PathFinder: a novel graph transformer model to infer multi-cell intra- and inter-cellular signaling pathways and communications.
bioRxiv : the preprint server for biology(2024)
摘要
Recently, large-scale scRNA-seq datasets have been generated to understand the complex and poorly understood signaling mechanisms within microenvironment of Alzheimer's Disease (AD), which are critical for identifying novel therapeutic targets and precision medicine. Though a set of targets have been identified, however, it remains a challenging to infer the core intra- and inter-multi-cell signaling communication networks using the scRNA-seq data, considering the complex and highly interactive background signaling network. Herein, we introduced a novel graph transformer model, PathFinder, to infer multi-cell intra- and inter-cellular signaling pathways and signaling communications among multi-cell types. Compared with existing models, the novel and unique design of PathFinder is based on the divide-and-conquer strategy, which divides the complex signaling networks into signaling paths, and then score and rank them using a novel graph transformer architecture to infer the intra- and inter-cell signaling communications. We evaluated PathFinder using scRNA-seq data of APOE4-genotype specific AD mice models and identified novel APOE4 altered intra- and inter-cell interaction networks among neurons, astrocytes, and microglia. PathFinder is a general signaling network inference model and can be applied to other omics data-driven signaling network inference.
更多查看译文
AI 理解论文
溯源树
样例
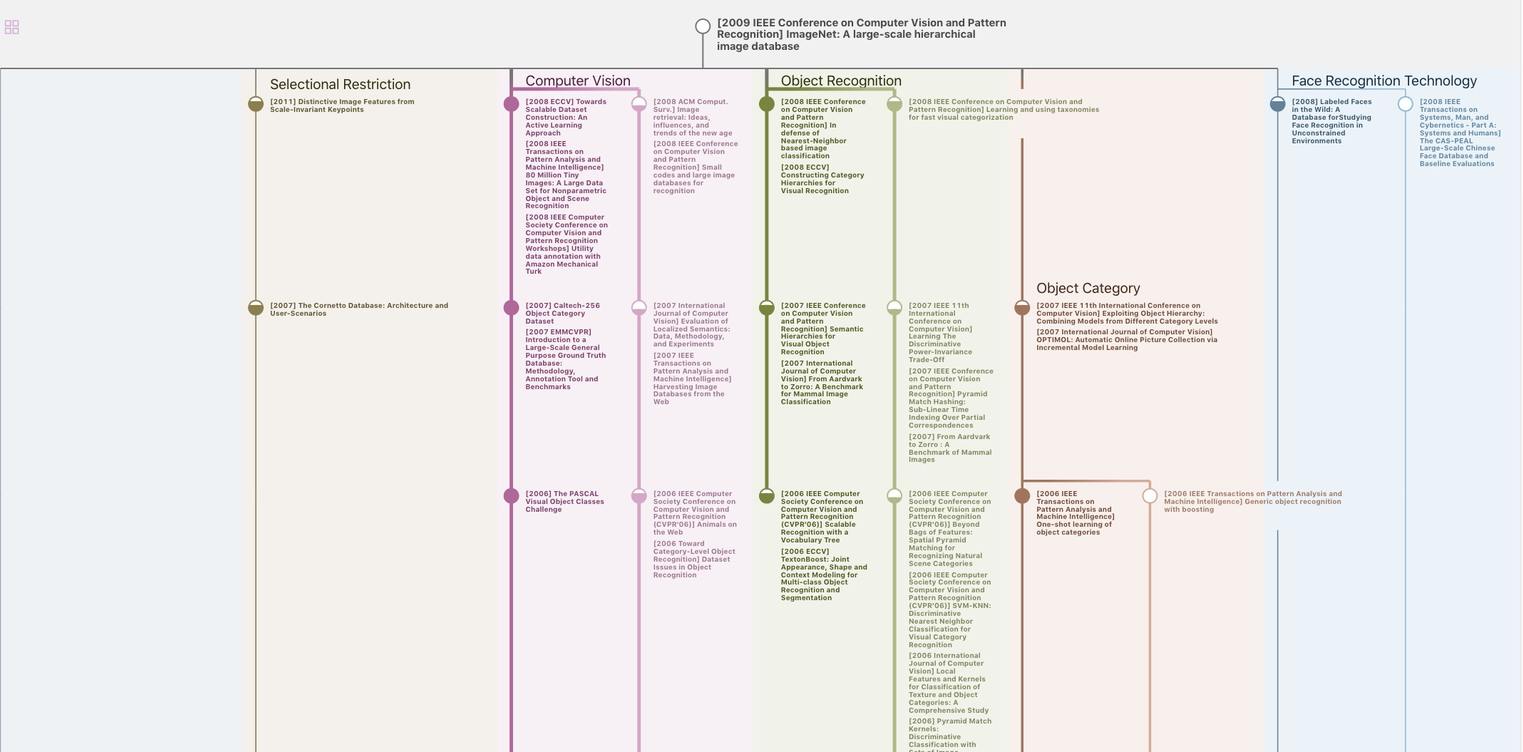
生成溯源树,研究论文发展脉络
Chat Paper
正在生成论文摘要