EI-MVSNet: Epipolar-Guided Multi-View Stereo Network With Interval-Aware Label
IEEE TRANSACTIONS ON IMAGE PROCESSING(2024)
摘要
Recent learning-based methods demonstrate their strong ability to estimate depth for multi-view stereo reconstruction. However, most of these methods directly extract features via regular or deformable convolutions, and few works consider the alignment of the receptive fields between views while constructing the cost volume. Through analyzing the constraint and inference of previous MVS networks, we find that there are still some shortcomings that hinder the performance. To deal with the above issues, we propose an Epipolar-Guided Multi-View Stereo Network with Interval-Aware Label (EI-MVSNet), which includes an epipolar-guided volume construction module and an interval-aware depth estimation module in a unified architecture for MVS. The proposed EI-MVSNet enjoys several merits. First, in the epipolar-guided volume construction module, we construct cost volume with features from aligned receptive fields between different pairs of reference and source images via epipolar-guided convolutions, which take rotation and scale changes into account. Second, in the interval-aware depth estimation module, we attempt to supervise the cost volume directly and make depth estimation independent of extraneous values by perceiving the upper and lower boundaries, which can achieve fine-grained predictions and enhance the reasoning ability of the network. Extensive experimental results on two standard benchmarks demonstrate that our EI-MVSNet performs favorably against state-of-the-art MVS methods. Specifically, our EI-MVSNet ranks $1_{st}$ on both intermediate and advanced subsets of the Tanks and Temples benchmark, which verifies the high precision and strong robustness of our model.
更多查看译文
关键词
Costs,Estimation,Feature extraction,Three-dimensional displays,Learning systems,Image reconstruction,Aggregates,Multi-view stereo,alignment,epipolar,interval-aware
AI 理解论文
溯源树
样例
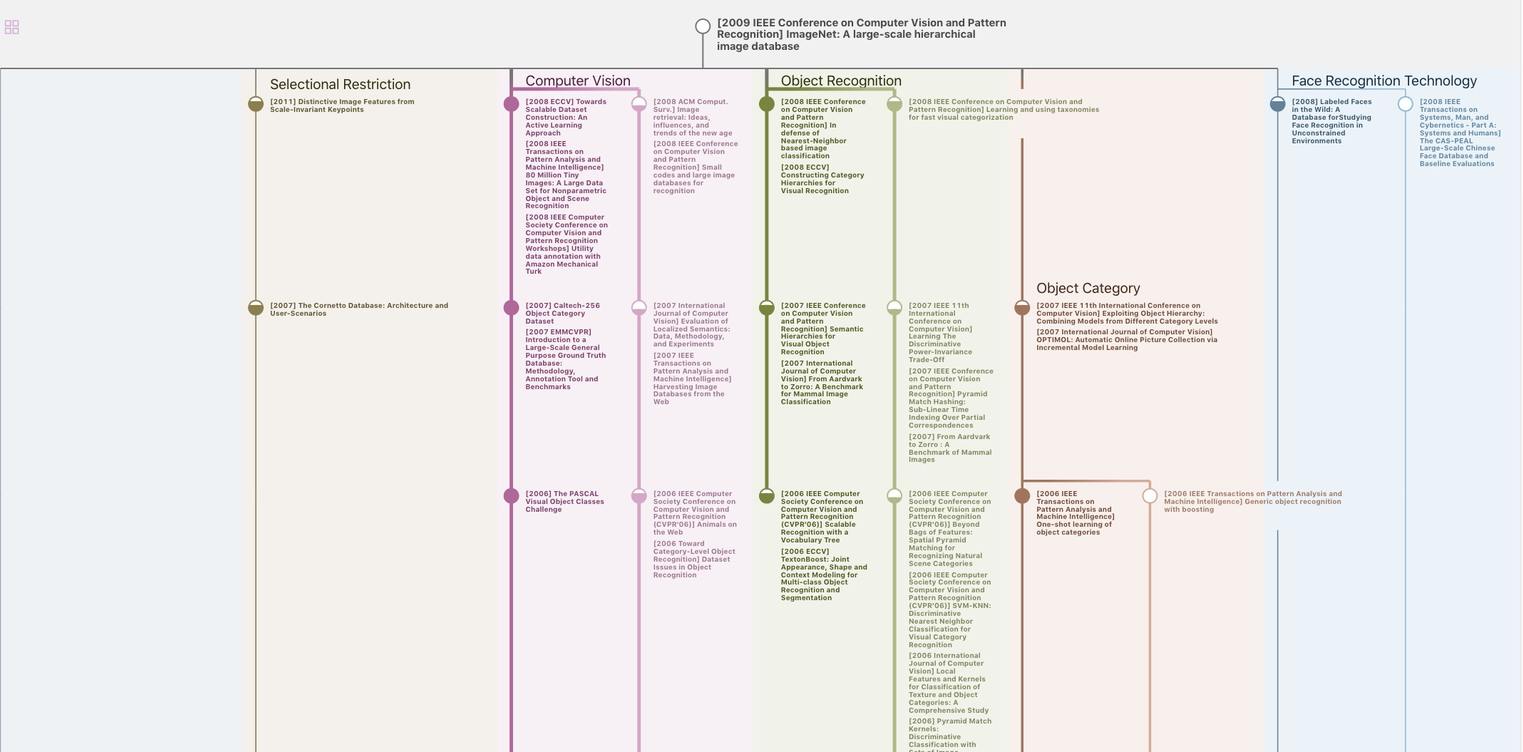
生成溯源树,研究论文发展脉络
Chat Paper
正在生成论文摘要