Monitoring the Epidemiology of Otitis Using Free-Text Pediatric Medical Notes: A Deep Learning Approach.
JOURNAL OF PERSONALIZED MEDICINE(2024)
Key words
otitis,machine learning,text mining,electronic medical record,real-world data
AI Read Science
Must-Reading Tree
Example
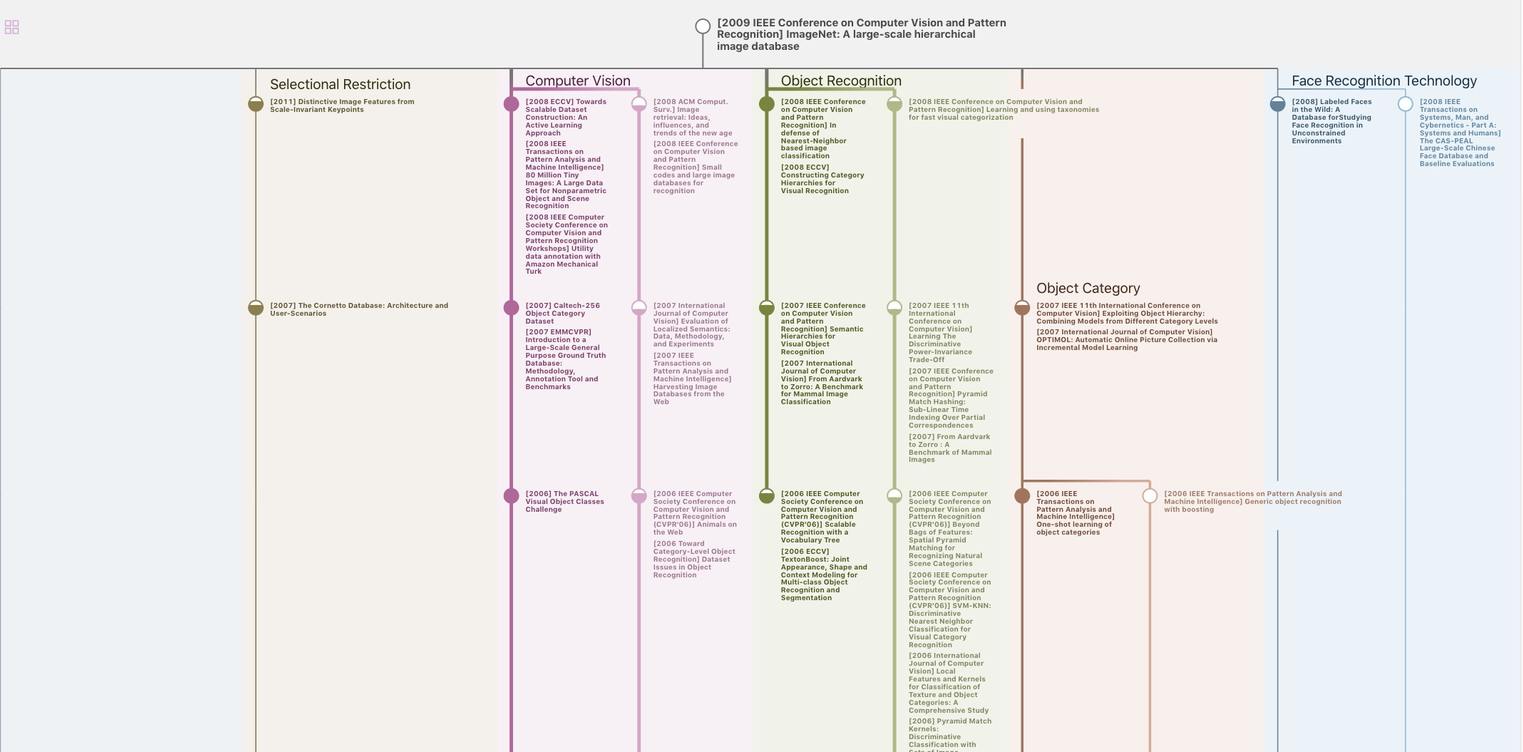
Generate MRT to find the research sequence of this paper
Chat Paper
Summary is being generated by the instructions you defined