Recognizing Wafer Map Patterns Using Semi-Supervised Contrastive Learning with Optimized Latent Representation Learning and Data Augmentation.
2023 IEEE International Test Conference (ITC)(2023)
摘要
Wafer map analysis is essential for process issue detection and yield improvement in semiconductor manufacturing. Accurate wafer map pattern recognition facilitates root-causing of abnormal chip fabrication conditions. However, manually annotating wafer map data is expensive and time-consuming, which drives up the demand for exploring label-efficient methods for wafer analysis. This paper proposes a novel contrastive learning framework for wafer map pattern feature extraction and classification. Under the semi-supervised learning setting, the proposed approach aims at learning from a large amount of unlabeled data while efficiently exploiting a small amount of expensive labeled data. Our method utilizes supervised contrastive learning on a small amount of labeled data to learn a better latent space representation with well-separated wafer pattern classes. Furthermore, a dual-encoder latent-space model is incorporated to best optimize the simultaneous use of labeled, unlabeled data, and varying types of data augmentations for representation learning. Finally, we enrich the semantics of the learned latent representation space by introducing a novel interwafer data augmentation to synthesize data which are not present in the given dataset. Experiments show that our method leads existing wafer pattern recognition techniques including recent contrastive learning based approaches by a large performance gain, and suggest that superior accuracy may be achieved simply by semi-supervised learning without resorting to labeling-intensive supervised learning.
更多查看译文
AI 理解论文
溯源树
样例
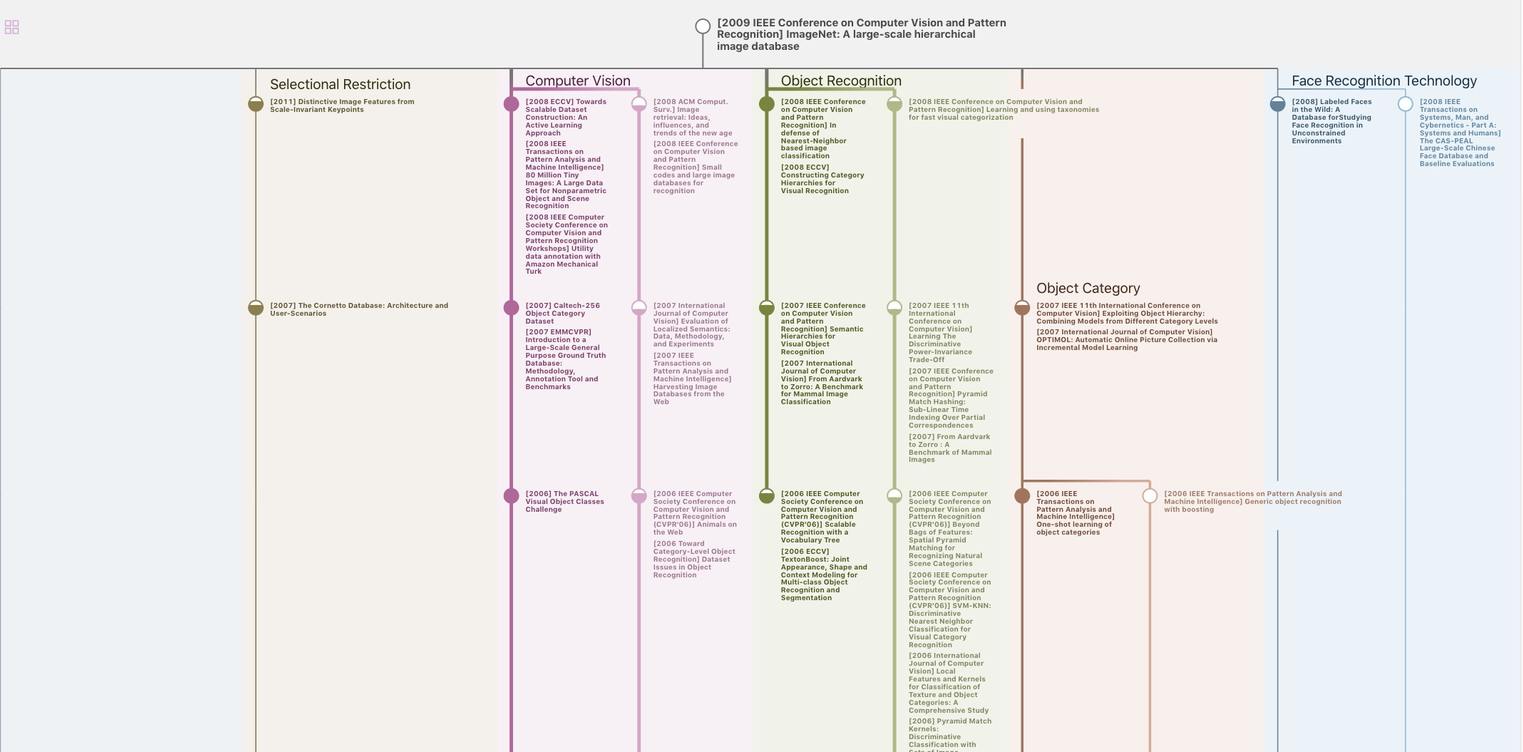
生成溯源树,研究论文发展脉络
Chat Paper
正在生成论文摘要