Interpretable classifier design by axiomatic fuzzy sets theory and derivative-free optimization
EXPERT SYSTEMS WITH APPLICATIONS(2024)
摘要
Axiomatic fuzzy sets (AFS) theory has seen extensive application in classification tasks in recent years. Yet, efficiently generating precise fuzzy descriptions for each target class from a multitude of complex concepts is challenging. This study aims to bridge this gap by transforming the challenge into a combinatorial optimization problem. We adopt an advanced model -based evolutionary optimization method, Randomized Coordinate Shrinking Classification (RACOS), to create an interpretable classifier within the AFS framework. By refining the definition of the complex concept set in AFS theory, we define a feasible search space for the optimization method. Innovative fitness functions have been developed focusing on semantic discrimination and prediction accuracy. Concurrently, we establish an encoding-decoding mechanism to link the solution vector with the complex concept for each fitness function. Ultimately, the complex concepts, guided by various fitness functions, are integrated into the class's fuzzy description using AFS logical operations. Our method demonstrates competitive classification performance and superior interpretability compared to other evolutionary fuzzy rule -based classifiers.
更多查看译文
关键词
Axiomatic fuzzy sets,Derivative-free optimization,Semantic interpretability
AI 理解论文
溯源树
样例
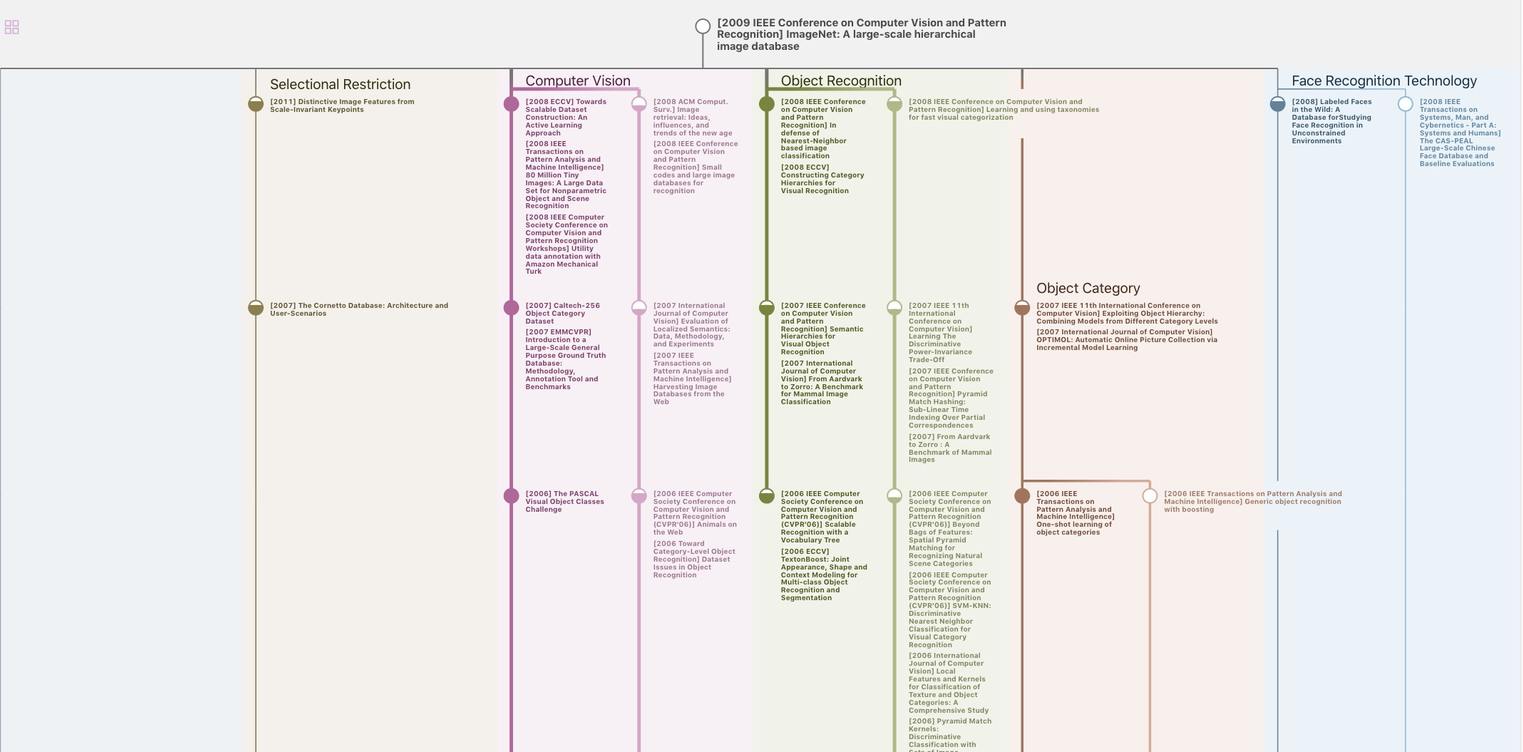
生成溯源树,研究论文发展脉络
Chat Paper
正在生成论文摘要