Methods for improving colorectal cancer annotation efficiency for artificial intelligence-observer training
WORLD JOURNAL OF RADIOLOGY(2023)
摘要
BACKGROUNDMissing occult cancer lesions accounts for the most diagnostic errors in retrospective radiology reviews as early cancer can be small or subtle, making the lesions difficult to detect. Second-observer is the most effective technique for reducing these events and can be economically implemented with the advent of artificial intelligence (AI). AIMTo achieve appropriate AI model training, a large annotated dataset is necessary to train the AI models. Our goal in this research is to compare two methods for decreasing the annotation time to establish ground truth: Skip-slice annotation and AI-initiated annotation. METHODSWe developed a 2D U-Net as an AI second observer for detecting colorectal cancer (CRC) and an ensemble of 5 differently initiated 2D U-Net for ensemble technique. Each model was trained with 51 cases of annotated CRC computed tomography of the abdomen and pelvis, tested with 7 cases, and validated with 20 cases from The Cancer Imaging Archive cases. The sensitivity, false positives per case, and estimated Dice coefficient were obtained for each method of training. We compared the two methods of annotations and the time reduction associated with the technique. The time differences were tested using Friedman's two-way analysis of variance. RESULTSSparse annotation significantly reduces the time for annotation particularly skipping 2 slices at a time (P < 0.001). Reduction of up to 2/3 of the annotation does not reduce AI model sensitivity or false positives per case. Although initializing human annotation with AI reduces the annotation time, the reduction is minimal, even when using an ensemble AI to decrease false positives. CONCLUSIONOur data support the sparse annotation technique as an efficient technique for reducing the time needed to establish the ground truth.
更多查看译文
关键词
Artificial intelligence,Colorectal cancer,Detection
AI 理解论文
溯源树
样例
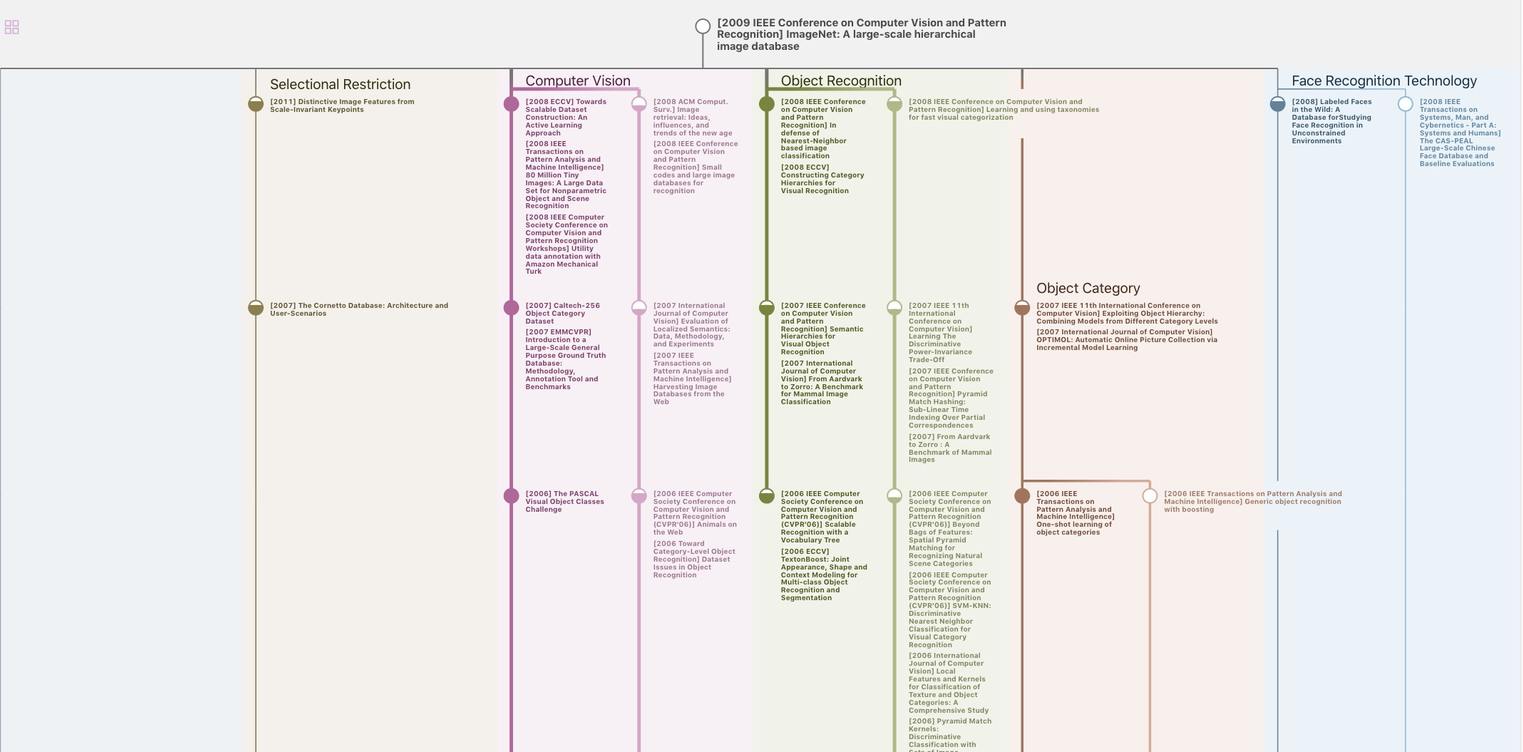
生成溯源树,研究论文发展脉络
Chat Paper
正在生成论文摘要