Federated Learning for distribution skewed data using sample weights
IEEE Transactions on Artificial Intelligence(2024)
摘要
One of the most challenging issues in federated learning is that the data is
often not independent and identically distributed (nonIID). Clients are
expected to contribute the same type of data and drawn from one global
distribution. However, data are often collected in different ways from
different resources. Thus, the data distributions among clients might be
different from the underlying global distribution. This creates a weight
divergence issue and reduces federated learning performance. This work focuses
on improving federated learning performance for skewed data distribution across
clients. The main idea is to adjust the client distribution closer to the
global distribution using sample weights. Thus, the machine learning model
converges faster with higher accuracy. We start from the fundamental concept of
empirical risk minimization and theoretically derive a solution for adjusting
the distribution skewness using sample weights. To determine sample weights, we
implicitly exchange density information by leveraging a neural network-based
density estimation model, MADE. The clients data distribution can then be
adjusted without exposing their raw data. Our experiment results on three
real-world datasets show that the proposed method not only improves federated
learning accuracy but also significantly reduces communication costs compared
to the other experimental methods.
更多查看译文
关键词
feature skewness,communication cost reduction,privacy preservation,deep learning
AI 理解论文
溯源树
样例
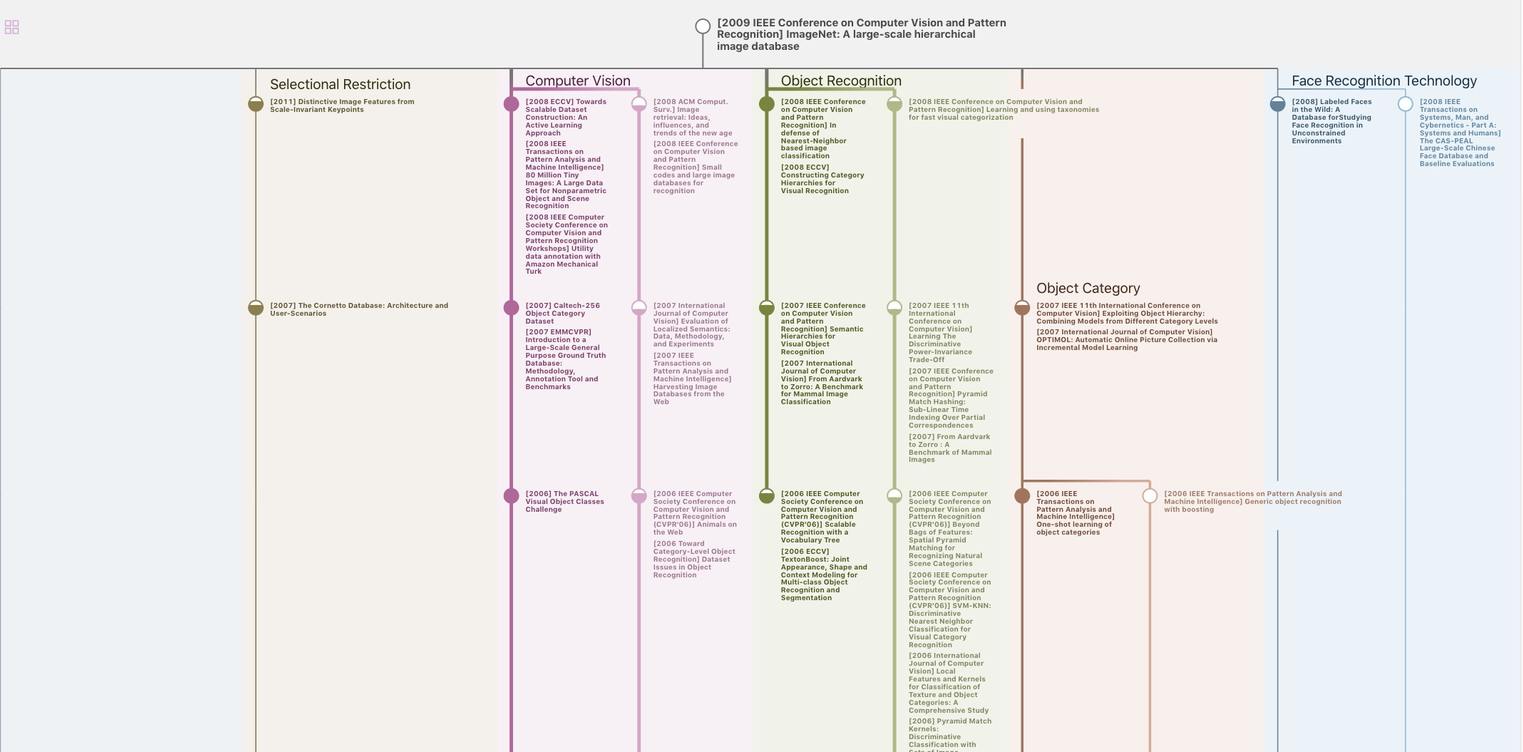
生成溯源树,研究论文发展脉络
Chat Paper
正在生成论文摘要