Using clinical text to refine unspecific condition codes in Dutch general practitioner EHR data
medrxiv(2024)
摘要
Objective: Observational studies using electronic health record (EHR) databases often face challenges due to unspecific clinical codes that can obscure detailed medical information, hindering precise data analysis. In this study, we aimed to assess the feasibility of refining these unspecific condition codes into more specific codes in a Dutch general practitioner (GP) EHR database by leveraging the available clinical free text. Methods: We utilized three approaches for text classification–search queries, semi–supervised learning, and supervised learning–to improve the specificity of ten unspecific International Classification of Primary Care (ICPC–1) codes. Two text representations and three machine learning algorithms were evaluated for the (semi–)supervised models. Additionally, we measured the improvement achieved by the refinement process on all code occurrences in the database. Results: The classification models performed well for most codes. In general, no single classification approach consistently outperformed the others. However, there were variations in the relative performance of the classification approaches within each code and in the use of different text representations and machine learning algorithms. Class imbalance and limited training data affected the performance of the (semi–)supervised models, yet the simple search queries remained particularly effective. Ultimately, the developed models improved the specificity of over half of all the unspecific code occurrences in the database. Conclusions: Our findings show the feasibility of using information from clinical text to improve the specificity of unspecific condition codes in observational healthcare databases, even with a limited range of machine–learning techniques and modest annotated training sets. Future work could investigate transfer learning, integration of structured data, alternative semi–supervised methods, and validation of models across healthcare settings. The improved level of detail enriches the interpretation of medical information and can benefit observational research and patient care.
### Competing Interest Statement
The authors have declared no competing interest.
### Funding Statement
This work has received support from the European Health Data & Evidence Network (EHDEN) project. EHDEN has received funding from the Innovative Medicines Initiative 2 Joint Undertaking (JU) under grant agreement No. 806968. The JU receives support from the European Union's Horizon 2020 research and innovation program and EFPIA.
### Author Declarations
I confirm all relevant ethical guidelines have been followed, and any necessary IRB and/or ethics committee approvals have been obtained.
Yes
The details of the IRB/oversight body that provided approval or exemption for the research described are given below:
The Integrated Primary Care Information (IPCI) database governance board approved this research under code 2022-04.
I confirm that all necessary patient/participant consent has been obtained and the appropriate institutional forms have been archived, and that any patient/participant/sample identifiers included were not known to anyone (e.g., hospital staff, patients or participants themselves) outside the research group so cannot be used to identify individuals.
Yes
I understand that all clinical trials and any other prospective interventional studies must be registered with an ICMJE-approved registry, such as ClinicalTrials.gov. I confirm that any such study reported in the manuscript has been registered and the trial registration ID is provided (note: if posting a prospective study registered retrospectively, please provide a statement in the trial ID field explaining why the study was not registered in advance).
Yes
I have followed all appropriate research reporting guidelines, such as any relevant EQUATOR Network research reporting checklist(s) and other pertinent material, if applicable.
Yes
The aggregated data used for generating the results, conclusions, and figures/tables in this study are available as supplementary data.
更多查看译文
AI 理解论文
溯源树
样例
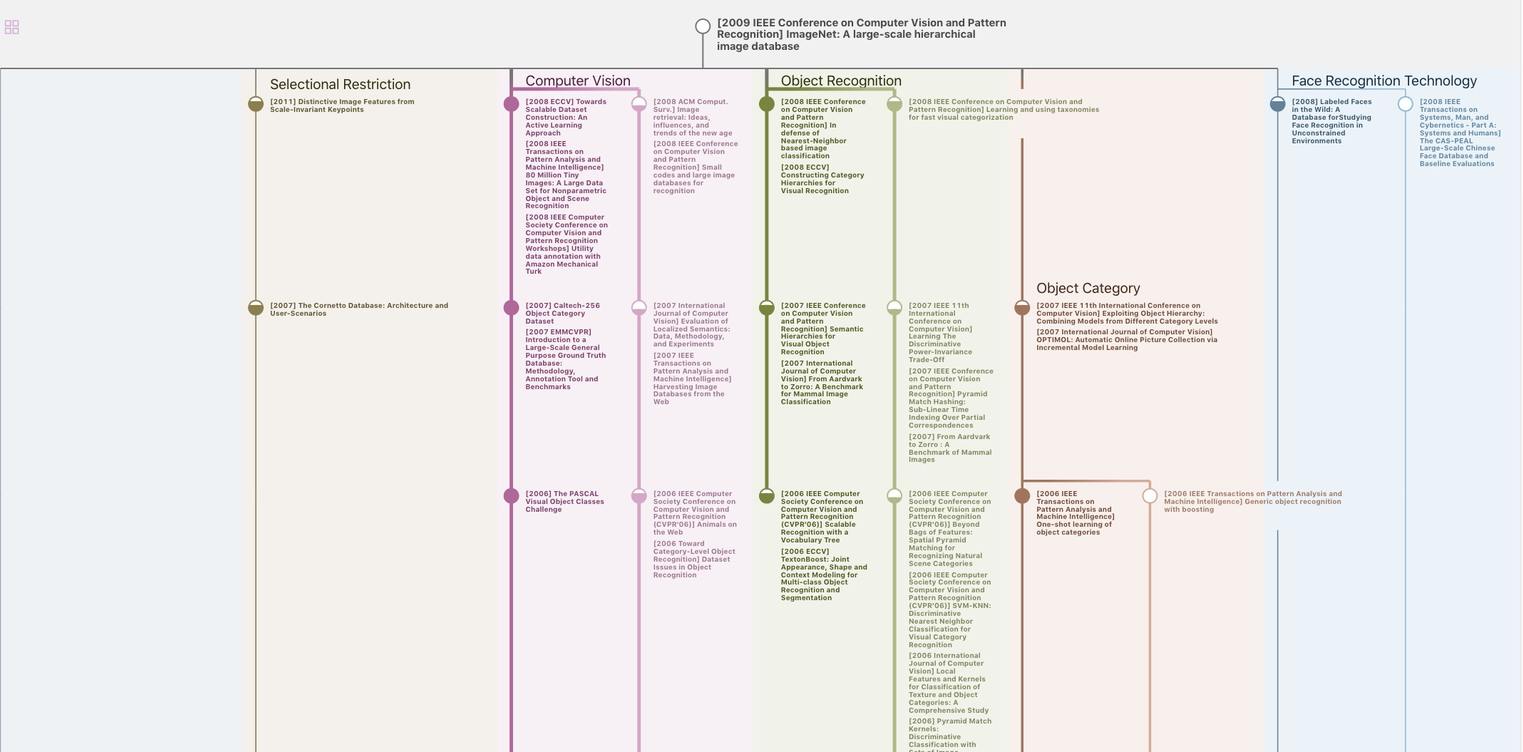
生成溯源树,研究论文发展脉络
Chat Paper
正在生成论文摘要