Multi-target Approaching Control of Hyper-redundant Manipulators using Reinforcement Learning.
2023 IEEE International Conference on Robotics and Biomimetics (ROBIO)(2023)
摘要
Hyper-redundant manipulators have superior dexterity. However, conventional control based on accurate modeling is difficult to achieve. This paper proposes a control method for the hyper-redundant manipulator to complete target approaching tasks using deep reinforcement learning. A hyper-redundant manipulator simulation model was first built and the corresponding forward kinematics equation was analyzed. Then, we used reinforcement learning methods to construct controllers for single target tasks, during which the impact of several key factors on the training was analyzed and optimized accordingly. On this basis, the multi-target task training was achieved by expanding the state to a generalized state. Finally, the proposed control method was verified in a dynamic simulation environment. Simulation results show the effectiveness of the proposed control method.
更多查看译文
AI 理解论文
溯源树
样例
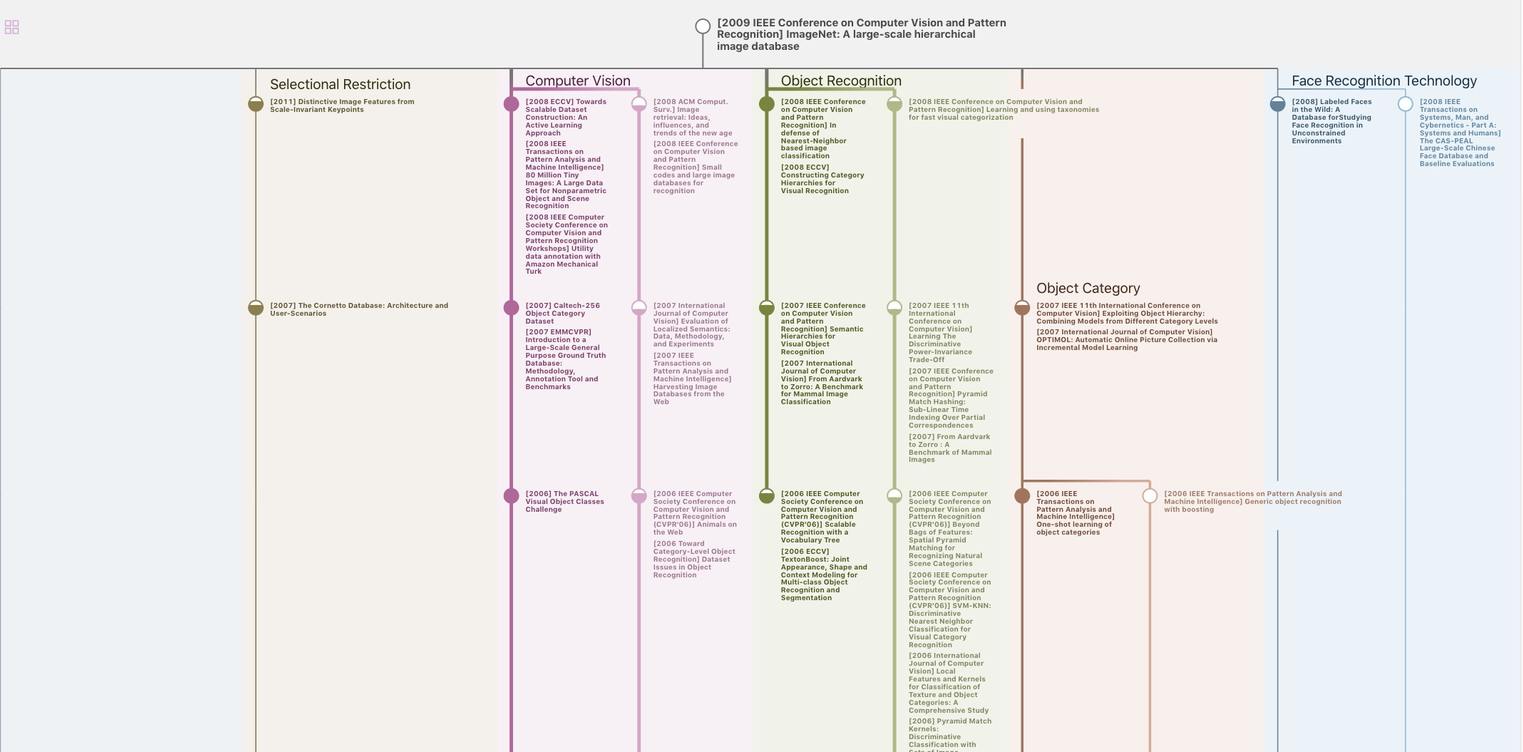
生成溯源树,研究论文发展脉络
Chat Paper
正在生成论文摘要