Optimal experimental design via gradient flow
CoRR(2024)
摘要
Optimal experimental design (OED) has far-reaching impacts in many scientific
domains. We study OED over a continuous-valued design space, a setting that
occurs often in practice. Optimization of a distributional function over an
infinite-dimensional probability measure space is conceptually distinct from
the discrete OED tasks that are conventionally tackled. We propose techniques
based on optimal transport and Wasserstein gradient flow. A practical
computational approach is derived from the Monte Carlo simulation, which
transforms the infinite-dimensional optimization problem to a
finite-dimensional problem over Euclidean space, to which gradient descent can
be applied. We discuss first-order criticality and study the convexity
properties of the OED objective. We apply our algorithm to the tomography
inverse problem, where the solution reveals optimal sensor placements for
imaging.
更多查看译文
AI 理解论文
溯源树
样例
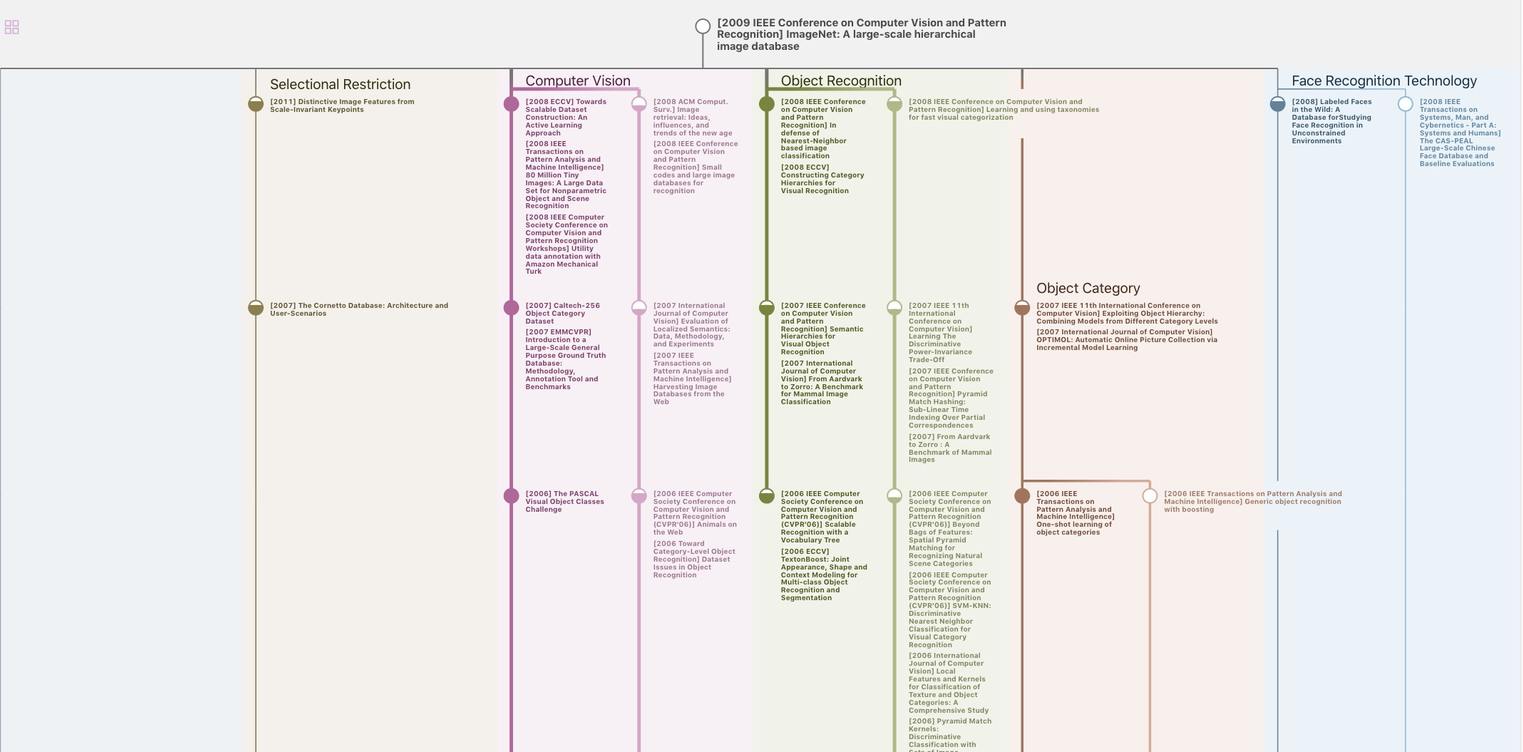
生成溯源树,研究论文发展脉络
Chat Paper
正在生成论文摘要