Distributed Experimental Design Networks
ACM SIGMETRICS Performance Evaluation Review(2024)
摘要
As edge computing capabilities increase, model learning deployments in diverse edge environments have emerged. In experimental design networks, introduced recently, network routing and rate allocation are designed to aid the transfer of data from sensors to heterogeneous learners. We design efficient experimental design network algorithms that are (a) distributed and (b) use multicast transmissions. This setting poses significant challenges as classic decentralization approaches often operate on (strictly) concave objectives under differentiable constraints. In contrast, the problem we study here has a non-convex, continuous DR-submodular objective, while multicast transmissions naturally result in non-differentiable constraints. From a technical standpoint, we propose a distributed Frank-Wolfe and a distributed projected gradient ascent algorithm that, coupled with a relaxation of non-differentiable constraints, yield allocations within a $1-1/e$ factor from the optimal. Numerical evaluations show that our proposed algorithms outperform competitors with respect to model learning quality.
更多查看译文
AI 理解论文
溯源树
样例
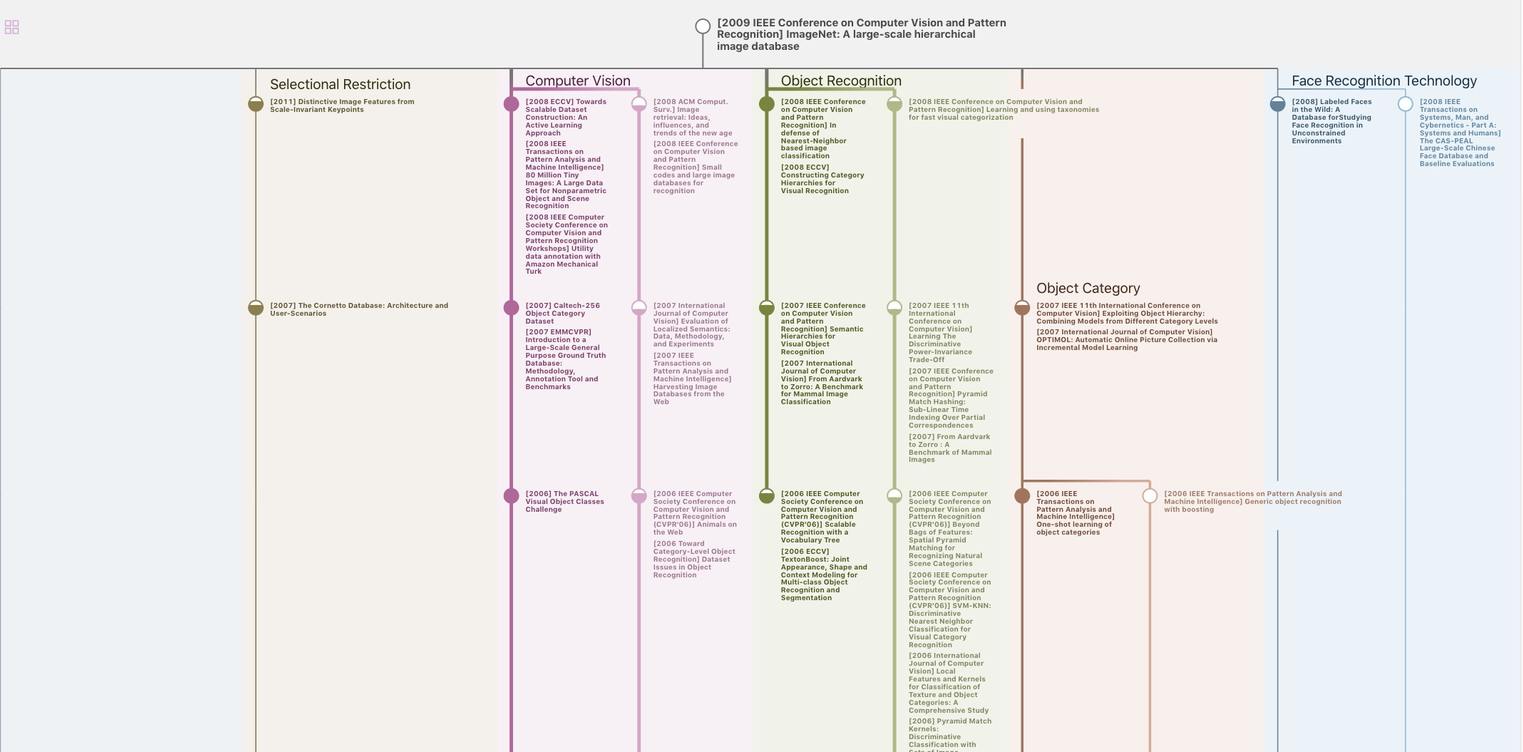
生成溯源树,研究论文发展脉络
Chat Paper
正在生成论文摘要