IGMG: Instance-guided multi-granularity for domain generalizable person re-identification
COMPUTER VISION AND IMAGE UNDERSTANDING(2024)
摘要
Learning generalized feature embedding is crucial for various computer vision tasks, including domain generalizable person re -identification (ReID). ReID aims to develop deep learning -based feature embeddings that can effectively recognize individuals in both trained (source) domains and unseen target domains. However, many state-of-the-art ReID methods suffer from overfitting as they train and test within the same source domain. To address this issue, we investigate the potential of multi -granularity approaches in mitigating domain shift challenges in person re -identification. Specifically, we propose a novel framework called instance -guided multigranularity (IGMG), which leverages style -free features through non -parametric Instance Normalization (IN) at multiple granularity levels. While high-level abstract concepts are often not shared across different classes, lowand mid -level features can offer more shareable information to enhance the model's generalization capabilities. By incorporating this concept, our framework can dynamically eliminate style variations across various levels of abstraction. As a result, it enables the model to capture fine-grained details and high-level semantics, leading to enhanced robustness against changes in data distribution. To validate the effectiveness of our approach, we conduct extensive experiments on multiple benchmark ReID datasets. The results consistently demonstrate that our proposed framework exhibits strong generalization capabilities, performing consistently well on unseen target domains. The code is available at https://github.com/mdamranhossenbhuiyan/IGMG/.
更多查看译文
关键词
Re-identification,Domain Generalization,Normalization,multi-granularity,Style-discrepancy
AI 理解论文
溯源树
样例
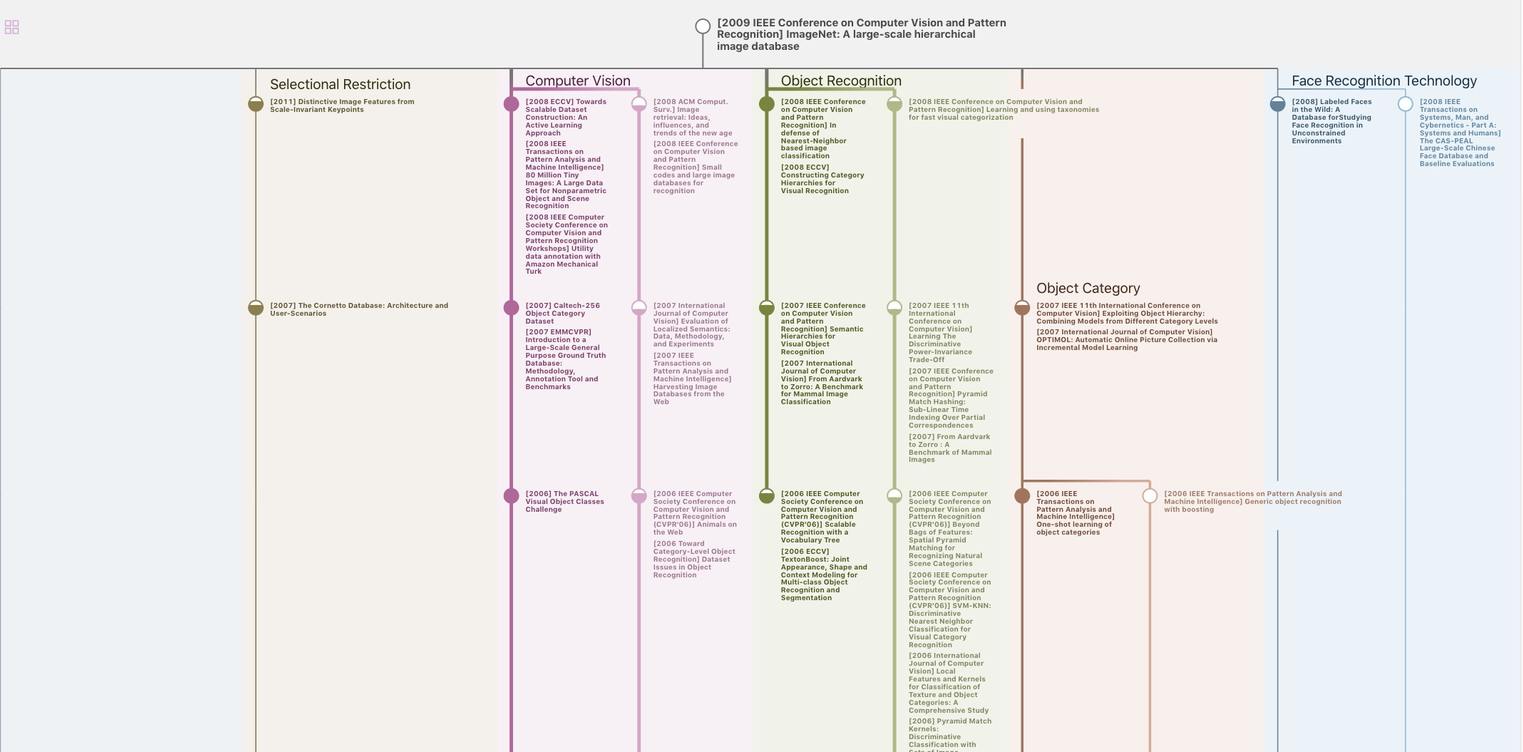
生成溯源树,研究论文发展脉络
Chat Paper
正在生成论文摘要