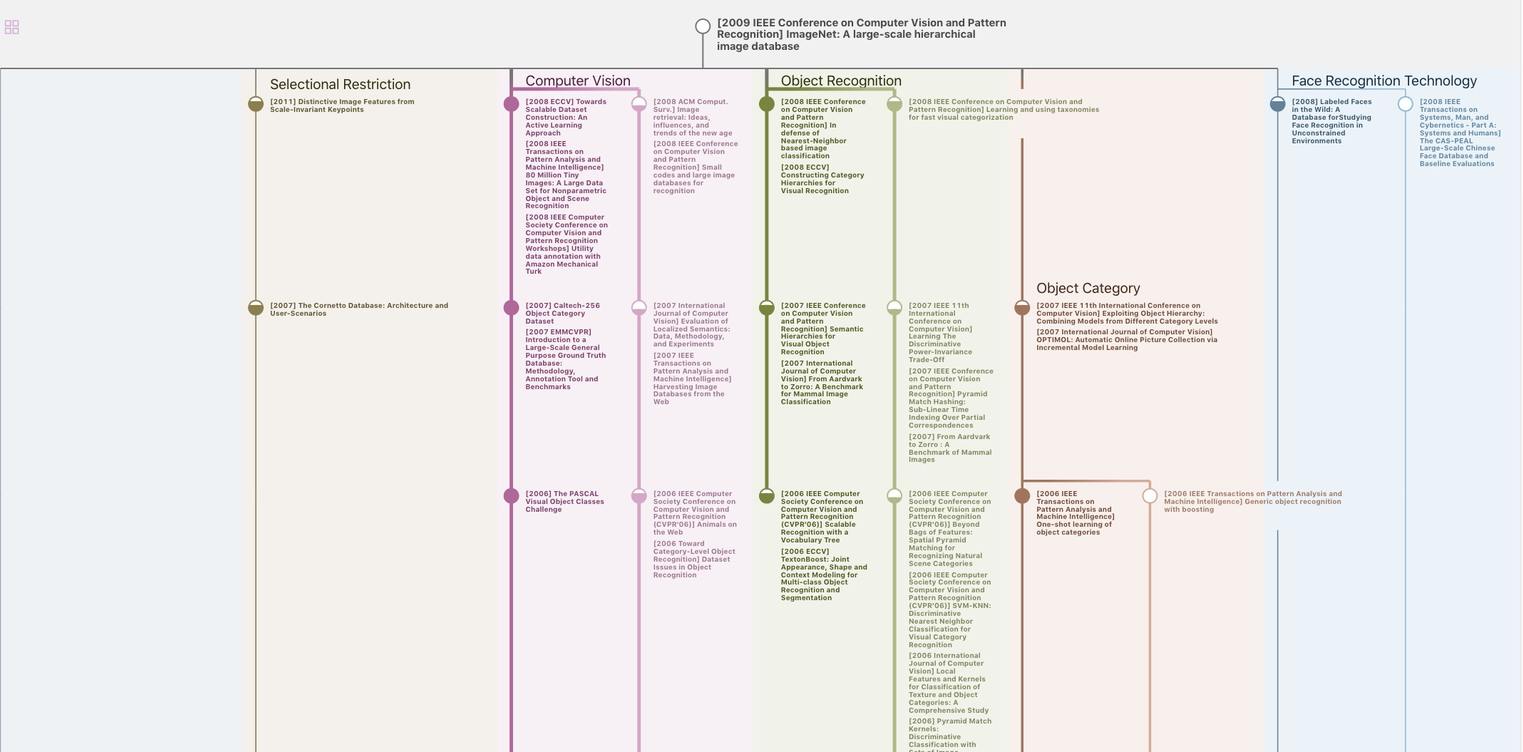
生成溯源树,研究论文发展脉络
Chat Paper
正在生成论文摘要