Debiasing Counterfactual Context With Causal Inference for Multi-Turn Dialogue Reasoning
IEEE-ACM TRANSACTIONS ON AUDIO SPEECH AND LANGUAGE PROCESSING(2024)
摘要
In the multi-turn dialogue reasoning task, existing models conduct word-level interaction on the entire context to gather reasoning evidence, which aims to select the logically correct one from the candidate response options. Observing the fact that the salient reasoning evidence usually comes from certain snippets of the whole dialogue session, one promising study direction is to explicitly identify the candidate reasoning contexts correlated with the dialogue reasoning options, called option-related contexts, and then make logical inference among them. However, such option-related contexts are stained with noisy information. As a result, existing models may reason unfairly with biased context and select wrong options. To tackle the context bias problem, in this article, we propose a novel CounterFactual learning framework for Dialogue Reasoning, named CF-DialReas, which mitigates the bias information by subtracting the counterfactual representation from the total causal representation. Specifically, we consider two scenarios, i.e., factual dialogue reasoning where the whole context is available to estimate the total causal representation, and the counterfactual dialogue reasoning, which firstly utilizes three different types of utterance selectors to select option-unrelated context, and then only the option-unrelated context is available to guess the counterfactual representation. Experimental results on two public dialogue reasoning datasets show that the model with our mechanism can obtain higher ranking measures, validating the effectiveness of counterfactual learning of CF-DialReas. Further analysis on the generality of CF-DialReas shows that our counterfactual learning mechanism is generally effective to the widely-used models.
更多查看译文
关键词
Dialogue reasoning,context bias,CounterFactual
AI 理解论文
溯源树
样例
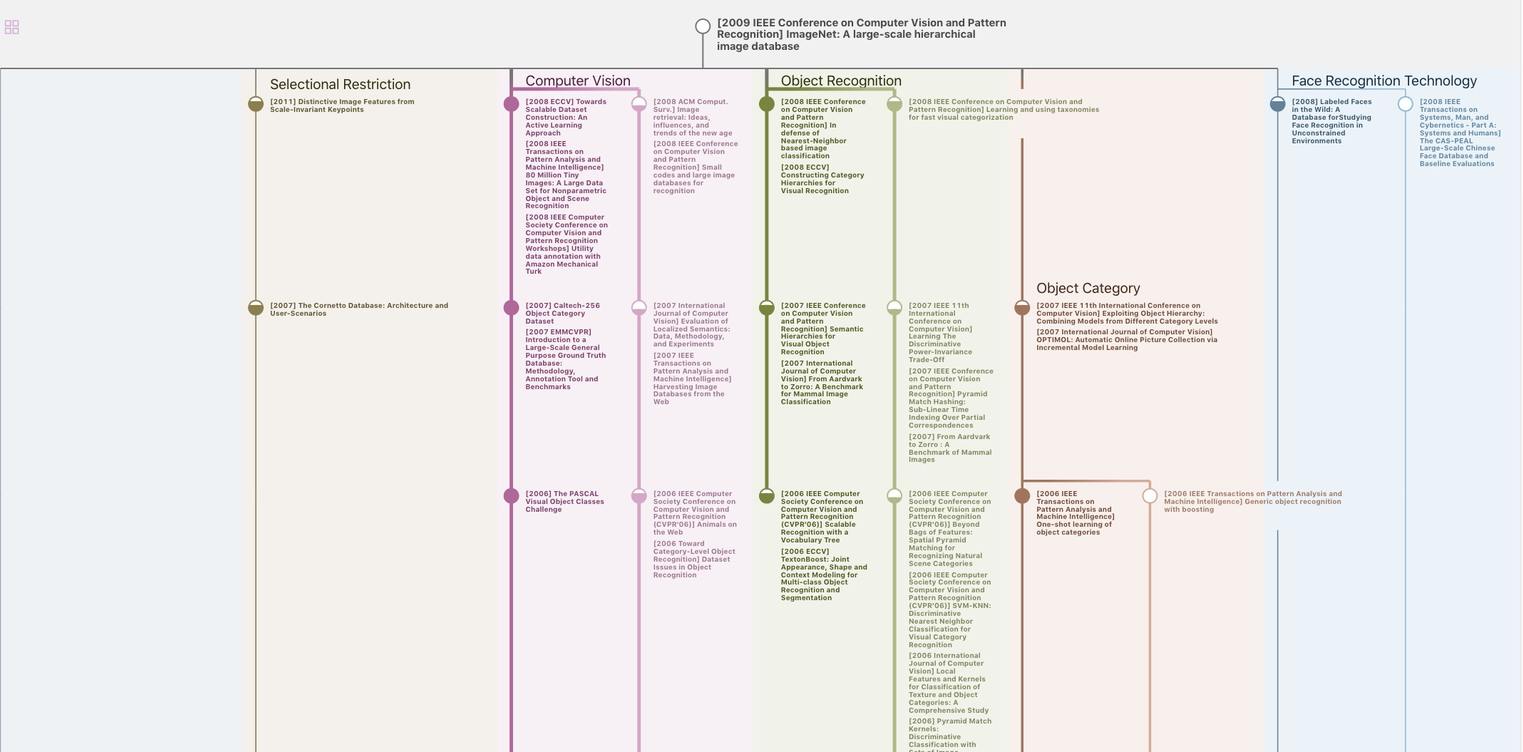
生成溯源树,研究论文发展脉络
Chat Paper
正在生成论文摘要