Attention-aware Social Graph Transformer Networks for Stochastic Trajectory Prediction
CoRR(2023)
摘要
Trajectory prediction is fundamental to various intelligent technologies, such as autonomous driving and robotics. The motion prediction of pedestrians and vehicles helps emergency braking, reduces collisions, and improves traffic safety. Current trajectory prediction research faces problems of complex social interactions, high dynamics and multi-modality. Especially, it still has limitations in long-time prediction. We propose Attention-aware Social Graph Transformer Networks for multi-modal trajectory prediction. We combine Graph Convolutional Networks and Transformer Networks by generating stable resolution pseudo-images from Spatio-temporal graphs through a designed stacking and interception method. Furthermore, we design the attention-aware module to handle social interaction information in scenarios involving mixed pedestrian-vehicle traffic. Thus, we maintain the advantages of the Graph and Transformer, i.e., the ability to aggregate information over an arbitrary number of neighbors and the ability to perform complex time-dependent data processing. We conduct experiments on datasets involving pedestrian, vehicle, and mixed trajectories, respectively. Our results demonstrate that our model minimizes displacement errors across various metrics and significantly reduces the likelihood of collisions. It is worth noting that our model effectively reduces the final displacement error, illustrating the ability of our model to predict for a long time.
更多查看译文
关键词
Motion Trajectory Prediction,Attention-aware,Social Interaction,Spatio-temporal Graph,Transformer
AI 理解论文
溯源树
样例
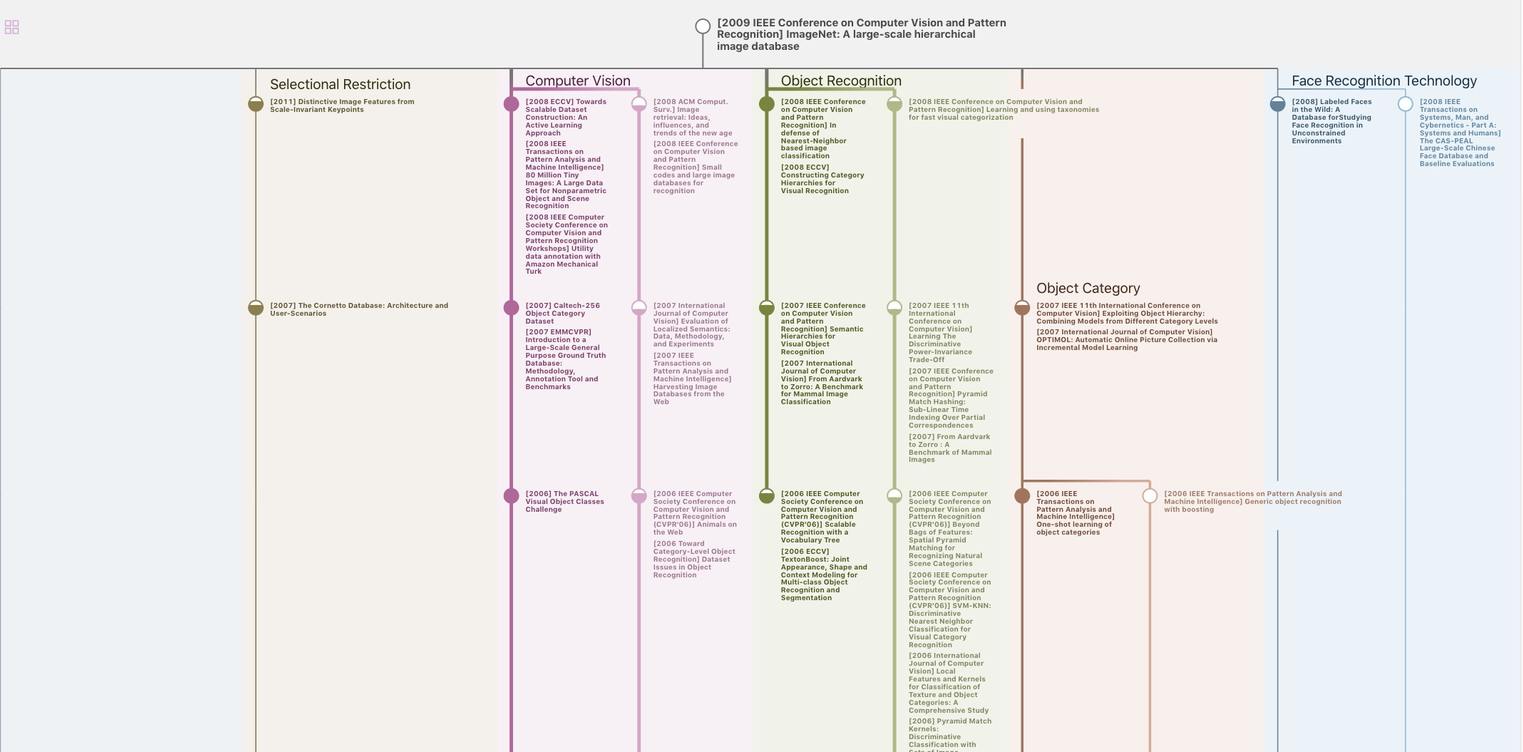
生成溯源树,研究论文发展脉络
Chat Paper
正在生成论文摘要