Spectral Knowledge Transfer for Remote Sensing Change Detection
IEEE TRANSACTIONS ON GEOSCIENCE AND REMOTE SENSING(2024)
Key words
Change detection (CD),data augmentation,deep learning,very-high-resolution (VHR) remote sensing (RS) images
AI Read Science
Must-Reading Tree
Example
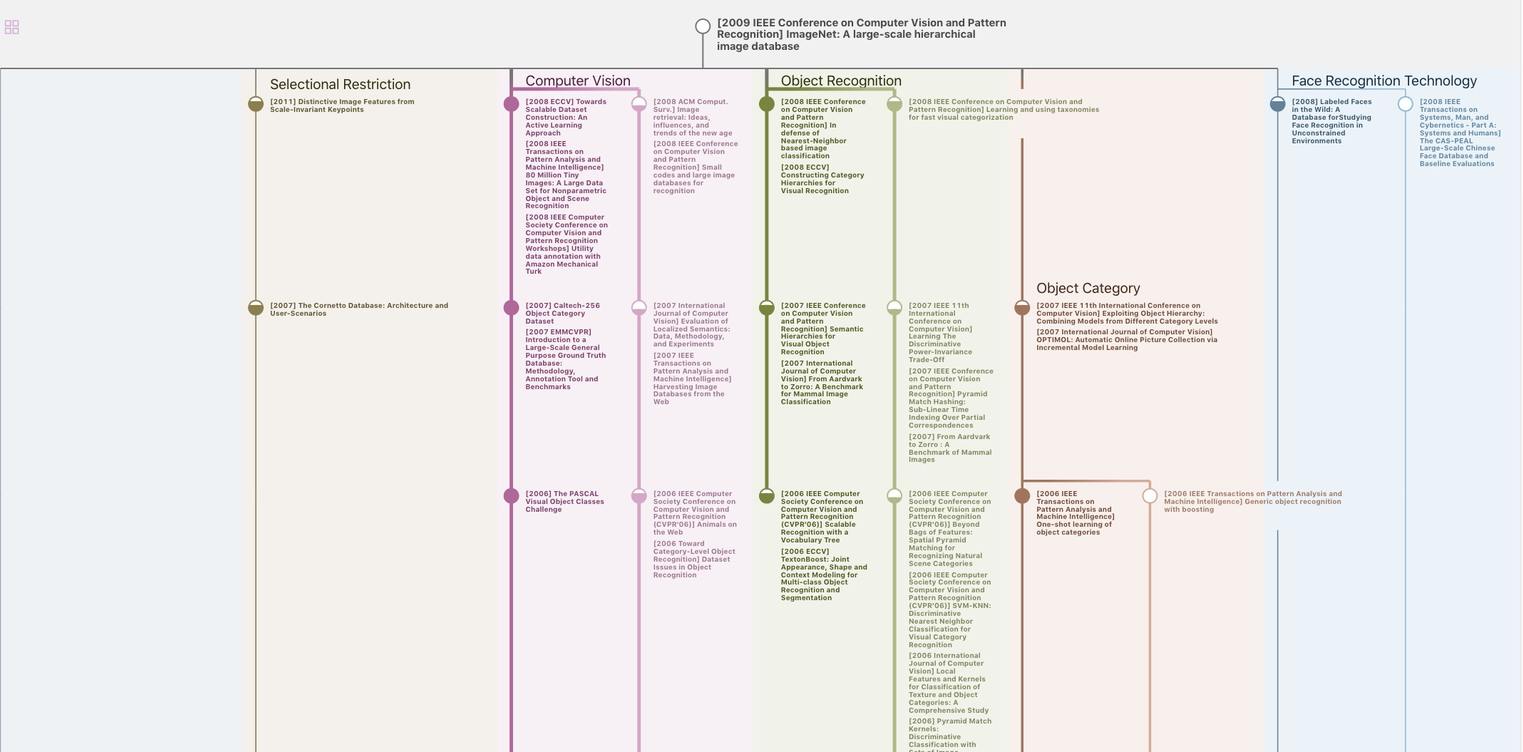
Generate MRT to find the research sequence of this paper
Chat Paper
Summary is being generated by the instructions you defined