Android Malware Family Clustering Based on Multiple Features
IEEE TRANSACTIONS ON RELIABILITY(2023)
摘要
Familiar analysis for malware plays an important role in comprehending the diversity of malicious behaviors and identifying the emerging security threats. Existing studies mainly focus on classifying malware into known families by supervised learning. However, these methods face two main challenges, 1) the lack of a large amount of labeled data and 2) the poor effectiveness in identifying unknown families of malware. To overcome these challenges, we propose a new method called multiple features (MulFC) based on unsupervised learning. In the method, we first leverage a decompiling tool to extract multiple features, including manifest features, application programming interface (API) features, and opcode features. Then, the opcode features are preprocessed to filter out the redundant ones to reduce the calculation cost. After that, we adopt the Jaccard index to calculate the similarities between malware and construct a malware network. Finally, InfoMap is applied to perform the clustering on the basis of the malware network. Overall, MulFC does not require the use of labeled data and can identify unknown families of malware. Experiments are conducted on two datasets for the performance evaluation of MulFC. The experimental results show that MulFC achieves 0.810 in terms of normalized mutual information, 0.576 in terms of adjusted rand index, 0.620 in terms of the Fowlkes-Mallows index, and 0.805 in terms of V-measure on average, and outperforms the state-of-the-art baseline method by 0.060, 0.054, 0.038, and 0.065, respectively.
更多查看译文
关键词
Android malware,InfoMap,malware family clustering,multiple features,unsupervised learning
AI 理解论文
溯源树
样例
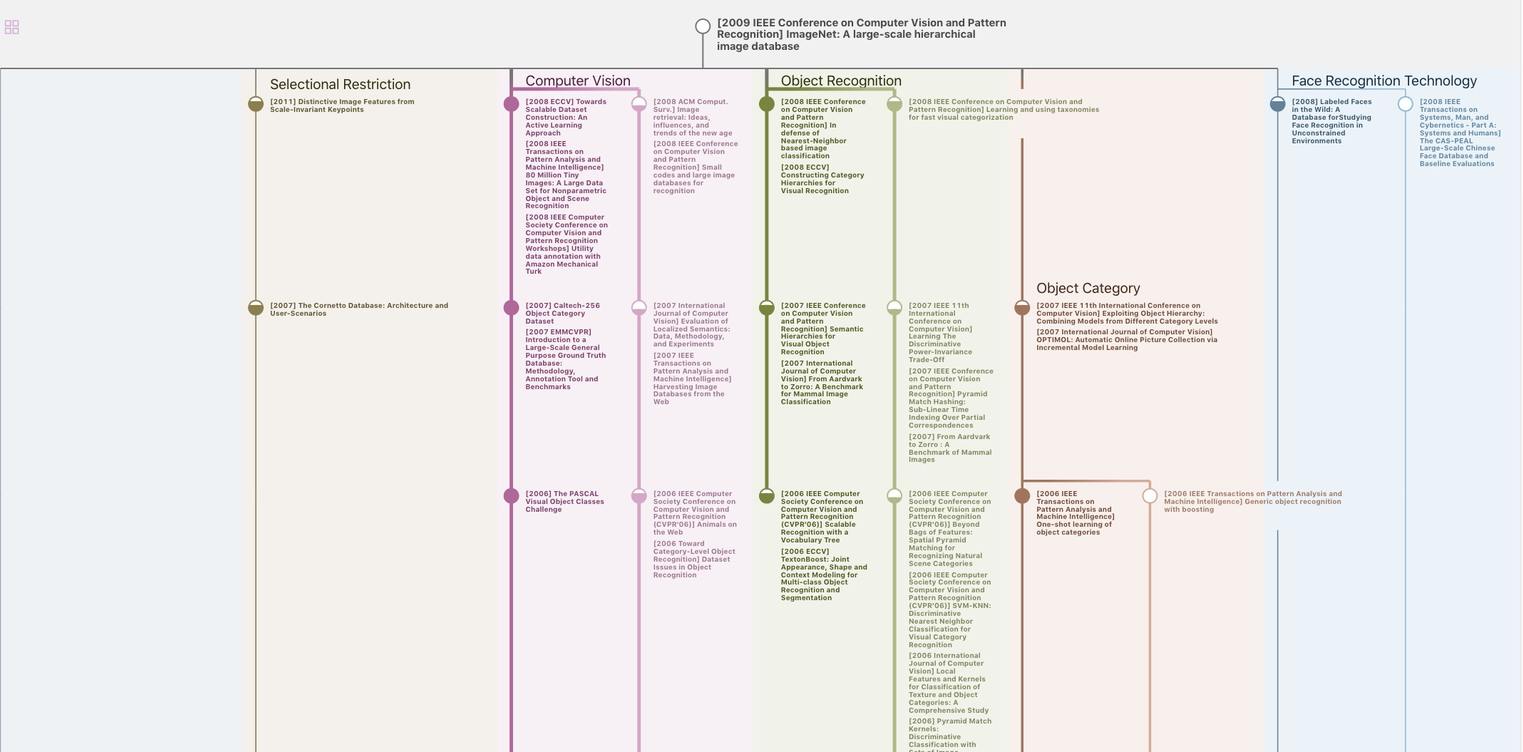
生成溯源树,研究论文发展脉络
Chat Paper
正在生成论文摘要