Parkinson's severity diagnosis explainable model based on 3D multi-head attention residual network
COMPUTERS IN BIOLOGY AND MEDICINE(2024)
摘要
The severity evaluation of Parkinson's disease (PD) is of great significance for the treatment of PD. However, existing methods either have limitations based on prior knowledge or are invasive methods. To propose a more generalized severity evaluation model, this paper proposes an explainable 3D multi -head attention residual convolution network. First, we introduce the 3D attention -based convolution layer to extract video features. Second, features will be fed into LSTM and residual backbone networks, which can be used to capture the contextual information of the video. Finally, we design a feature compression module to condense the learned contextual features. We develop some interpretable experiments to better explain this black -box model so that it can be better generalized. Experiments show that our model can achieve state-of-the-art diagnosis performance. The proposed lightweight but effective model is expected to serve as a suitable end -to -end deep learning baseline in future research on PD video -based severity evaluation and has the potential for large-scale application in PD telemedicine. The source code is available at https://github.com/JackAILab/MARNet.
更多查看译文
关键词
Parkinson's disease diagnosis,Explainable deep learning,Severity diagnosis,Telemedicine,Multi-head attention
AI 理解论文
溯源树
样例
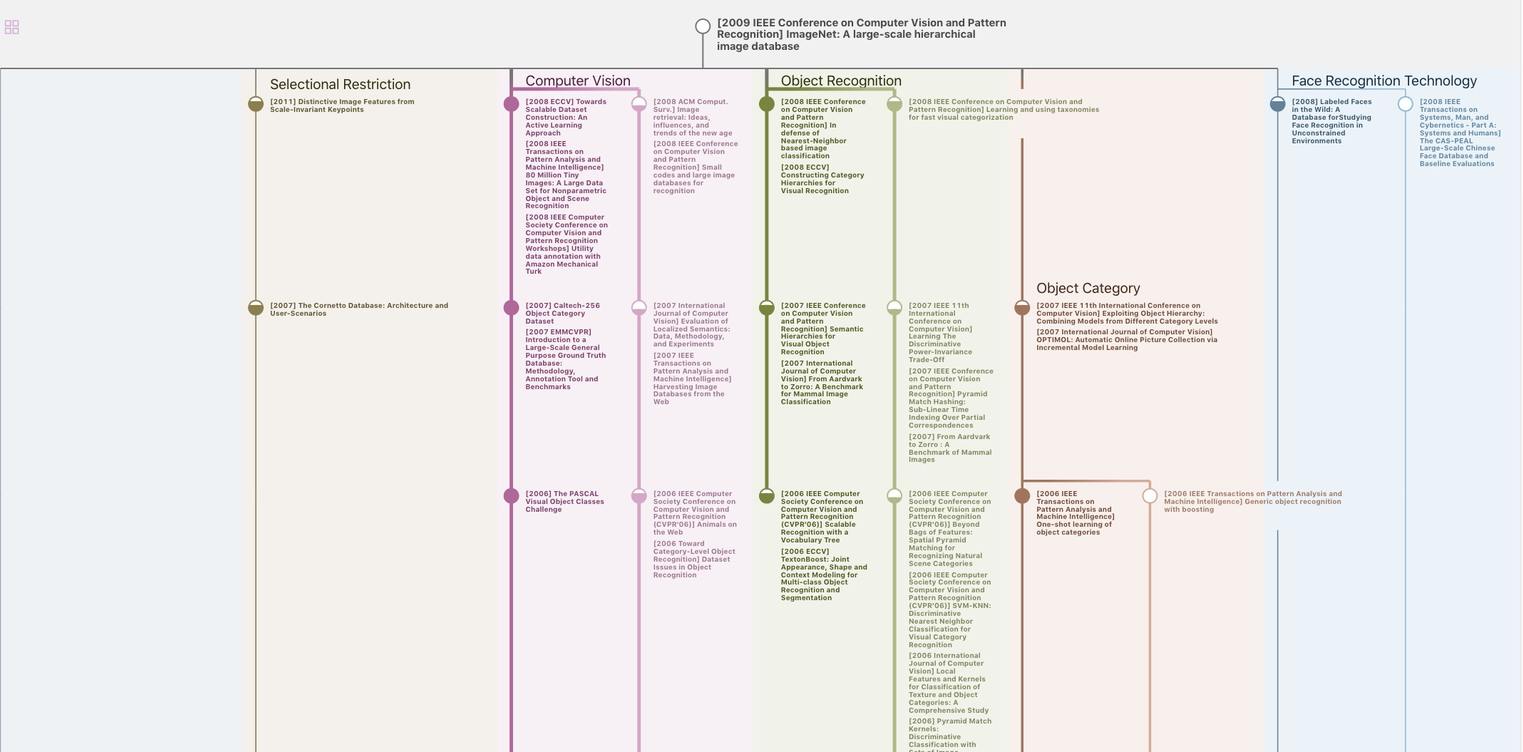
生成溯源树,研究论文发展脉络
Chat Paper
正在生成论文摘要