Recognizing Hand Movements Using EEG-Signal Classification
2023 Eleventh International Conference on Intelligent Computing and Information Systems (ICICIS)(2023)
摘要
Classifying various precise manual movements based on electroencephalogram (EEG) signals presents an important research obstacle, particularly in the category of motor rehabilitation within brain-computer interface (BCI) applications. This study focuses on classifying EEG signals into six hand movement classes (forward, backward, up, down, right, left) utilizing machine learning and deep learning methods. The goal is to detect the person's current thoughts and intentions, including motor imagery and real movements, to assist individuals with physical disabilities or limb loss in controlling their robotic arms. After data collection and preprocessing, feature extraction is performed on the EEG signals obtained from the five signal bands (alpha, beta, theta, delta, gamma). Deep learning techniques include the use of recurrent neural networks with long short-term memory (RNN-LSTM) and convolutional neural networks (CNN), in addition to more traditional classifiers like Support Vector Machines (SVM) and Linear Discriminant Analysis (LDA), an overall best accuracy 70% is achieved in detecting the 6 hand movements classes from one subject. DWT with Logistic Regression, also CSP using SVM (one vs rest) achieved the same accuracy. EEG-Net also got 70% accuracy on the 6 classes. 93% accuracy is achieved for binary classification (2 classes) with CSP as a feature and Random Forest classifier also DWT using LSTM achieved the same accuracy using a 60-channel EEG system, the results that overcome the results from literature review on the same dataset. Cross subjects' technique is experimented also in this study. Using cross subjects' data from 4 classes, CSP using LSTM shows high accuracy of 61%. The use of a 60-channel EEG system and the adoption of deep learning techniques resulted in the best accuracy for hand movement recognition in this study.
更多查看译文
关键词
EEG signal classification,Hand movement,signals,BCI system- Multi-class Classification
AI 理解论文
溯源树
样例
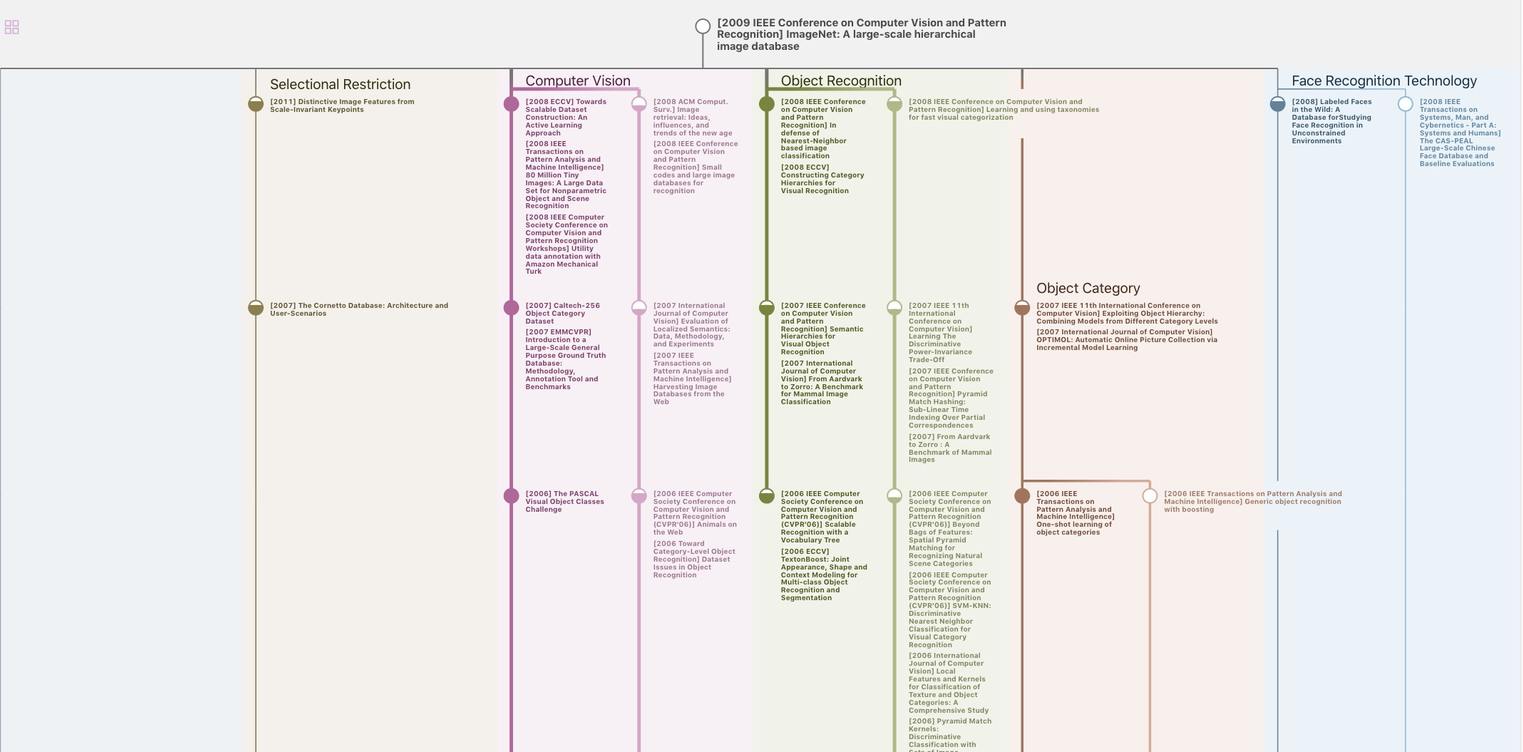
生成溯源树,研究论文发展脉络
Chat Paper
正在生成论文摘要