Detecting Semantic Similarity of Diseases Based Machine Learning
2023 IEEE International Conference on Bioinformatics and Biomedicine (BIBM)(2023)
Key words
disease-disease associations,machine learning,Grouping-Scoring-Modeling approach,semantic similarity,Jaccard similarity,GediNETPro,Monte Carlo cross-validation,K-means clustering algorithm,diversity metrics,Jaccard index
AI Read Science
Must-Reading Tree
Example
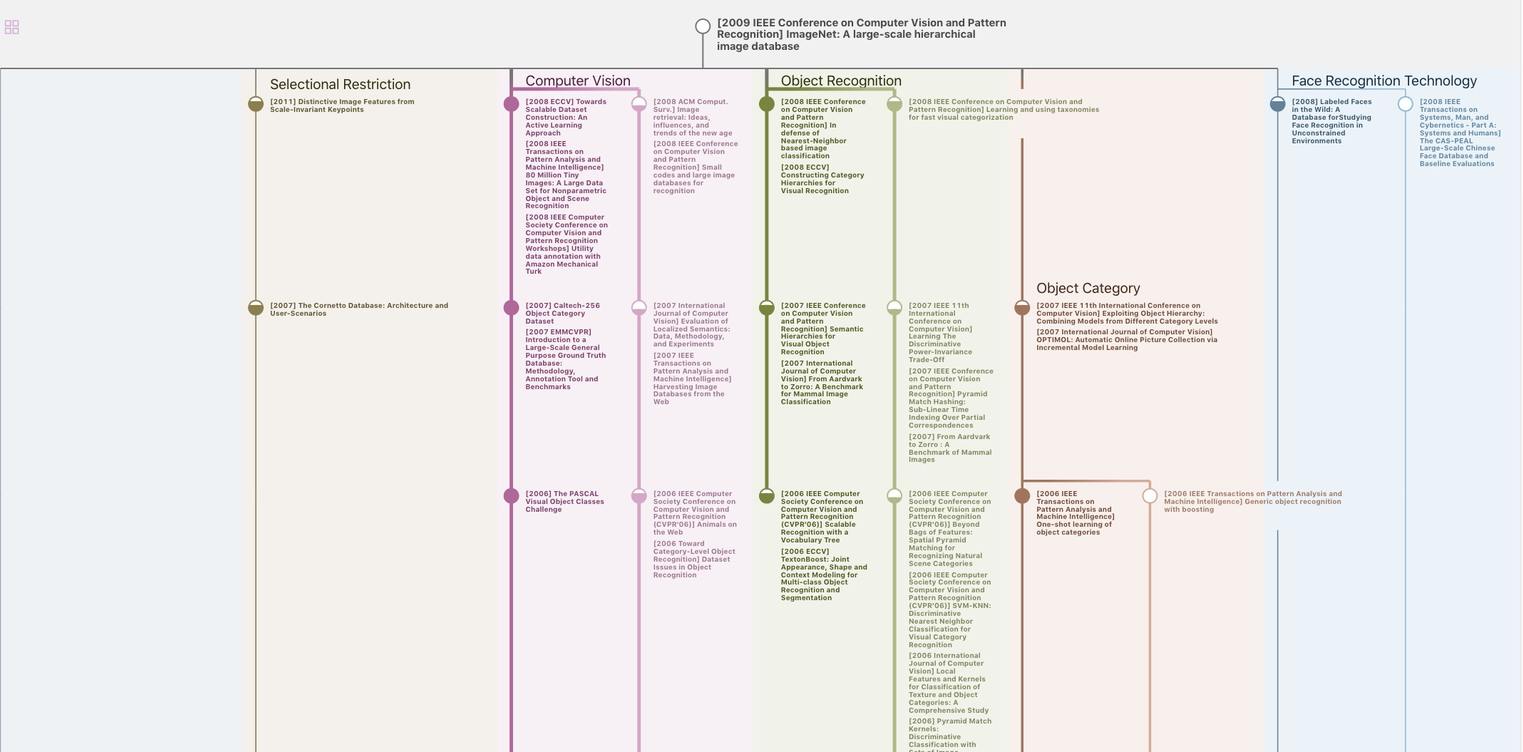
Generate MRT to find the research sequence of this paper
Chat Paper
Summary is being generated by the instructions you defined