HAZARD Challenge: Embodied Decision Making in Dynamically Changing Environments
CoRR(2024)
摘要
Recent advances in high-fidelity virtual environments serve as one of the major driving forces for building intelligent embodied agents to perceive, reason and interact with the physical world. Typically, these environments remain unchanged unless agents interact with them. However, in real-world scenarios, agents might also face dynamically changing environments characterized by unexpected events and need to rapidly take action accordingly. To remedy this gap, we propose a new simulated embodied benchmark, called HAZARD, specifically designed to assess the decision-making abilities of embodied agents in dynamic situations. HAZARD consists of three unexpected disaster scenarios, including fire, flood, and wind, and specifically supports the utilization of large language models (LLMs) to assist common sense reasoning and decision-making. This benchmark enables us to evaluate autonomous agents' decision-making capabilities across various pipelines, including reinforcement learning (RL), rule-based, and search-based methods in dynamically changing environments. As a first step toward addressing this challenge using large language models, we further develop an LLM-based agent and perform an in-depth analysis of its promise and challenge of solving these challenging tasks. HAZARD is available at https://vis-www.cs.umass.edu/hazard/.
更多查看译文
关键词
embodied AI,decision making
AI 理解论文
溯源树
样例
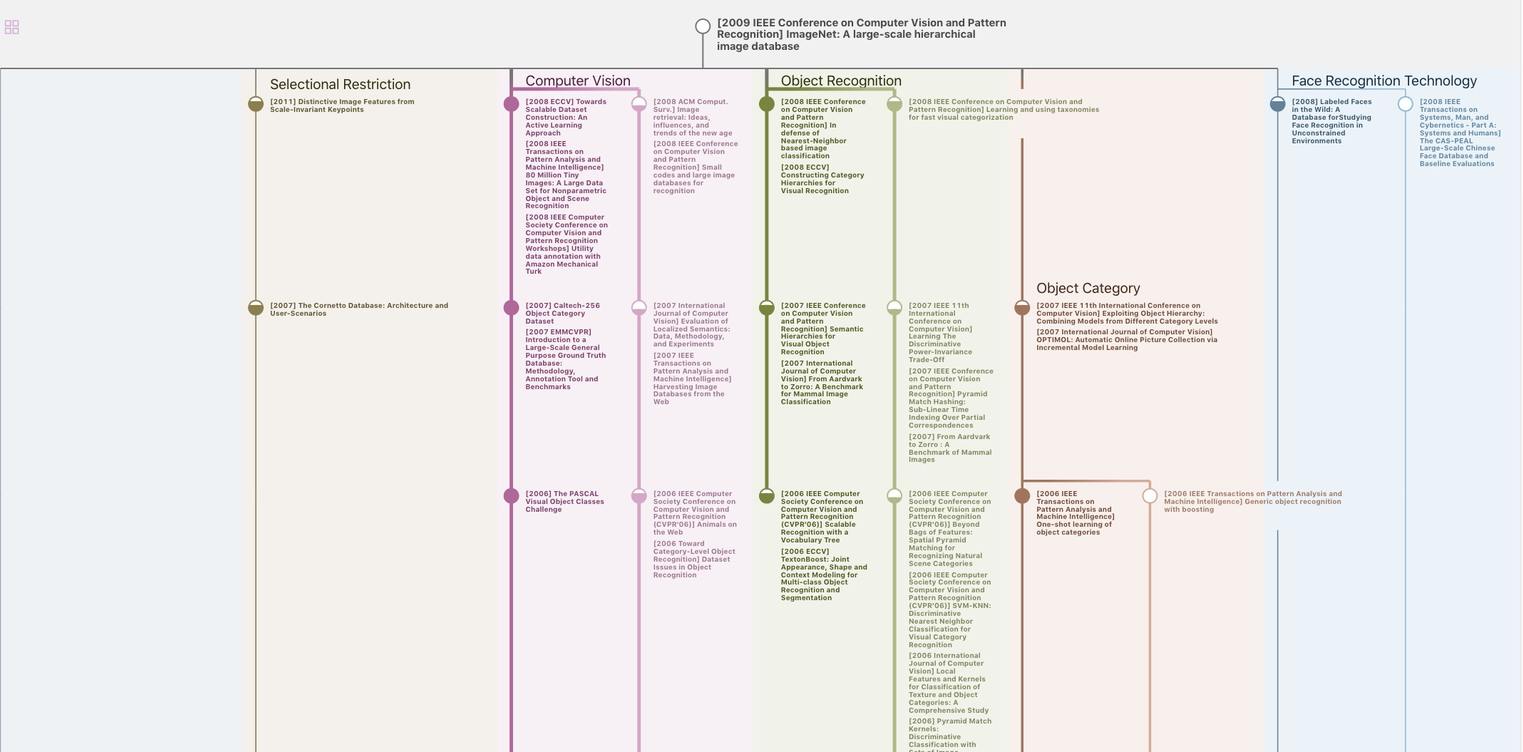
生成溯源树,研究论文发展脉络
Chat Paper
正在生成论文摘要