HQ-VAE: Hierarchical Discrete Representation Learning with Variational Bayes
CoRR(2023)
摘要
Vector quantization (VQ) is a technique to deterministically learn features
with discrete codebook representations. It is commonly performed with a
variational autoencoding model, VQ-VAE, which can be further extended to
hierarchical structures for making high-fidelity reconstructions. However, such
hierarchical extensions of VQ-VAE often suffer from the codebook/layer collapse
issue, where the codebook is not efficiently used to express the data, and
hence degrades reconstruction accuracy. To mitigate this problem, we propose a
novel unified framework to stochastically learn hierarchical discrete
representation on the basis of the variational Bayes framework, called
hierarchically quantized variational autoencoder (HQ-VAE). HQ-VAE naturally
generalizes the hierarchical variants of VQ-VAE, such as VQ-VAE-2 and
residual-quantized VAE (RQ-VAE), and provides them with a Bayesian training
scheme. Our comprehensive experiments on image datasets show that HQ-VAE
enhances codebook usage and improves reconstruction performance. We also
validated HQ-VAE in terms of its applicability to a different modality with an
audio dataset.
更多查看译文
AI 理解论文
溯源树
样例
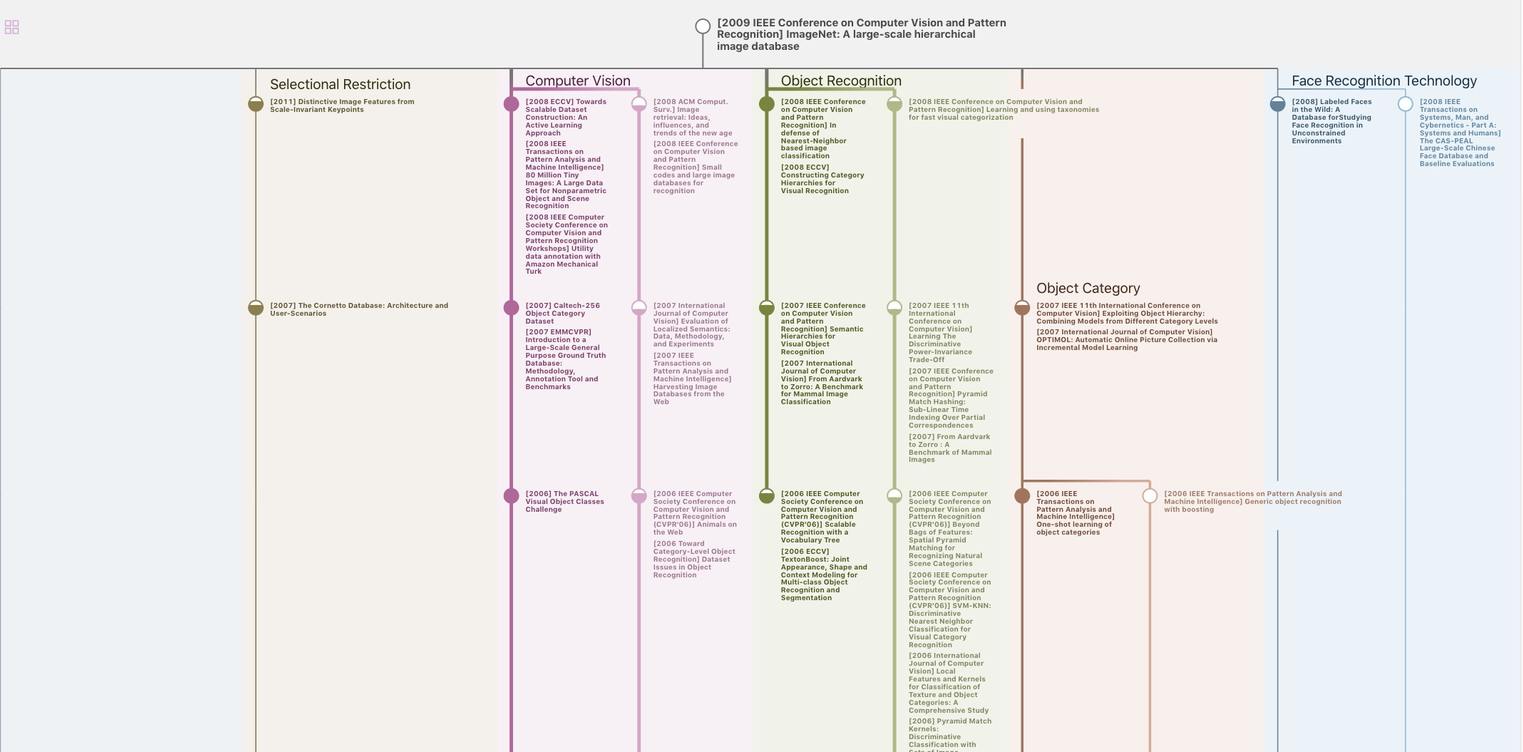
生成溯源树,研究论文发展脉络
Chat Paper
正在生成论文摘要