Integrating Summary Information from Many External Studies with Population Heterogeneity and a Study of COVID-19 Pandemic Impact on Mental Health of People with Bipolar Disorder
Statistics in Biosciences(2024)
摘要
The penalized constrained maximum likelihood (PCML) method integrates summary information from external studies to improve model parameter estimation for an index study of interest in the context of population heterogeneity. It simultaneously selects and incorporates relevant external information. However, it only considers scenarios where the number of external studies is small. There are situations where many external studies exist for possible information integration. Here, we extend the PCML method by allowing the number of external studies to increase according to the sample size of the index (internal) study, motivated by a study of the COVID-19 pandemic impact on mental health of people with bipolar disorder. Our extension allows leveraging the many relevant external studies of mental health before and during the COVID-19 pandemic. Within our general framework, the asymptotic properties of the PCML estimator, including external information selection consistency and oracle efficiency, are established. We also carry out comprehensive simulation studies under varying numbers of external studies. The method is then applied to the bipolar-COVID study to integrate useful external information from the many existing mental health studies. Integrating external information helps to reveal mental health outcome trends from pre-pandemic to pandemic periods.
更多查看译文
关键词
Constrained maximum likelihood,Data integration,Empirical likelihood,Shrinkage,Summary information
AI 理解论文
溯源树
样例
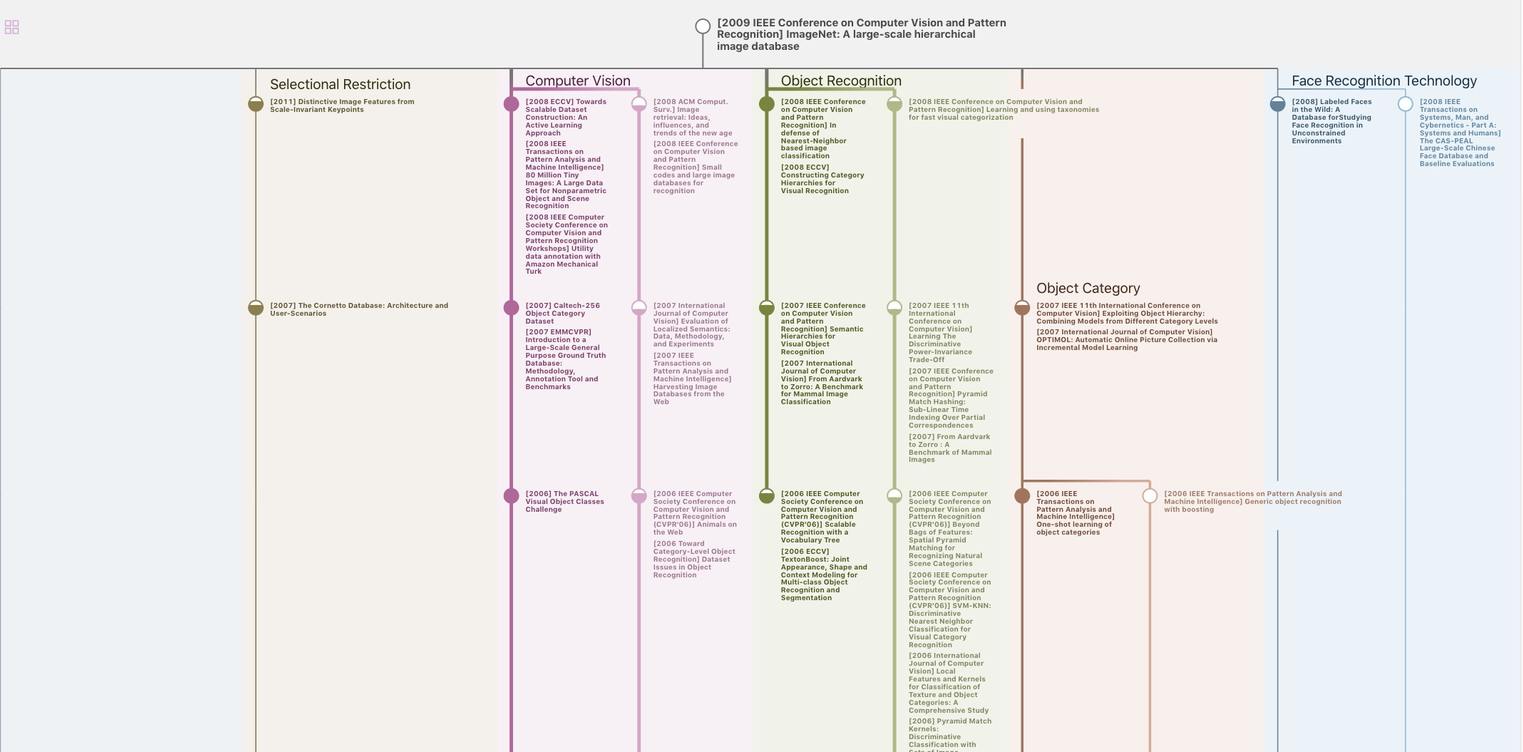
生成溯源树,研究论文发展脉络
Chat Paper
正在生成论文摘要